DiEvD-SF: Disruptive Event Detection Using Continual Machine Learning With Selective Forgetting
IEEE TRANSACTIONS ON COMPUTATIONAL SOCIAL SYSTEMS(2024)
摘要
Detecting disruptive events (DEs), such as riots, protests, and natural calamities, from social media is essential for studying geopolitical dynamics. To automate the process, existing methods rely on classical machine learning (ML) models applied to static datasets, which is counterproductive. To detect DEs from dynamic data streams, this article introduces a novel DiEvD-SF framework, which uses continual machine learning (CML) with selective forgetting. Twitter (currently "X") is used as a real-time and dynamic data source for validation. DiEvD-SF considers the temporal nature of DEs and "selectively forgets" outdated DEs through machine unlearning. To the best of our knowledge, this article is the first to apply CML with selective forgetting to discard outdated DEs and to continue learning about the new DEs. Extensive evaluation using a painstakingly collected Twitter dataset shows that the proposed framework continually identifies new DEs with an average incremental accuracy of 78.942% and successfully forgets old DEs with an average forgetting time of 118.498 seconds, which is better than the state-of-the-art. Additionally, computational analysis is performed to establish the effectiveness of the DiEvD-SF framework by applying various candidate selection strategies.
更多查看译文
关键词
Continual machine learning (CML),disruptive events (DEs) identification,event classification,selective forgetting,Twitter dataset
AI 理解论文
溯源树
样例
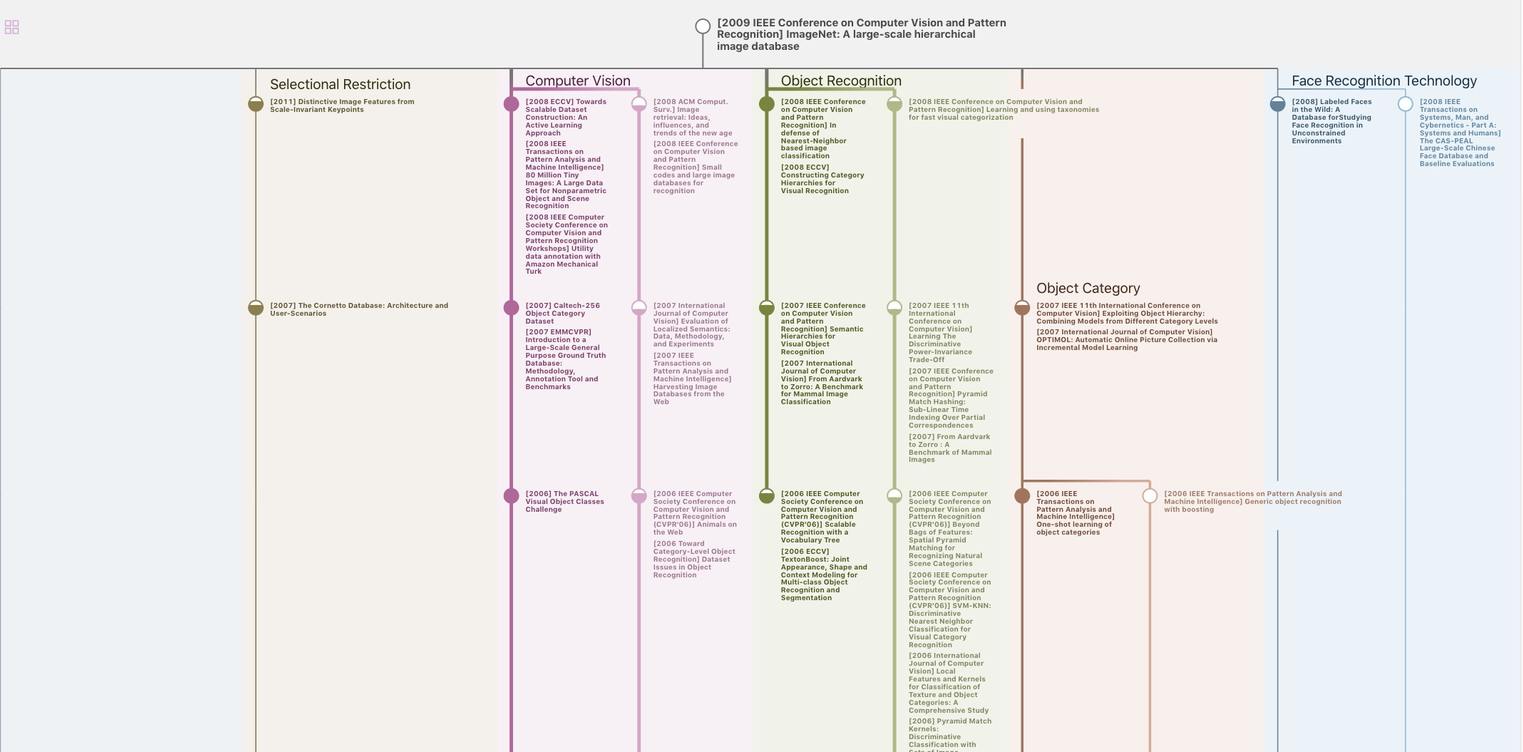
生成溯源树,研究论文发展脉络
Chat Paper
正在生成论文摘要