PainMeter: Automatic Assessment of Pain Intensity Levels From Multiple Physiological Signals Using Machine Learning
IEEE ACCESS(2024)
摘要
Pain assessment traditionally relies on self-report, but it is subjective and influenced by various factors. To address this, there's a need for an affordable and scalable objective pain identification method. Current research suggests that pain has physiological markers beyond the brain, such as changes in cardiovascular activity and electrodermal responses. Utilizing these markers, real-time pain detection algorithms were developed using the BioVid Heat Pain dataset, consisting of 86 healthy individuals experiencing acute pain. Three physiological signals were collected (ECG, GSR, EMG). Various machine learning models were employed to lay the foundation for future advancements in creating sophisticated pain categorization algorithms. The goal is to develop a machine learning model capable of accurately classifying levels of pain experienced based solely on physiological signals. The proposed method produced an accuracy score of 87% for binary classification and 52% accuracy for multi-class classification, with the highest-performing machine learning model being Random Forests. These results suggest that the PainMeter can be deployed in field settings using wearable sensors, offering real-time, unbiased pain sensing and management capabilities.
更多查看译文
关键词
ECG,EMG,GSR,machine learning,pain intensity,physiology,signal,classification
AI 理解论文
溯源树
样例
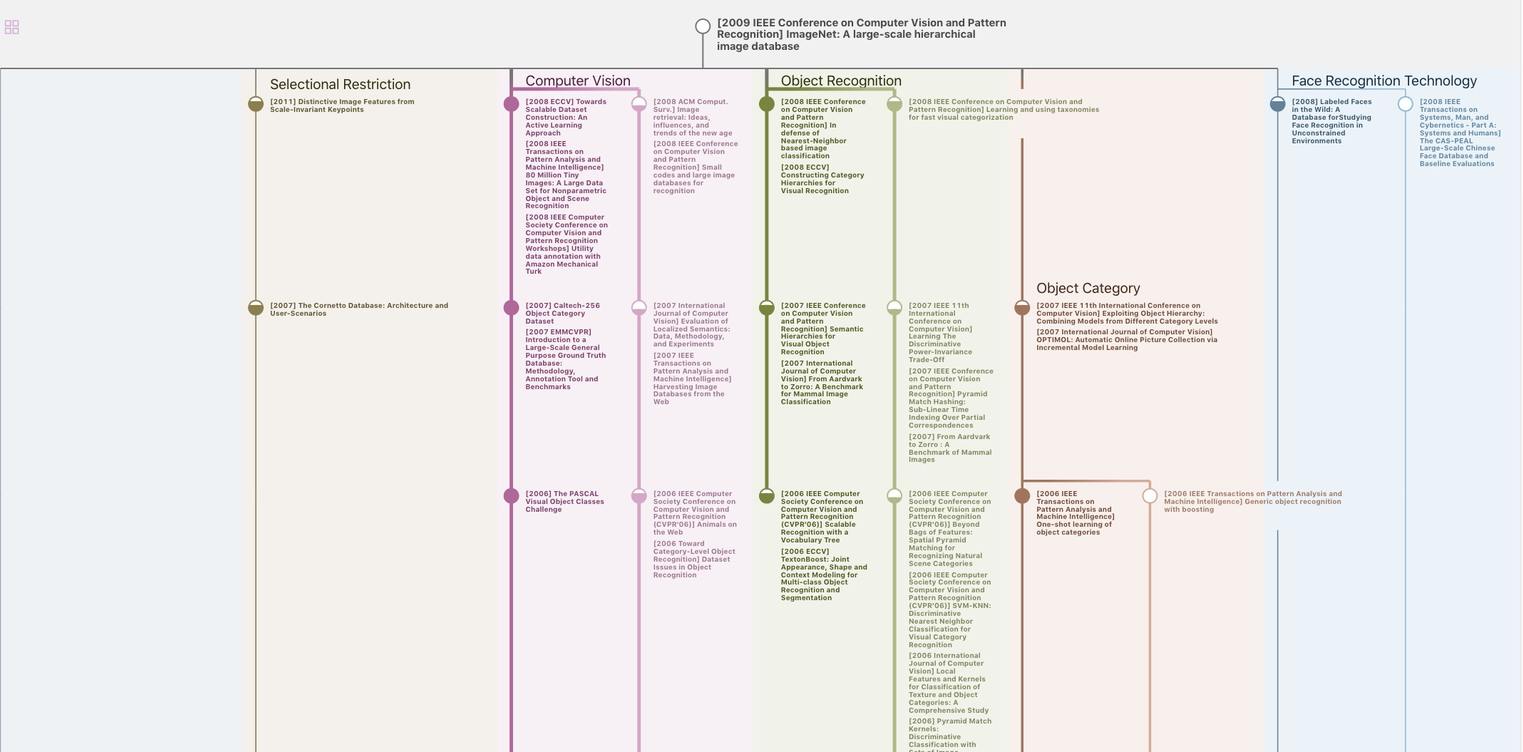
生成溯源树,研究论文发展脉络
Chat Paper
正在生成论文摘要