Hierarchical structural graph neural network with local relation enhancement for hyperspectral image classification
DIGITAL SIGNAL PROCESSING(2024)
摘要
In recent years, graph convolutional networks (GCNs) have made remarkable achievements in the hyperspectral image (HSI) classification task. However, existing GCN-based methods cannot adequately encode similarity edge relationship between superpixels, and few of them use hierarchical mechanism to extract complementary features. This paper addresses these issues and proposes a hierarchical structural graph neural network with local relation enhancement (HSLRE) for HSI classification. Specifically, the features of the pixel -level graph structures are extracted and then embedded into the superpixel-level graph structure to ensure that it does not lose the fine texture features of the original HSI. Secondly, a novel hierarchical framework, which consists of multiple coarsening and refining stages, is proposed to extract multi -level features. In the first coarsening stage, the relational graph convolution (RGC) is introduced to enhance local relations and obtain discriminative features from the superpixel-level graph. In the subsequent coarsening stages, graph convolution (GC) is used to extract features. The refining stages correspond to the coarsening stages, which are used to restore the graphs to their original structures. Finally, to enhance the fluidity of feature information, the fully connected layers and two different types of graph convolutional layers are utilized to extract the linear and nonlinear features of the nodes in parallel, which are fused in a weighted way to form effective features. Experimental results on several benchmark HSI datasets illustrate the effectiveness of the HSLRE.
更多查看译文
关键词
Hyperspectral image classification,Graph convolutional network,Relational graph convolution,Hierarchical framework
AI 理解论文
溯源树
样例
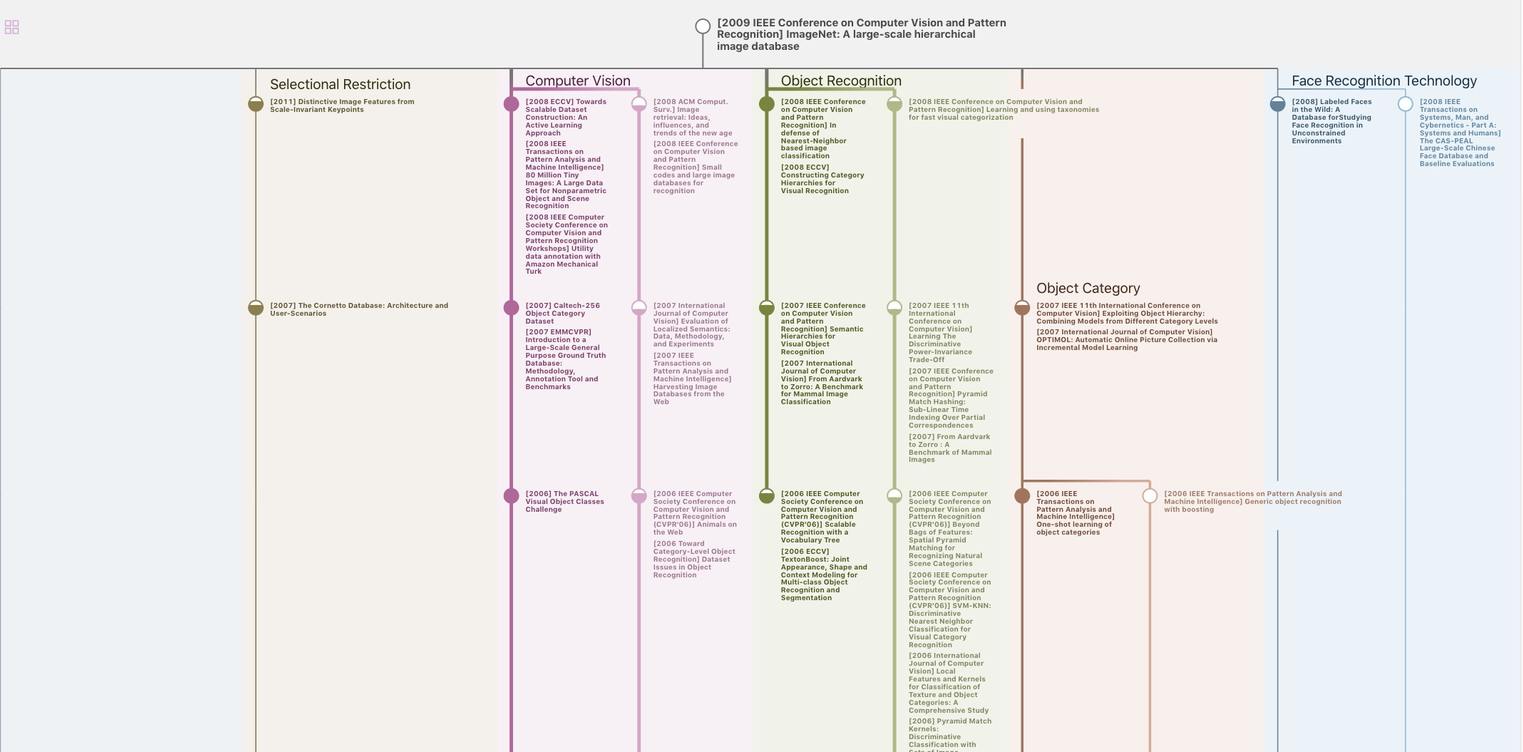
生成溯源树,研究论文发展脉络
Chat Paper
正在生成论文摘要