Transforming the Synthesis of Carbon Nanotubes with Machine Learning Models and Automation
arxiv(2024)
摘要
Carbon-based nanomaterials (CBNs) are showing significant potential in
various fields, such as electronics, energy, and mechanics. However, their
practical applications face synthesis challenges stemming from the complexities
of structural control, large-area uniformity, and high yield. Current research
methodologies fall short in addressing the multi-variable, coupled interactions
inherent to CBNs production. Machine learning methods excel at navigating such
complexities. Their integration with automated synthesis platforms has
demonstrated remarkable potential in accelerating chemical synthesis research,
but remains underexplored in the nanomaterial domain. Here we introduce Carbon
Copilot (CARCO), an artificial intelligence (AI)-driven platform that
integrates transformer-based language models tailored for carbon materials,
robotic chemical vapor deposition (CVD), and data-driven machine learning
models, empowering accelerated research of CBNs synthesis. Employing CARCO, we
demonstrate innovative catalyst discovery by predicting a superior
Titanium-Platinum bimetallic catalyst for high-density horizontally aligned
carbon nanotube (HACNT) array synthesis, validated through over 500
experiments. Furthermore, with the assistance of millions of virtual
experiments, we achieved an unprecedented 56.25
HACNT arrays with predetermined densities in the real world. All were
accomplished within just 43 days. This work not only advances the field of
HACNT arrays but also exemplifies the integration of AI with human expertise to
overcome the limitations of traditional experimental approaches, marking a
paradigm shift in nanomaterials research and paving the way for broader
applications.
更多查看译文
AI 理解论文
溯源树
样例
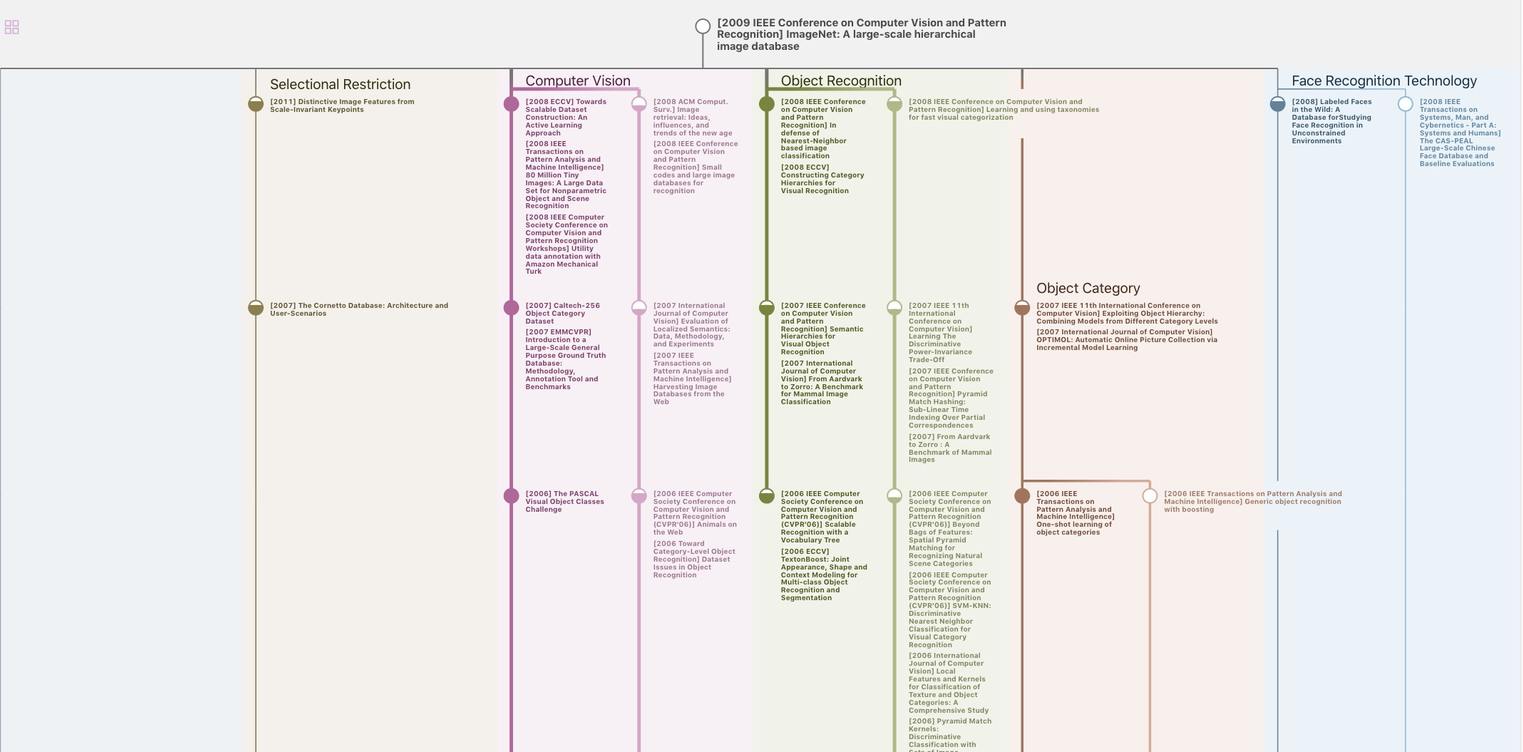
生成溯源树,研究论文发展脉络
Chat Paper
正在生成论文摘要