A Comparison Study of Depth Map Estimation in Indoor Environments Using pix2pix and CycleGAN
IEEE LATIN AMERICA TRANSACTIONS(2024)
摘要
This article presents a Deep Learning-based approach for comparing automatic depth map estimation in indoor environments, with the aim of using them in navigation aid systems for visually impaired individuals. Depth map estimation is a laborious process, as most high-precision systems consist of complex stereo vision systems. The methodology utilizes Generative Adversarial Networks (GANs) techniques for generating depth maps from single RGB images. The study introduces methods for generating depth maps using pix2pix and CycleGAN. The major challenges still lie in the need to use large datasets, which are coupled with long training times. Additionally, a comparison of L1 Loss with a variation of the MonoDepth2 and DenseDepth systems was performed, using ResNet50 and ResNet18 as encoders, which are mentioned in this work, for comparison and validation of the presented method. The results demonstrate that CycleGAN is capable of generating more reliable maps compared to pix2pix and DepthNetResNet50, with an L1 Loss approximately 2,5 times smaller than pix2pix, approximately 2,4 times smaller than DepthNetResNet50, and approximately 14 times smaller than DepthNetResNet18.
更多查看译文
关键词
Estimation,Generative adversarial networks,Navigation,Indoor environment,Image segmentation,Drones,Atmospheric modeling,depth map,navigation system for visually impaired people,generative adversarial networks,pix2pix,CycleGAN,MonoDepth2,DenseDepth,DepthNetResnet50,DepthNetResnet18
AI 理解论文
溯源树
样例
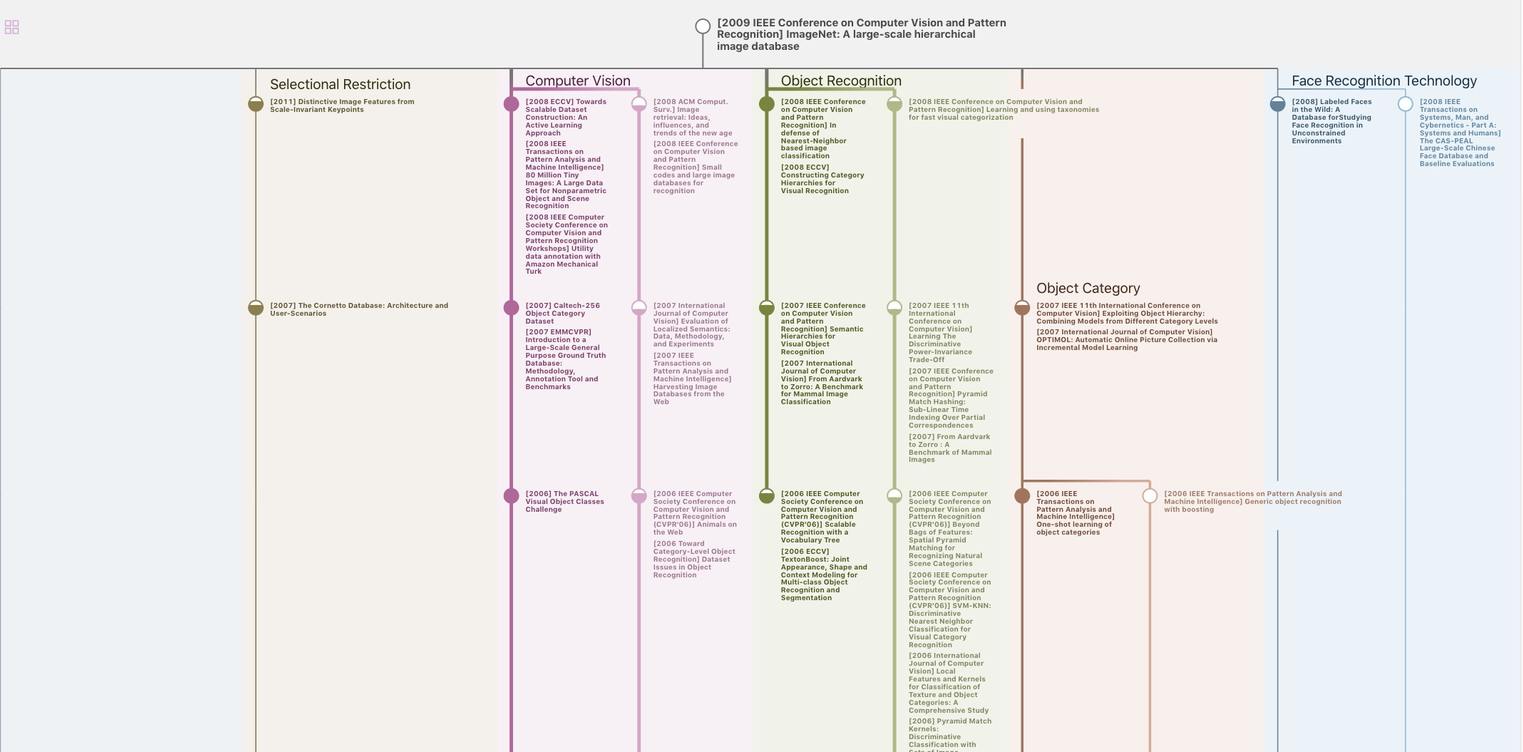
生成溯源树,研究论文发展脉络
Chat Paper
正在生成论文摘要