A Semiparametric Approach for Robust and Efficient Learning with Biobank Data
arxiv(2024)
摘要
With the increasing availability of electronic health records (EHR) linked
with biobank data for translational research, a critical step in realizing its
potential is to accurately classify phenotypes for patients. Existing
approaches to achieve this goal are based on error-prone EHR surrogate
outcomes, assisted and validated by a small set of labels obtained via medical
chart review, which may also be subject to misclassification. Ignoring the
noise in these outcomes can induce severe estimation and validation bias to
both EHR phenotyping and risking modeling with biomarkers collected in the
biobank. To overcome this challenge, we propose a novel unsupervised and
semiparametric approach to jointly model multiple noisy EHR outcomes with their
linked biobank features. Our approach primarily aims at disease risk modeling
with the baseline biomarkers, and is also able to produce a predictive EHR
phenotyping model and validate its performance without observations of the true
disease outcome. It consists of composite and nonparametric regression steps
free of any parametric model specification, followed by a parametric projection
step to reduce the uncertainty and improve the estimation efficiency. We show
that our method is robust to violations of the parametric assumptions while
attaining the desirable root-n convergence rates on risk modeling. Our
developed method outperforms existing methods in extensive simulation studies,
as well as a real-world application in phenotyping and genetic risk modeling of
type II diabetes.
更多查看译文
AI 理解论文
溯源树
样例
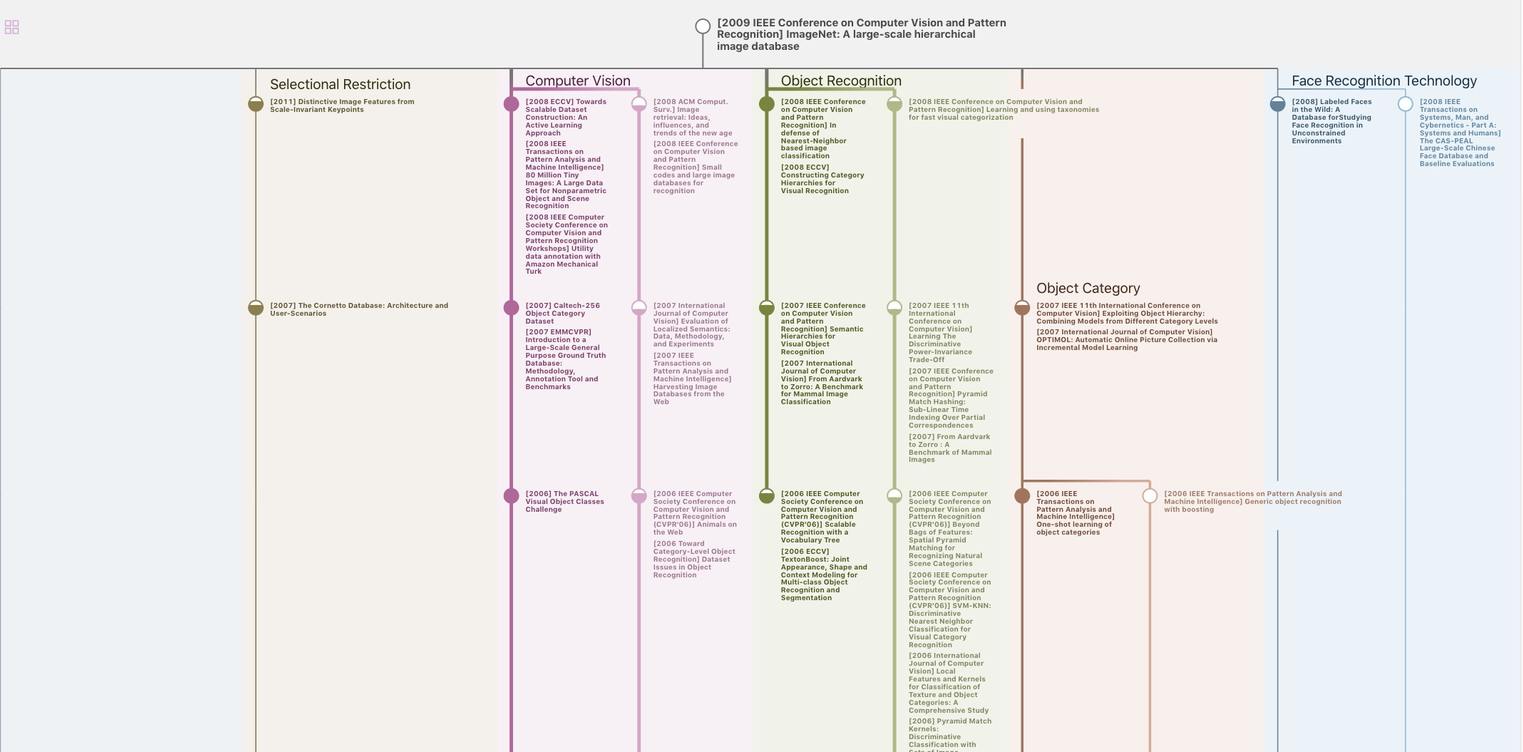
生成溯源树,研究论文发展脉络
Chat Paper
正在生成论文摘要