Optimising post-disaster waste collection by a deep learning-enhanced differential evolution approach
ENGINEERING APPLICATIONS OF ARTIFICIAL INTELLIGENCE(2024)
摘要
In the aftermath of natural disasters, efficient waste collection becomes a crucial challenge, owing to the dynamic and unpredictable nature of waste generation, coupled with resource constraints. This paper presents an innovative hybrid methodology that synergizes Long Short-Term Memory (LSTM) machine learning with Differential Evolution (DE) optimisation to augment waste collection efforts post-disaster. The approach leverages real-time data to forecast waste generation with high accuracy, facilitating the development of adaptable waste collection strategies. Our approach is designed to dynamically update collection plans in response to evolving scenarios, ensuring timely and effective decision-making. Field tests conducted in an earthquake-prone city have demonstrated the superior performance of this method in managing waste collection under fluctuating conditions. Moreover, an in-depth sensitivity analysis helps in identifying key areas for improvement. Significantly outperforming traditional models, this method offers substantial time savings and equips disaster response teams with a robust tool for addressing the challenges of waste collection.
更多查看译文
关键词
Post -disaster waste management,Long short-term memory,Differential evolution,Real-time data analysis,Metaheuristic
AI 理解论文
溯源树
样例
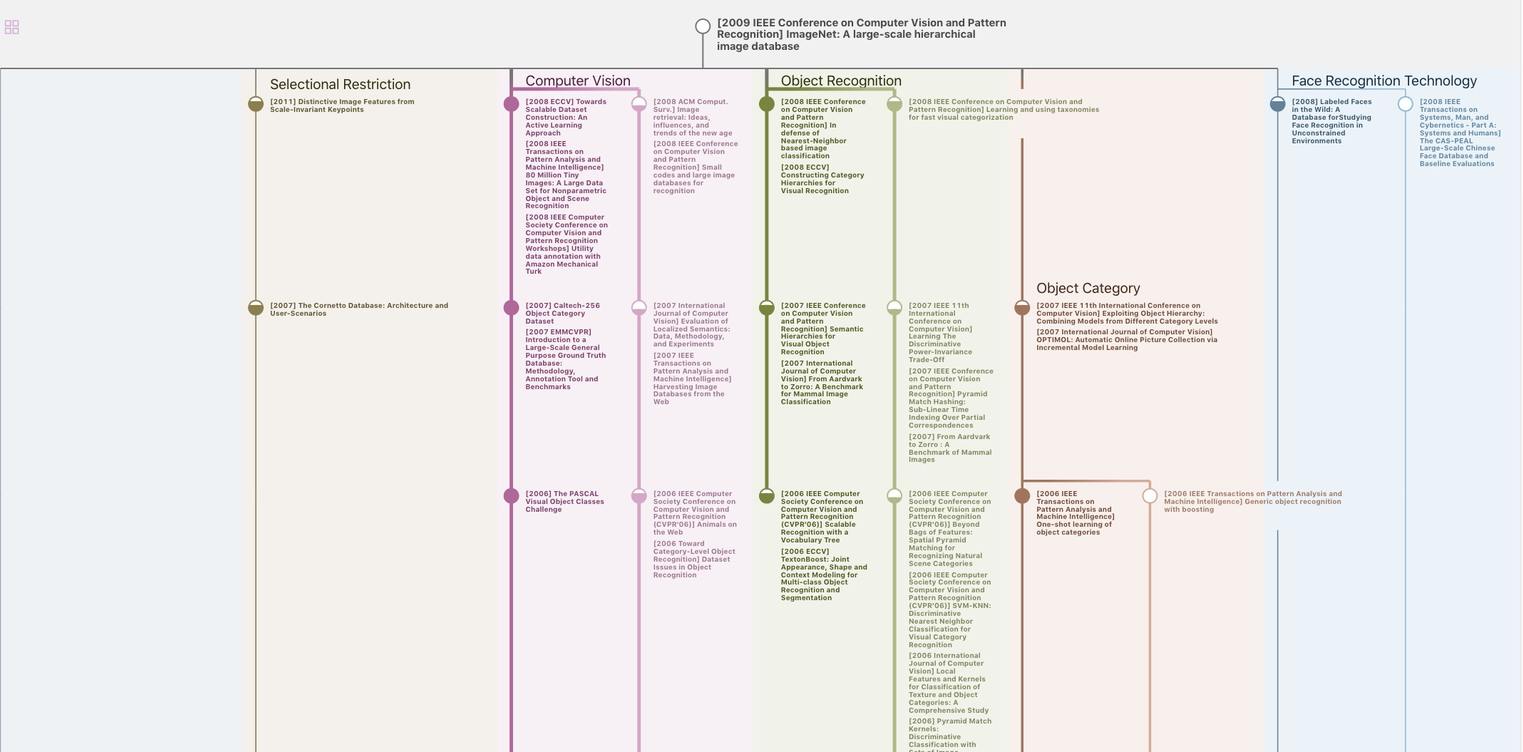
生成溯源树,研究论文发展脉络
Chat Paper
正在生成论文摘要