Addressing equifinality in agent-based modeling: a sequential parameter space search method based on sensitivity analysis
INTERNATIONAL JOURNAL OF GEOGRAPHICAL INFORMATION SCIENCE(2024)
摘要
This study addresses the challenge of equifinality in agent-based modeling (ABM) by introducing a novel sequential calibration approach. Equifinality arises when multiple models equally fit observed data, risking the selection of an inaccurate model. In the context of ABM, such a situation might arise due to limitations in data, such as aggregating observations into coarse spatial units. It can lead to situations where successfully calibrated model parameters may still result in reliability issues due to uncertainties in accurately calibrating the inner mechanisms. To tackle this, we propose a method that sequentially calibrates model parameters using diverse outcomes from multiple datasets. The method aims to identify optimal parameter combinations while mitigating computational intensity. We validate our approach through indoor pedestrian movement simulation, utilizing three distinct outcomes: (1) the count of grid cells crossed by individuals, (2) the number of people in each grid cell over time (fine grid) and (3) the number of people in each grid cell over time (coarse grid). As a result, the optimal calibrated parameter combinations were selected based on high test accuracy to avoid overfitting. This method addresses equifinality while reducing computational intensity of parameter calibration for spatially explicit models, as well as ABM in general.
更多查看译文
关键词
Agent-based modeling,equifinality,calibration,sequential calibration approach,sensitivity analysis
AI 理解论文
溯源树
样例
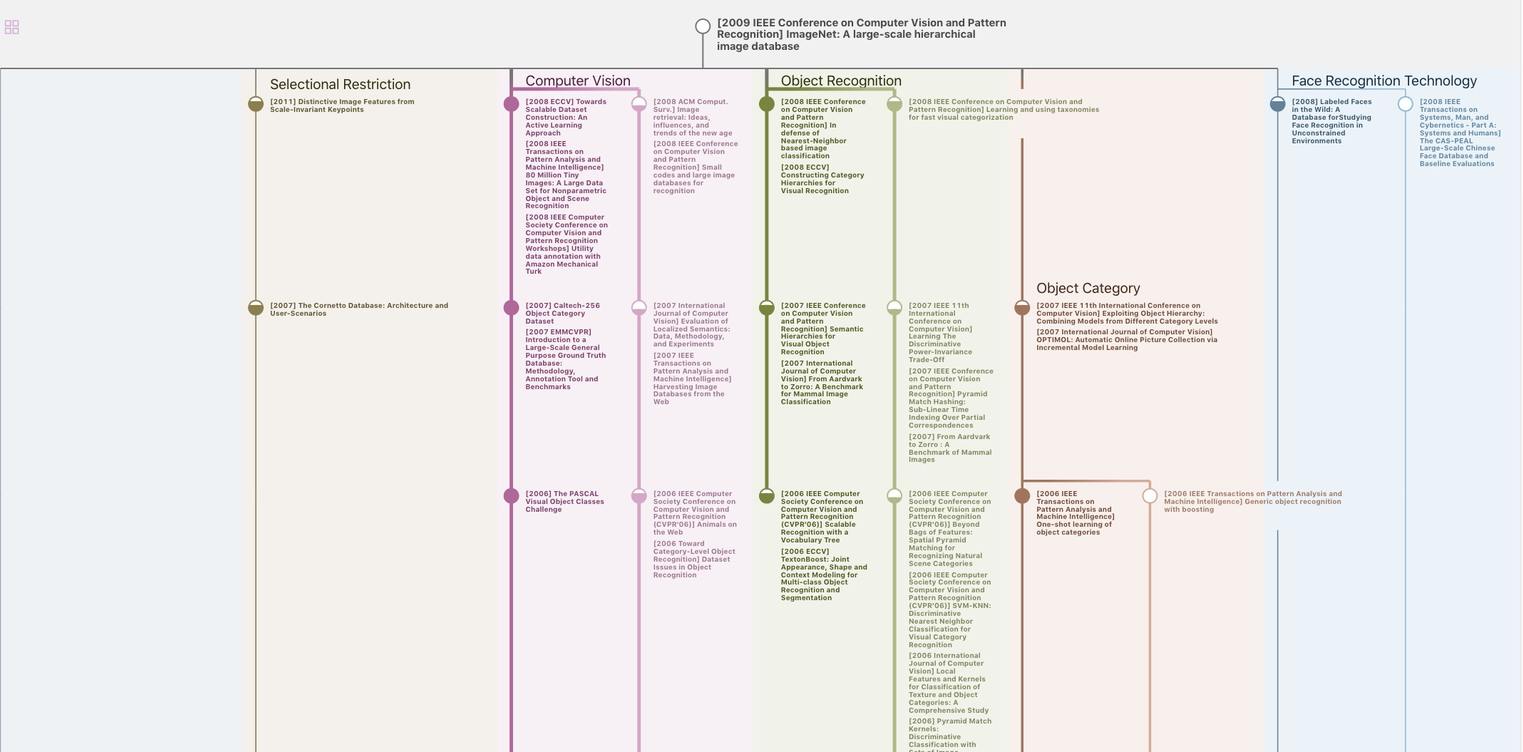
生成溯源树,研究论文发展脉络
Chat Paper
正在生成论文摘要