Collaborative-sequential optimization for aero-engine maintenance based on multi-agent reinforcement learning
EXPERT SYSTEMS WITH APPLICATIONS(2024)
摘要
To ensure the safety of aircraft, traditional intensive aero-engine inspections often lead to inefficient resource allocation. Consequently, there has been a growing research effort on optimization of inspection intervals. This study presents a collaborative-sequential optimization method for aero-engine maintenance, leveraging multiagent reinforcement learning (MARL) techniques. Addressing the challenge of passive agents responding uniformly to rewards, the value decomposition network (VDN) architecture is adopted. Besides, the order requirements between inspection and maintenance determine that there should be timing between multiple agents. Therefore, a sequential simulation environment is constructed to transform multi-agent sequential decisionmaking into a simultaneous process. The proposed method is capable of dynamically adjusting inspection intervals under varying aero-engine degradation conditions. In comparative analysis against baseline strategies and fixed inspection interval strategies, the cost of the proposed method is up to 33.49 % of intensive inspection and 71.6 % of interval optimization, which verifies the superiority of this method.
更多查看译文
关键词
Multi-agent reinforcement learning,Dynamic inspection interval,Maintenance strategy,Aero-engine
AI 理解论文
溯源树
样例
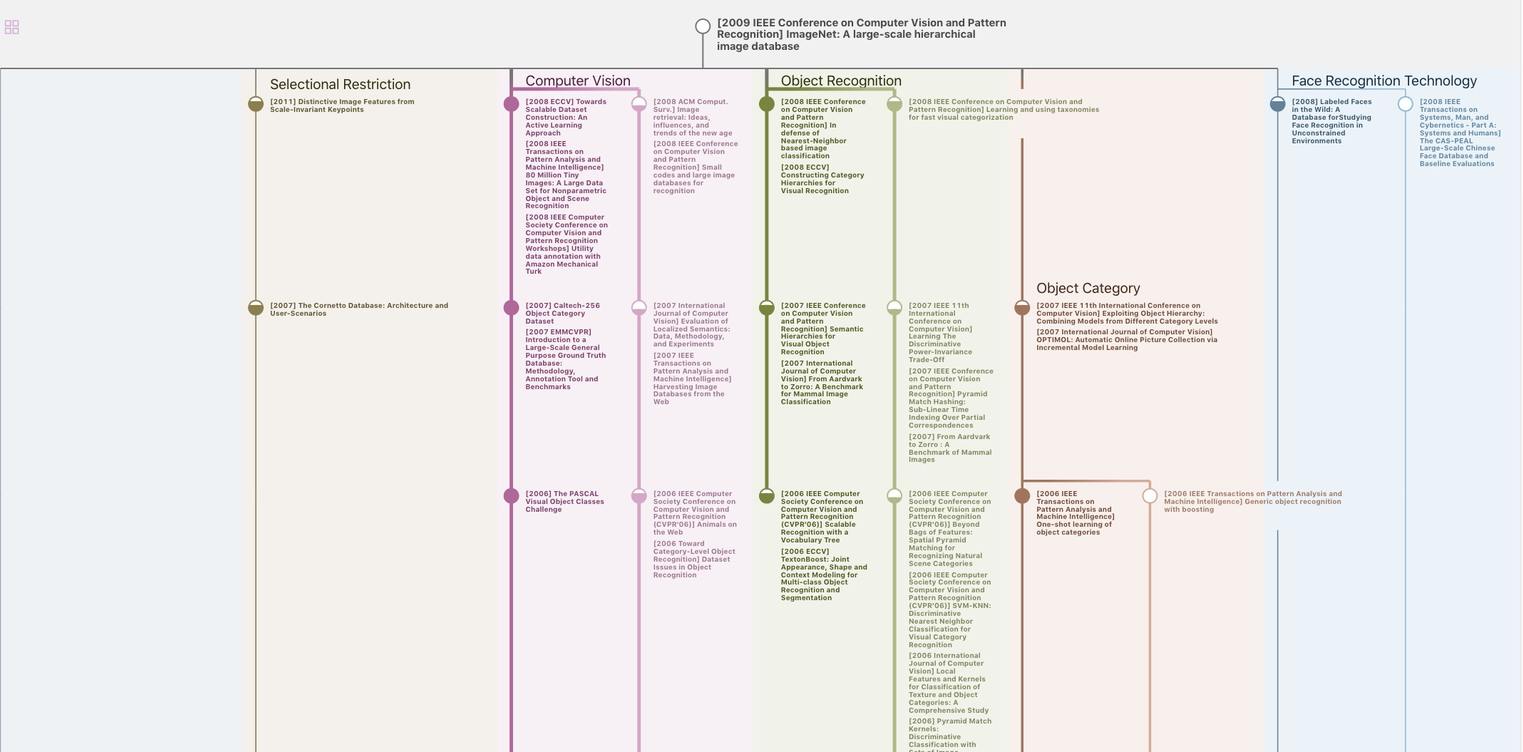
生成溯源树,研究论文发展脉络
Chat Paper
正在生成论文摘要