Deep Learning Techniques for Automatic Text Summarization: A Review
2024 IEEE International Students' Conference on Electrical, Electronics and Computer Science (SCEECS)(2024)
摘要
The volume of text data has grown drastically since the internet’s inception, which has had a profound impact on how data is gathered, analyzed, and understood. To address this issues text summarization has come into the picture which is a critical natural language processing (NLP) task aimed at condensing lengthy documents or articles into concise and coherent representations, preserving the essential information while eliminating redundancy and irrelevant details. This study examines the most recent advancements in extractive and abstractive text summarizing approaches. In extractive summarization, the most significant sections and paragraphs from the source material are identified and selected based on a score determined by the overall amount of words in that paragraph. Abstractive summarization, it generates condensed summaries by interpreting and rephrasing text, often using natural language generation techniques. Additionally, we explore the challenges and evaluation metrics, datasets associated with automatic summarization, emphasizing the need for more robust evaluation protocols that align with human judgments. In sum, this paper serves as a comprehensive guide to text summarization, offering insights into its evolution, current landscape, challenges, and potential for transformative impact across various domains.
更多查看译文
关键词
Text summarization,Natural language processing (NLP),Extractive summarization,Abstractive summarization,Evaluation metrics,Robust evaluation protocols,Human judgments
AI 理解论文
溯源树
样例
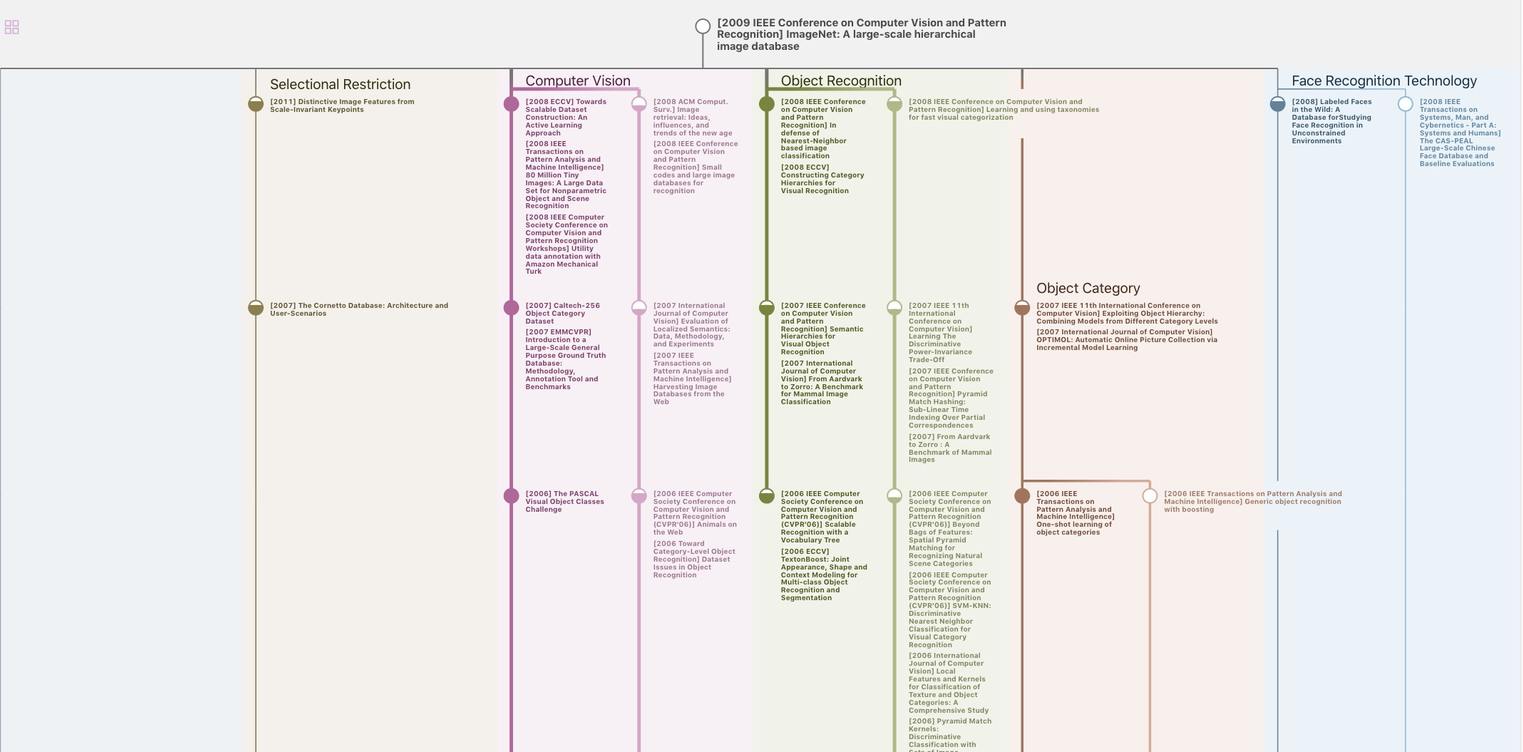
生成溯源树,研究论文发展脉络
Chat Paper
正在生成论文摘要