SpiCS-Net: Circuit Switched Network on Chip for Area-Efficient Spiking Recurrent Neural Networks.
International Conference on VLSI Design(2024)
摘要
Spiking Recurrent Neural Networks (SRNNs) present an alternative computing paradigm to traditional von Neumann computing in the post-Moore’s law era because they omit the memory-processor bottleneck and enable low-power resource-efficient computations. When utilized as a reservoir in a Reservoir Computing (RC) system, SRNNs enable applications in temporal signal processing involving complex data patterns, e.g., chaotic time-series prediction and control in non-linear dynamical systems. Implementing the RC system based on SRNNs on hardware would enable low-power and area-efficient computing, suitable for various applications in resource-constrained systems like battery-powered edge devices. However, one of the significant challenges in achieving this on hardware relates to the Network-on-Chip (NoC) implementation for the SRNN. This NoC implementation challenge stems from the requirement to support reconfigurable connectivity across the chip to enable connections between any arbitrary pair of neurons within the network. Traditional NoC approaches are packet-based spike routing networks that require extra packet-handling circuits and are targeted for large-scale SRNNs. In this work, we propose SpiCS-Net, pronounced spikes-net, a Spike-routing Clos Circuit-Switched Network-on-Chip architecture. SpiCS-Net is a switch-based communication scheme that avoids the conversion of spikes into data packets. Thus, SpiCS-Net is highly efficient when compared to packet-based networks. SpiCS-Net is evaluated and compared with state-of-the-art for several key network design metrics, including throughput, area, and power consumption. Compared to packet-based approaches in the literature, SpiCS-Net offers up to 9% higher throughput and nearly $13\times$ savings in area.
更多查看译文
关键词
Neuromorphic,Hardware,Network-on-Chip,NoC,SpiCS-Net,Circuit Switching,AER,SRNN,Neural Networks
AI 理解论文
溯源树
样例
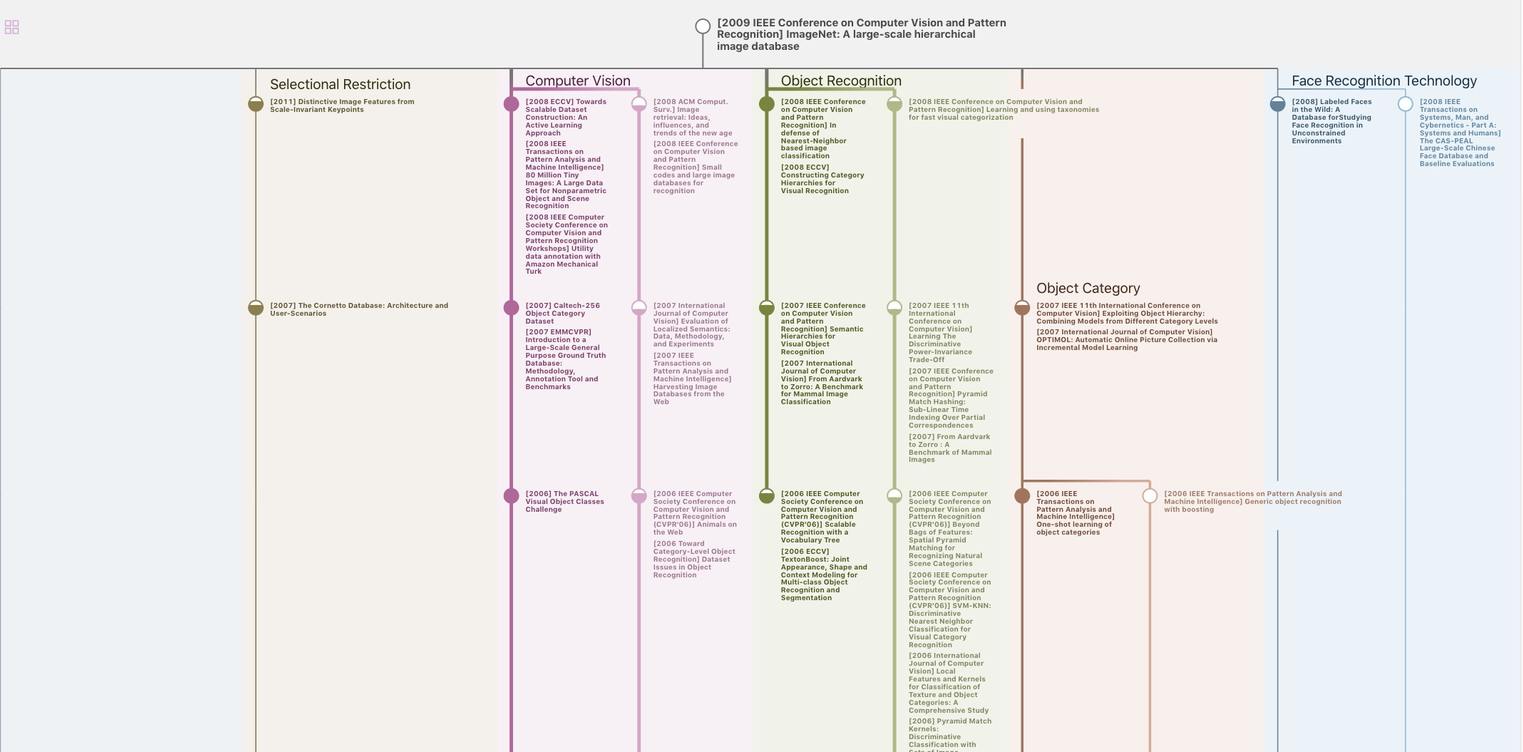
生成溯源树,研究论文发展脉络
Chat Paper
正在生成论文摘要