Characterization of mixing in nanoparticle hetero-aggregates by convolutional neural networks
NANO SELECT(2024)
摘要
Formation of hetero-contacts between particles of different materials in nanoparticle hetero-aggregates can lead to new functional properties. Improvement of the functional behavior requires a detailed characterization of mixing between the two types of particles, in order to correlate different mixing with the performance of the material. Scanning transmission electron microscopy (STEM) is an option for this task. To obtain statistically relevant results, STEM-images of many hetero-aggregates have to be acquired and evaluated. This can be time-consuming if it is done manually. In the present work, the applicability of convolutional neural networks for the automated analysis of STEM-images acquired from TiO2-WO3 nanoparticle hetero-aggregates is investigated. Hetero-aggregates are obtained in a double flame spray pyrolysis (DFSP) setup, in which a variation of setup parameters is expected to affect the mixing of TiO2 and WO3. Mixing is investigated by a measurement of cluster sizes (the number of connected particles of the same material within an aggregate) and coordination numbers (the number of particle contacts with particles of the same or the different material). Results show that the distribution of measured values is wide for both quantities, rendering it challenging to correlate mixing with parameters varied in the DFSP setup.
更多查看译文
关键词
convolutional neural networks,double flame spray pyrolysis,hetero-aggregate,nanoparticle mixing,scanning transmission electron microscopy
AI 理解论文
溯源树
样例
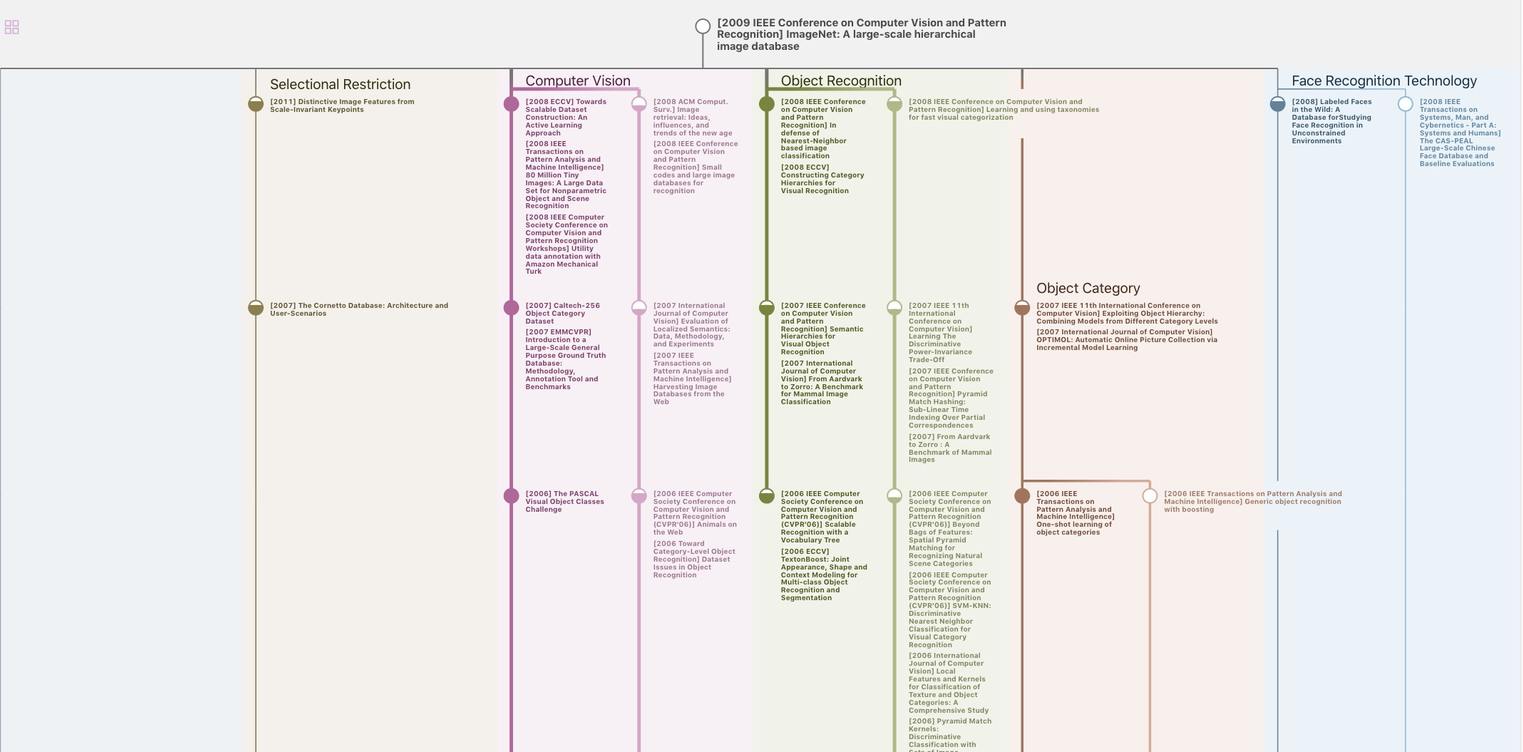
生成溯源树,研究论文发展脉络
Chat Paper
正在生成论文摘要