Iterated Learning Improves Compositionality in Large Vision-Language Models
arxiv(2024)
摘要
A fundamental characteristic common to both human vision and natural language
is their compositional nature. Yet, despite the performance gains contributed
by large vision and language pretraining, recent investigations find that
most-if not all-our state-of-the-art vision-language models struggle at
compositionality. They are unable to distinguish between images of " a girl in
white facing a man in black" and "a girl in black facing a man in white".
Moreover, prior work suggests that compositionality doesn't arise with scale:
larger model sizes or training data don't help. This paper develops a new
iterated training algorithm that incentivizes compositionality. We draw on
decades of cognitive science research that identifies cultural transmission-the
need to teach a new generation-as a necessary inductive prior that incentivizes
humans to develop compositional languages. Specifically, we reframe
vision-language contrastive learning as the Lewis Signaling Game between a
vision agent and a language agent, and operationalize cultural transmission by
iteratively resetting one of the agent's weights during training. After every
iteration, this training paradigm induces representations that become "easier
to learn", a property of compositional languages: e.g. our model trained on
CC3M and CC12M improves standard CLIP by 4.7
SugarCrepe benchmark.
更多查看译文
AI 理解论文
溯源树
样例
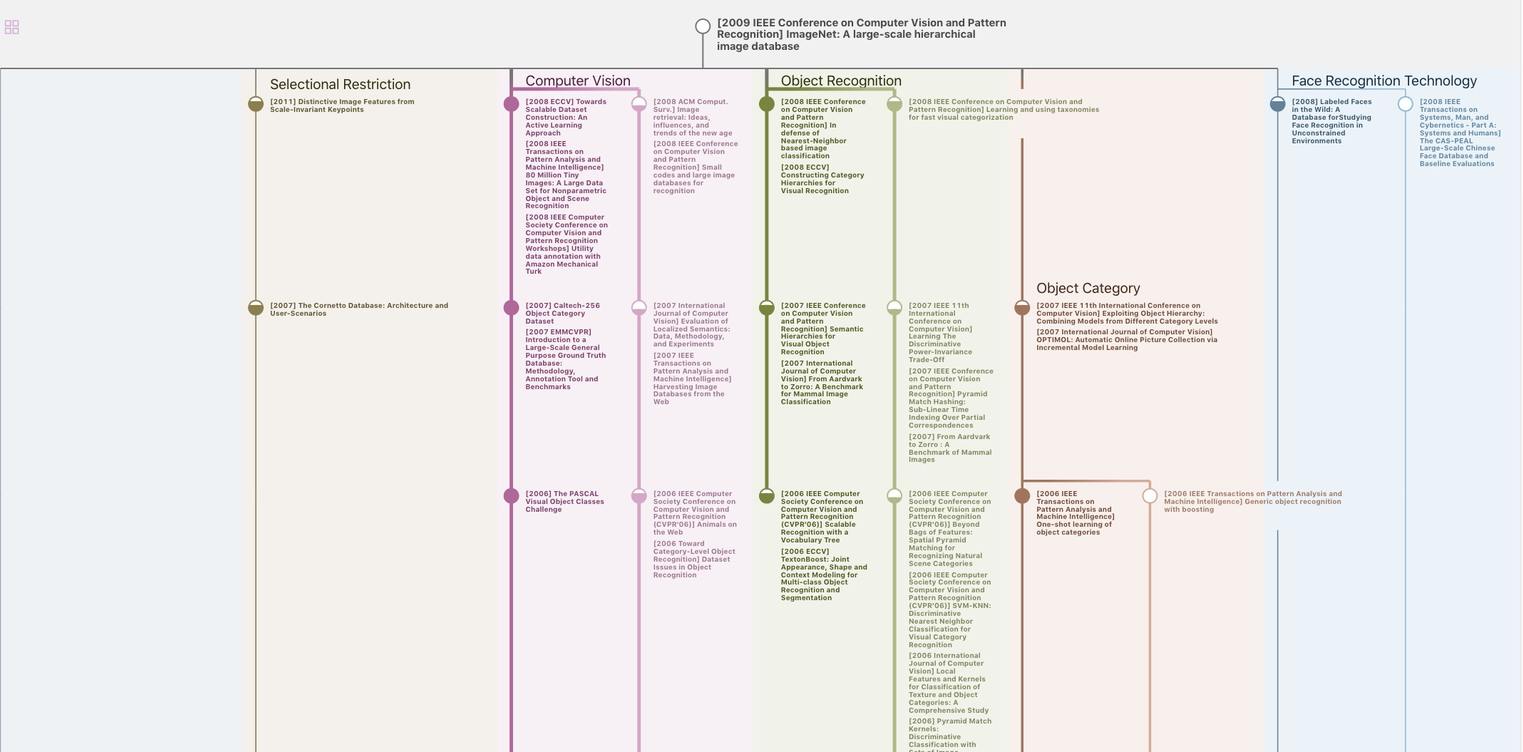
生成溯源树,研究论文发展脉络
Chat Paper
正在生成论文摘要