Channel Charting for Beam Management in Sub-THz Systems.
Asilomar Conference on Signals, Systems and Computers(2023)
摘要
Sub-THz communication is emerging as a promising technology to improve the cellular system capacity in the sixth-generation (6G) era. Compared to conventional microwave communication networks, sub-THz systems require a high degree of densification and support multi-connectivity to counter signal blockage. Efficient beam selection and user scheduling are two key problems for realizing multiuser sub- THz communication in practical systems. We focus on the problem of joint beam and user scheduling. To devise low-complexity scheduling scheme, we consider a machine learning (ML) based solution by casting the involved optimization problem as a statistical learning problem, which we parameterize via a deep neural network (DNN) architecture suitable for the problem at hand. To counter the large parameter space of the learning problem entailed by dealing with high-dimensional sub-THz CSI, we resort to a lower dimensional channel state information (CSI) representation which we extract via the Channel charting (CC) framework. CC is a machine learning framework which leverages dimensionality reduction algorithms to map high-dimensional channel state information (CSI) to a representative lower-dimensional feature space. Depending on the employed metric, the feature space may represent different properties encoded in the CSI, such as, pairwise spatial proximity or interference relationships. The channel charting framework plays the role of a feature extraction step, which is important to facilitate learning. We provide numerical evaluation of the developed ML based approach against a developed optimization based approach and the optimal exhaustive search solution. The proposed approach show a considerable achievable sum rate performance over the optimization based approach.
更多查看译文
关键词
Beam Management,sub-THz Systems,Machine Learning,Optimization Problem,Deep Neural Network,Feature Space,Feature Representation,Communication Network,Practical Systems,Learning Problem,Exhaustive Search,Low-dimensional Space,Deep Neural Network Architecture,Statistical Problems,Dimensionality Reduction Algorithms,Feature Extraction Step,User Scheduling,Low-dimensional Feature Space,Training Set,Covariance Matrix,User Equipment,Subgradient Method,Radio Resource Management,Finite Set,Lagrange Multiplier,Primal Variables,Additive Noise,Antenna Array,Dimensional Space,Multiple-input Multiple-output
AI 理解论文
溯源树
样例
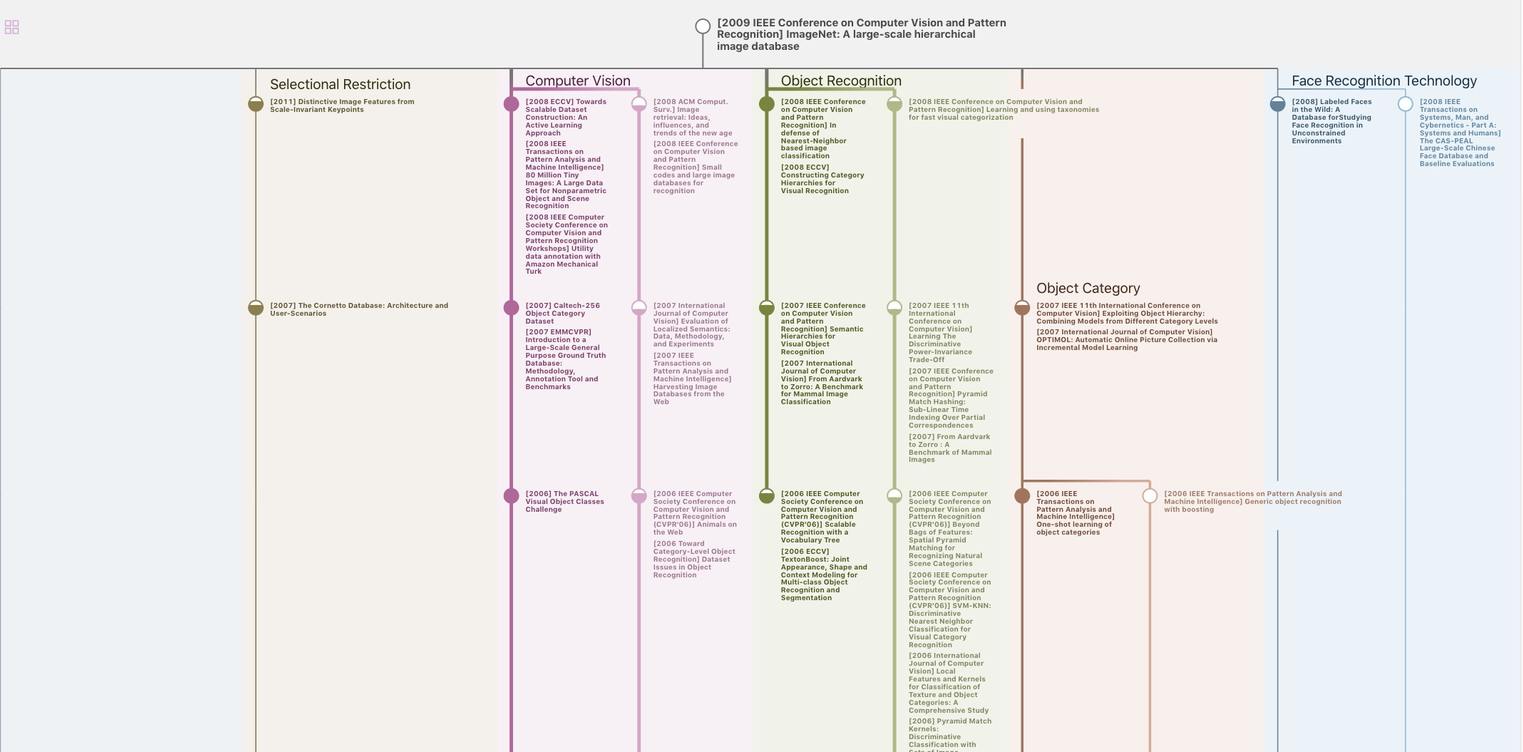
生成溯源树,研究论文发展脉络
Chat Paper
正在生成论文摘要