FSDGNet: frequency and spatial dual guide network for crack detection
JOURNAL OF ELECTRONIC IMAGING(2024)
摘要
Cracks are one of the major threats to the safe operation of civil infrastructures, so timely and accurate detection of cracks is crucial for accident prevention. However, in practical applications, the poor continuity and low contrast of many cracks (e.g., pavement cracks) pose a great challenge to image-based crack detection. In previous approaches, the detection results are often unsatisfactory due to the limitation of the dataset and the singularity of the detection method. To solve this problem, we propose a frequency and spatial dual guide network for crack detection. First, global frequency domain information is processed by the fast Fourier transform to extract low-level features, and then the convolutional neural network uses global frequency domain information to learn local features of the image to extract high-level features. Finally, the feature maps of the two are weighted and fused element by element to obtain the final detection result. Extensive experiments show that our method is superior to any other existing method. (c) 2024 SPIE and IS&T
更多查看译文
关键词
deep learning,convolutional neural network,Fourier transform,crack detection,feature fusion
AI 理解论文
溯源树
样例
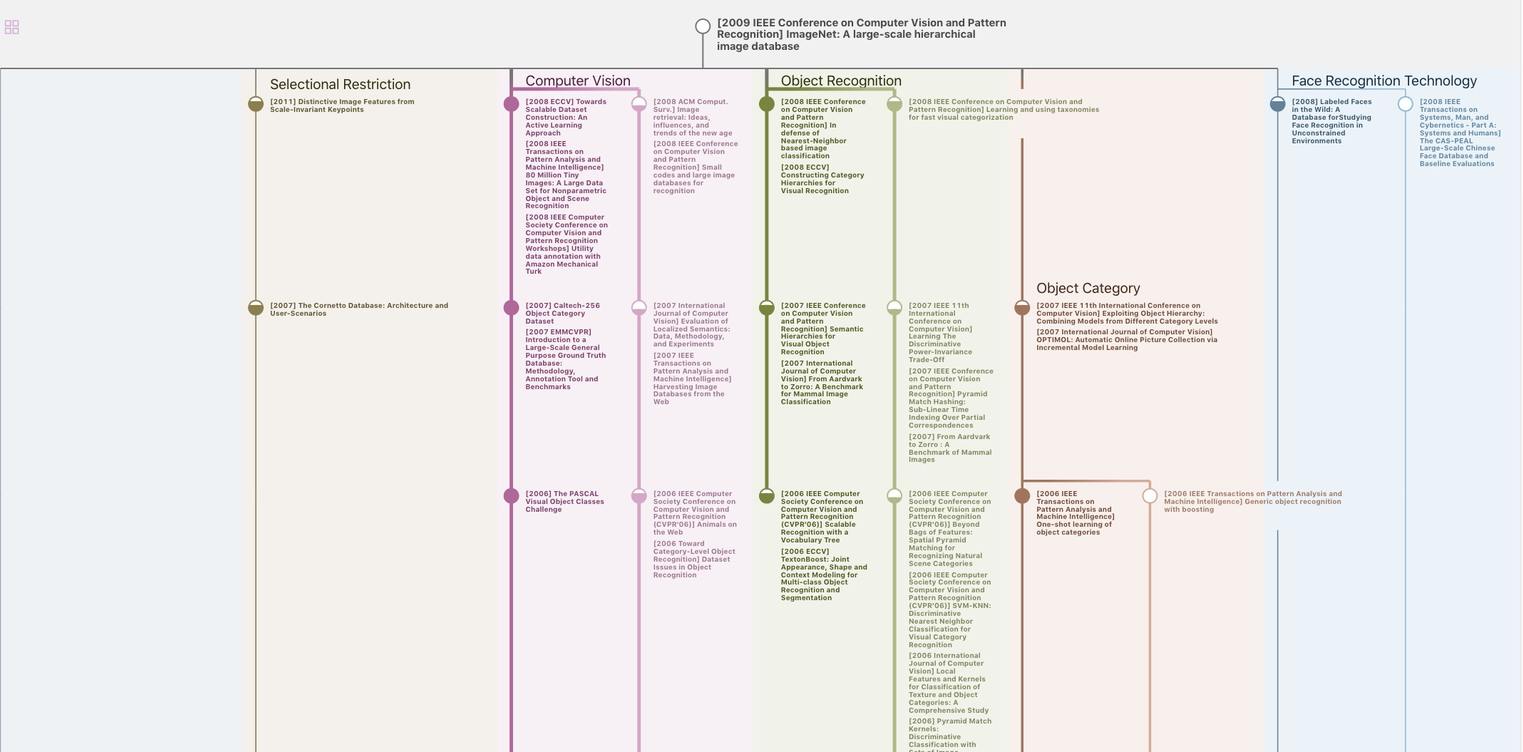
生成溯源树,研究论文发展脉络
Chat Paper
正在生成论文摘要