Quantizing separable convolution of MobileNets with mixed precision
JOURNAL OF ELECTRONIC IMAGING(2024)
摘要
As deep learning moves toward edge computing, researchers have developed techniques for efficient resource usage and accurate inference on mobile devices. Quantization, as one of the key approaches, enables the deployment of deep learning models on embedded platforms. However, MobileNet's accuracy suffers due to quantization errors in depth-wise separable convolutions. To reach a smaller model size, we turn to a mixed-precision quantization strategy instead of uniform quantization. Motivated to gain a higher precision, a quantization-friendly separable convolution architecture has been conducted in a mixed precision quantization strategy search. Our approach introduces a quantization-friendly separable convolution architecture, enhancing MobileNet's accuracy by addressing redundancy and quantization loss. Our framework demonstrates an eight times model size reduction with minimal accuracy loss compared to fixed-bit quantization. Evaluating on the ImageNet dataset and common objects in context dataset, our modified MobileNets almost closed the gap to the floating pipeline across 2-, 4-, 6-, and 8-bit settings. In the ablation experiment, after mixed quantization, our model can still maintain an accuracy of 72.84%, whereas our model has been compressed more than eight times.
更多查看译文
关键词
computer vision,model compression,parameters quantization,edge computing
AI 理解论文
溯源树
样例
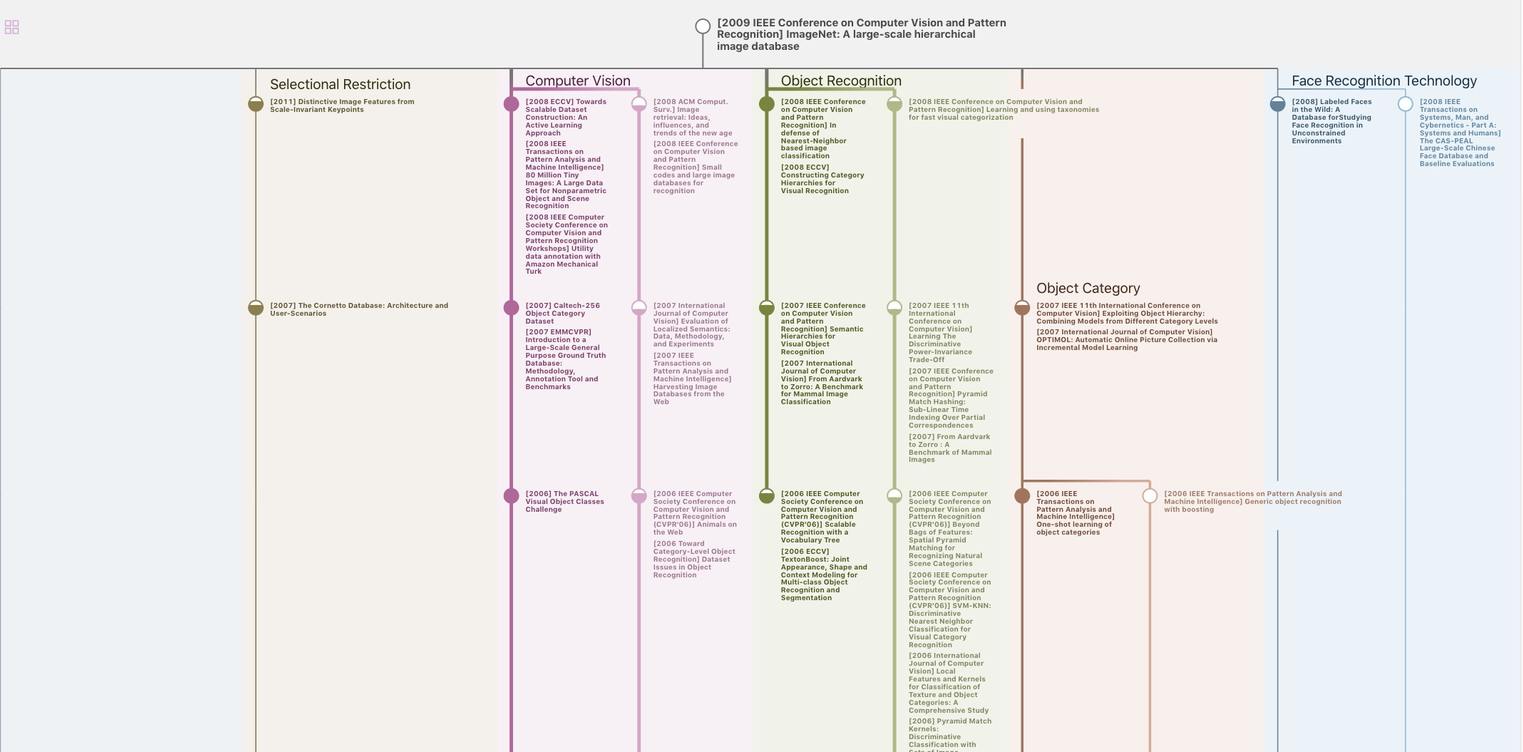
生成溯源树,研究论文发展脉络
Chat Paper
正在生成论文摘要