Real-Time 2D/3D Registration via CNN Regression and Centroid Alignment
IEEE TRANSACTIONS ON AUTOMATION SCIENCE AND ENGINEERING(2024)
摘要
Registration of pre-operative 3D volumes and intra-operative 2D images is critical for neurological interventions. In various 2D/3D registration tasks, deep learning-based approaches have become popular and achieved tremendous success. However, due to vast space of transformation parameters, estimation errors are significant in these approaches. To tackle above issues, a novel learning-based framework for 2D/3D registration is proposed, consisting of CNN regression and centroid alignment. The former introduces a residual regression network (Res-RegNet) to preliminarily estimate transformation parameters. To further reduce estimation errors, the latter utilizes target vessel centroids to refine projected images. The proposed framework is individually trained and evaluated on three patients, reaching mean Dice of 76.69%, 78.51%, and 85.39%, respectively, all outperforming baseline methods. Extensive ablation studies demonstrate centroid alignment can significantly improve registration performance. As a normalization layer in Res-RegNet, SPADE can modulate activations using binarized inputs through a spatially-adaptive, learned transformation. Semantic information of inputs is preserved to learn better representations for parameter estimation. Moreover, the inference rate of our framework is about 21 FPS combined with the state-of-the-art segmentation model, significantly surpassing real-time requirements (6 similar to 12 FPS) in clinical practice. These promising results indicate the potential of the framework to facilitate various 2D/3D registration tasks.
更多查看译文
关键词
Chronic carotid artery occlusion,real-time,2D/3D registration,regression,centroid alignment
AI 理解论文
溯源树
样例
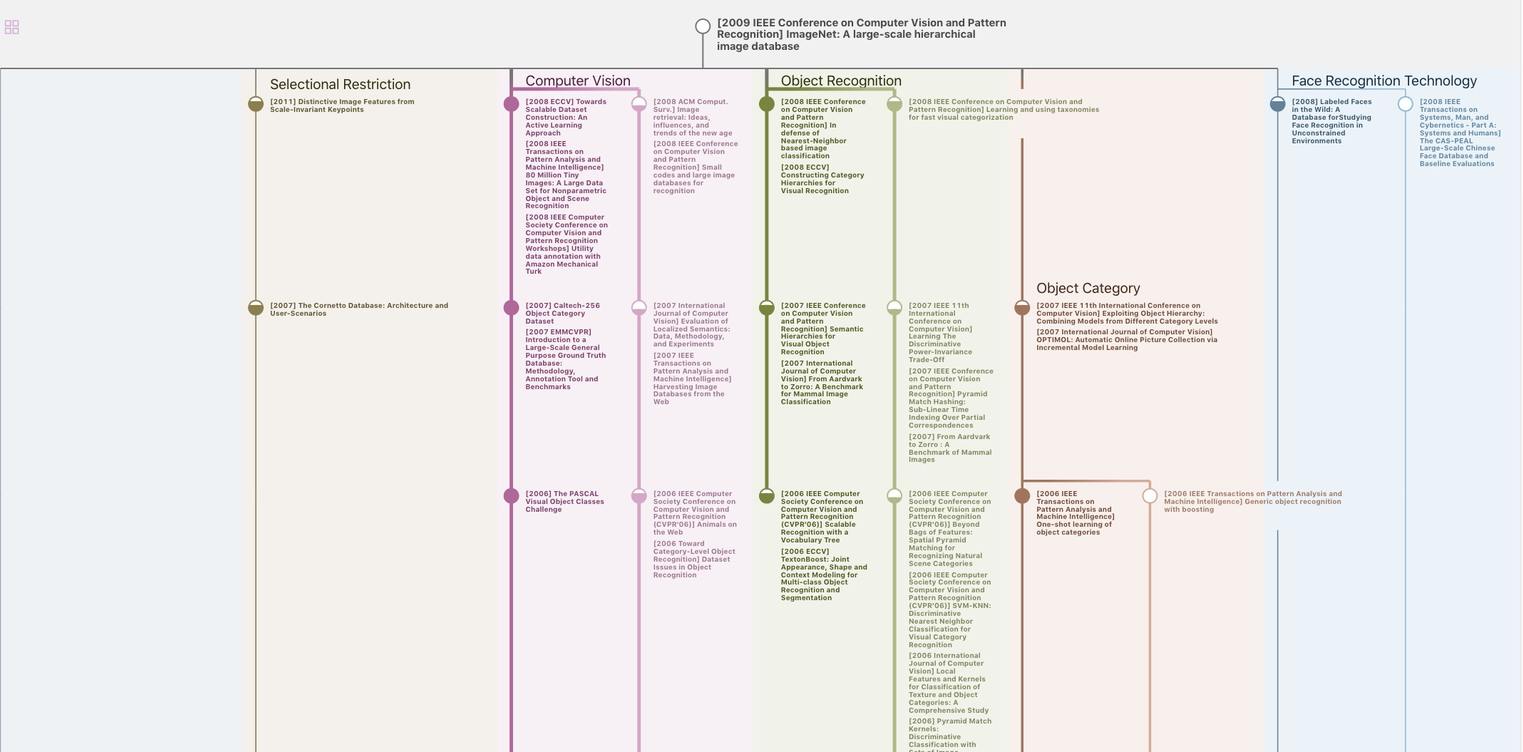
生成溯源树,研究论文发展脉络
Chat Paper
正在生成论文摘要