Instance-level 6D pose estimation based on multi-task parameter sharing for robotic grasping.
Scientific reports(2024)
摘要
Six-dimensional pose estimation task predicts its 3D rotation matrix and 3D translation matrix in the world coordinate system by inputting the color image or depth image of the target object. Existing methods usually use deep neural networks to directly predict or regress object poses based on keypoint methods. The prediction results usually have deviations depending on whether the surface shape of the object is prominent or not and the size of the object. To solve this problem, we propose the six-dimensional pose estimation based on multi-task parameter sharing (PMP) framework to incorporate object category information into the pose estimation network through the form of an object classification auxiliary task. First, we extract the image features and point cloud features of the target object separately, and fuse them point by point; then, we share the confidence of each keypoint in pose estimation task and the knowledge of the classification task, get the key points with higher confidence, and predict the object pose; finally, the obtained object pose is passed through an iterative optimization network to obtain the final pose. The experimental results on the LineMOD dataset show that the proposed method can improve the accuracy of pose estimation and narrow the gap in the prediction accuracy of objects with different shapes. We also tested on a new dataset of small-scale objects, which contains object RGBD images and accurate 3D point cloud information. The proposed method is applied to the grasping experiment on the UR5 robotic arm, which satisfies the real-time pose estimation results during the grasping process.
更多查看译文
AI 理解论文
溯源树
样例
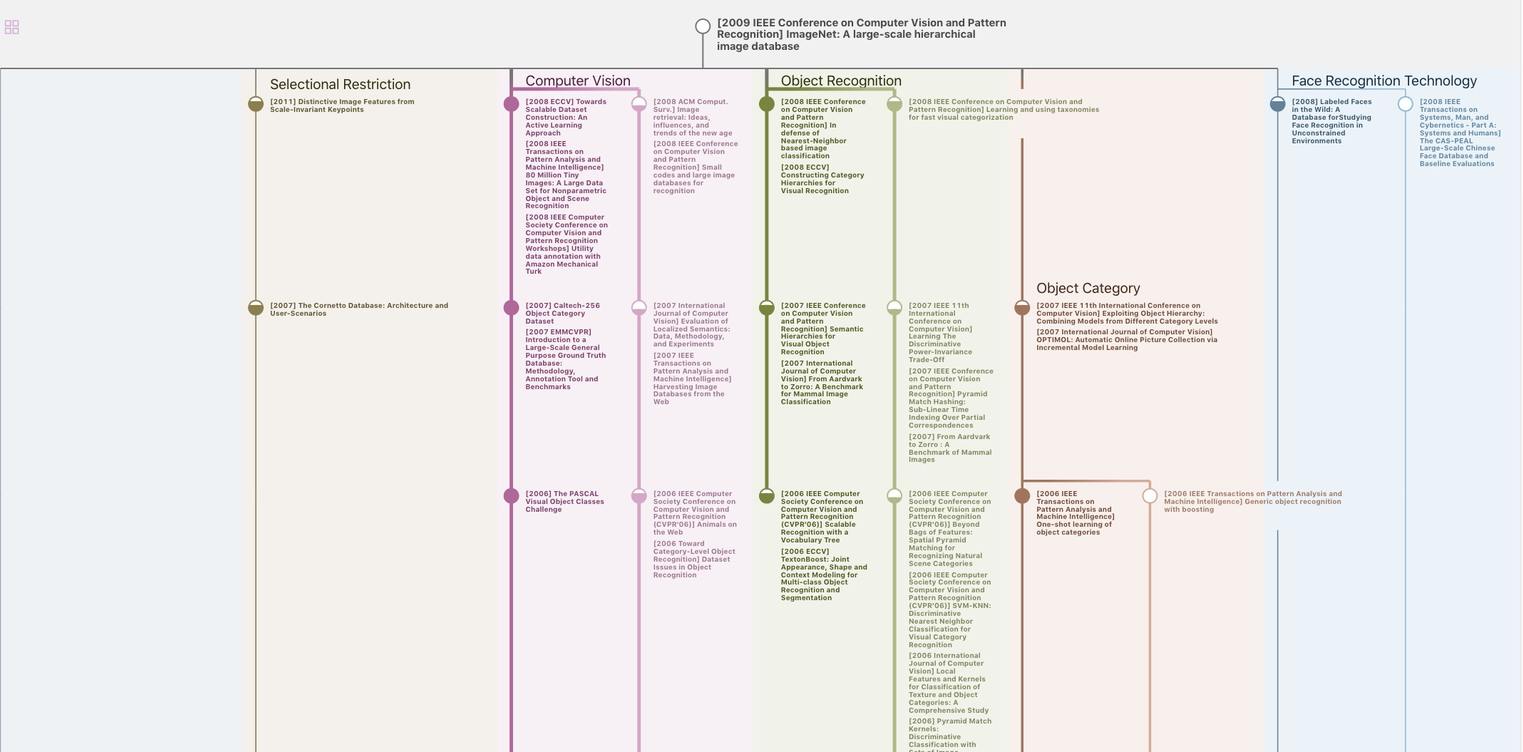
生成溯源树,研究论文发展脉络
Chat Paper
正在生成论文摘要