Ridge regularization for spatial autoregressive models with multicollinearity issues
AStA Advances in Statistical Analysis(2024)
摘要
This work proposes a new method for building an explanatory spatial autoregressive model in a multicollinearity context. We use Ridge regularization to bypass the collinearity issue. We present new estimation algorithms that allow for the estimation of the regression coefficients as well as the spatial dependence parameter. A spatial cross-validation procedure is used to tune the regularization parameter. In fact, ordinary cross-validation techniques are not applicable to spatially dependent observations. Variable importance is assessed by permutation tests since classical tests are not valid after Ridge regularization. We assess the performance of our methodology through numerical experiments conducted on simulated synthetic data. Finally, we apply our method to a real data set and evaluate the impact of some socioeconomic variables on the COVID-19 intensity in France.
更多查看译文
关键词
Spatial autoregressive models,Multicollinearity,Ridge regularization,Spatial cross-validation,Variable importance,Permutation tests
AI 理解论文
溯源树
样例
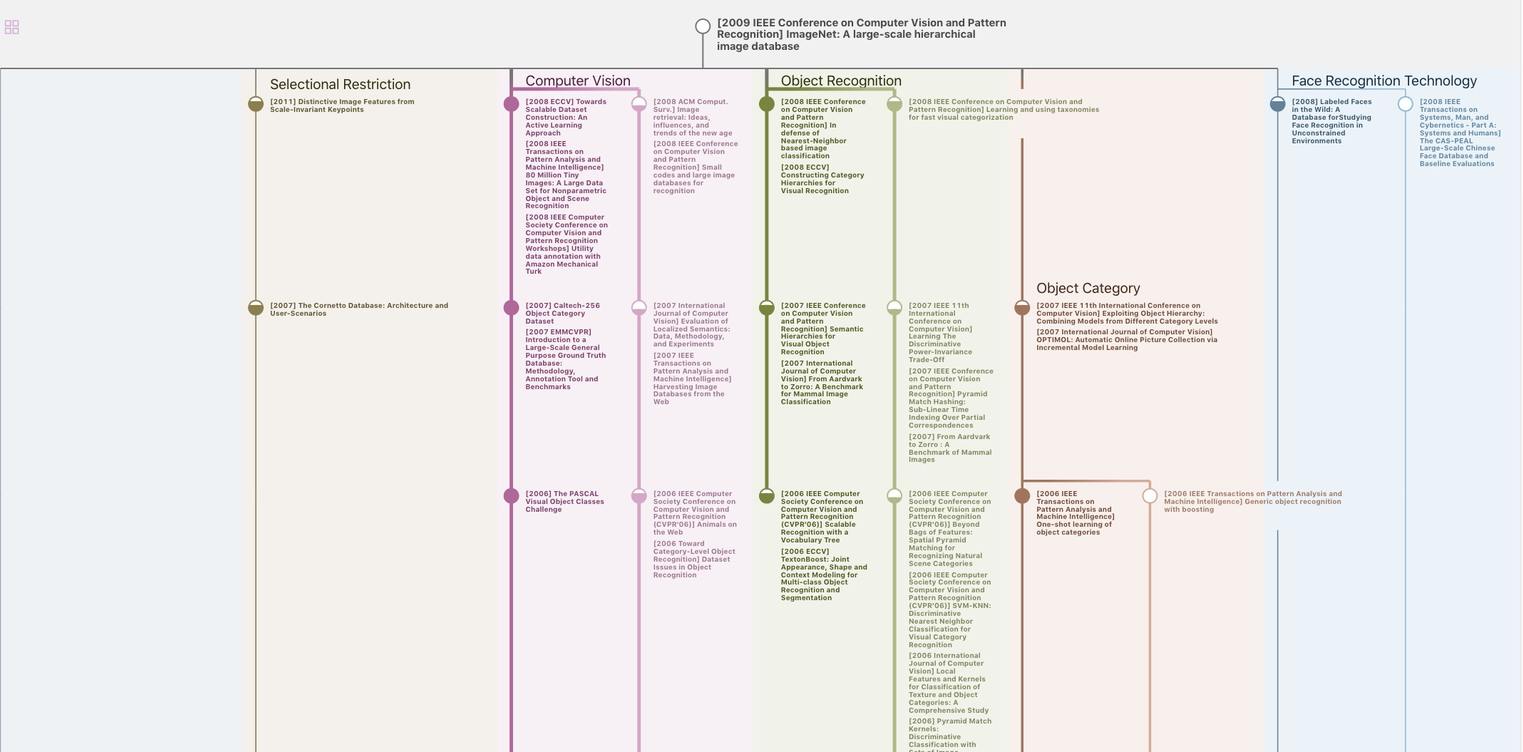
生成溯源树,研究论文发展脉络
Chat Paper
正在生成论文摘要