Ranking-based architecture generation for surrogate-assisted neural architecture search
CONCURRENCY AND COMPUTATION-PRACTICE & EXPERIENCE(2024)
摘要
Architectures generation optimization has been received a lot of attention in neural architecture search (NAS) since its efficiency in generating architecture. By learning the architecture representation through unsupervised learning and constructing a latent space, the prediction process of predictors is simplified, leading to improved efficiency in architecture search. However, searching for architectures with top performance in complex and large NAS search spaces remains challenging. In this paper, an approach that combined a ranker and generative model is proposed to address this challenge through regularizing the latent space and identifying architectures with top rankings. We introduce the ranking error to gradually regulate the training of the generative model, making it easier to identify architecture representations in the latent space. Additionally, a surrogate-assisted evolutionary search method that utilized neural network Bayesian optimization is proposed to efficiently explore promising architectures in the latent space. We demonstrate the benefits of our approach in optimizing architectures with top rankings, and our method outperforms state-of-the-art techniques on various NAS benchmarks. The code is available at .
更多查看译文
关键词
generative model,neural architecture search,ranker,surrogate-assisted evolutionary algorithm
AI 理解论文
溯源树
样例
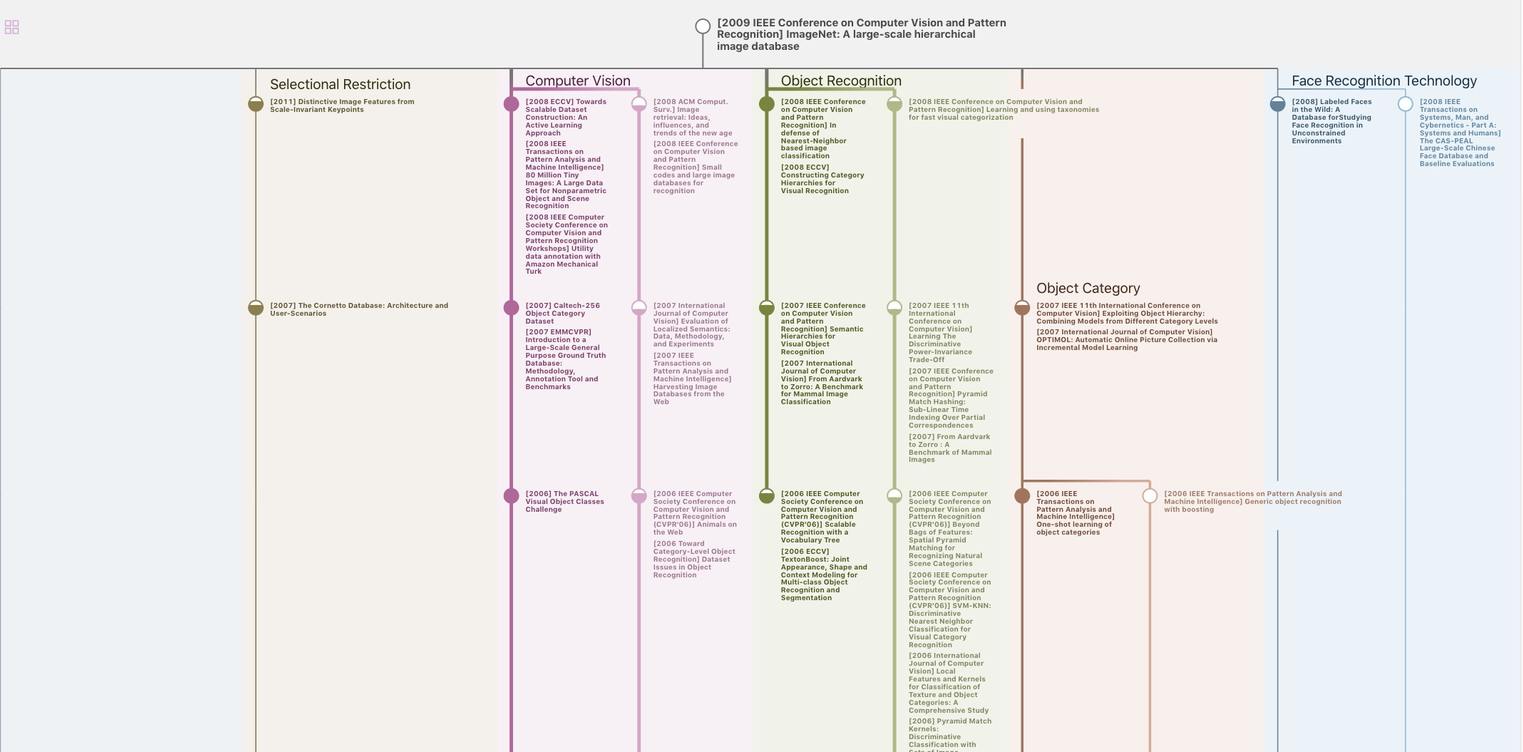
生成溯源树,研究论文发展脉络
Chat Paper
正在生成论文摘要