Credit risk prediction based on causal machine learning: Bayesian network learning, default inference, and interpretation
JOURNAL OF FORECASTING(2024)
摘要
The predictive and interpretable power of models is crucial for financial risk management. The purpose of this study was to perform credit risk prediction in a structured causal network with four stages-data processing, structural learning, parameter learning, and interpretation of inferences-and use six real credit datasets to conduct empirical research on the proposed model. Compared with traditional machine learning algorithms, we comprehensively explain the results of credit default through forward and reverse reasoning. We also compared our model with the post hoc interpretation models local interpretable model-agnostic explanations (LIME) and shapley additive explanations (SHAP) to verify the interpretability of Bayesian networks. The experimental results show that the prediction performance of Bayesian networks is superior to traditional machine learning models and similar to the performance of ensemble models. Furthermore, Bayesian networks offer valuable insights into the interplay of features by considering their causal relationships and enable an assessment of how individual features influence the prediction outcome. In this study, what-if analysis was performed to assess credit default probabilities under various conditions. This analysis provides decision-makers with the necessary tools to make informed judgments about the risk profile of borrowers. Consequently, we consider Bayesian networks as a viable tool for credit risk prediction models in terms of prediction performance and interpretability.
更多查看译文
关键词
Bayesian network,causal inference,credit risk prediction,interpretation
AI 理解论文
溯源树
样例
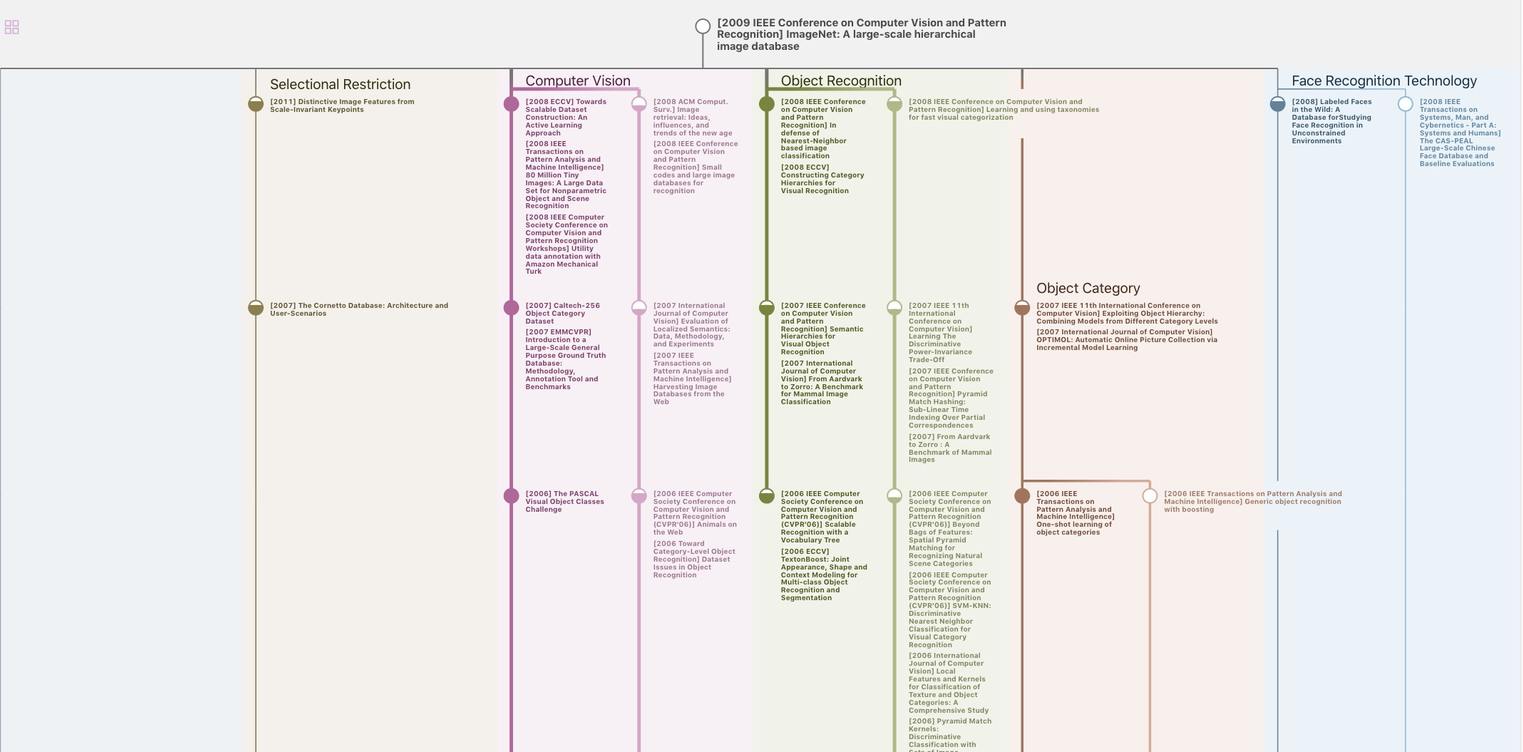
生成溯源树,研究论文发展脉络
Chat Paper
正在生成论文摘要