A chance for models to show their quality: Stochastic process model-log dimensions
Information Systems(2024)
摘要
Process models describe the desired or observed behaviour of organisations. In stochastic process mining, computational analysis of trace data yields process models which describe process paths and their probability of execution. To understand the quality of these models, and to compare them, quantitative quality measures are used.This research investigates model comparison empirically, using stochastic process models built from real-life logs. The experimental design collects a large number of models generated randomly and using process discovery techniques. Twenty-five different metrics are taken on these models, using both existing process model metrics and new, exploratory ones. The results are analysed quantitatively, making particular use of principal component analysis.Based on this analysis, we suggest three stochastic process model dimensions: adhesion, relevance and simplicity. We also suggest possible metrics for these dimensions, and demonstrate their use on example models.
更多查看译文
关键词
Stochastic process mining,Process conformance,Stochastic Petri nets,Adhesion,Relevance,Simplicity
AI 理解论文
溯源树
样例
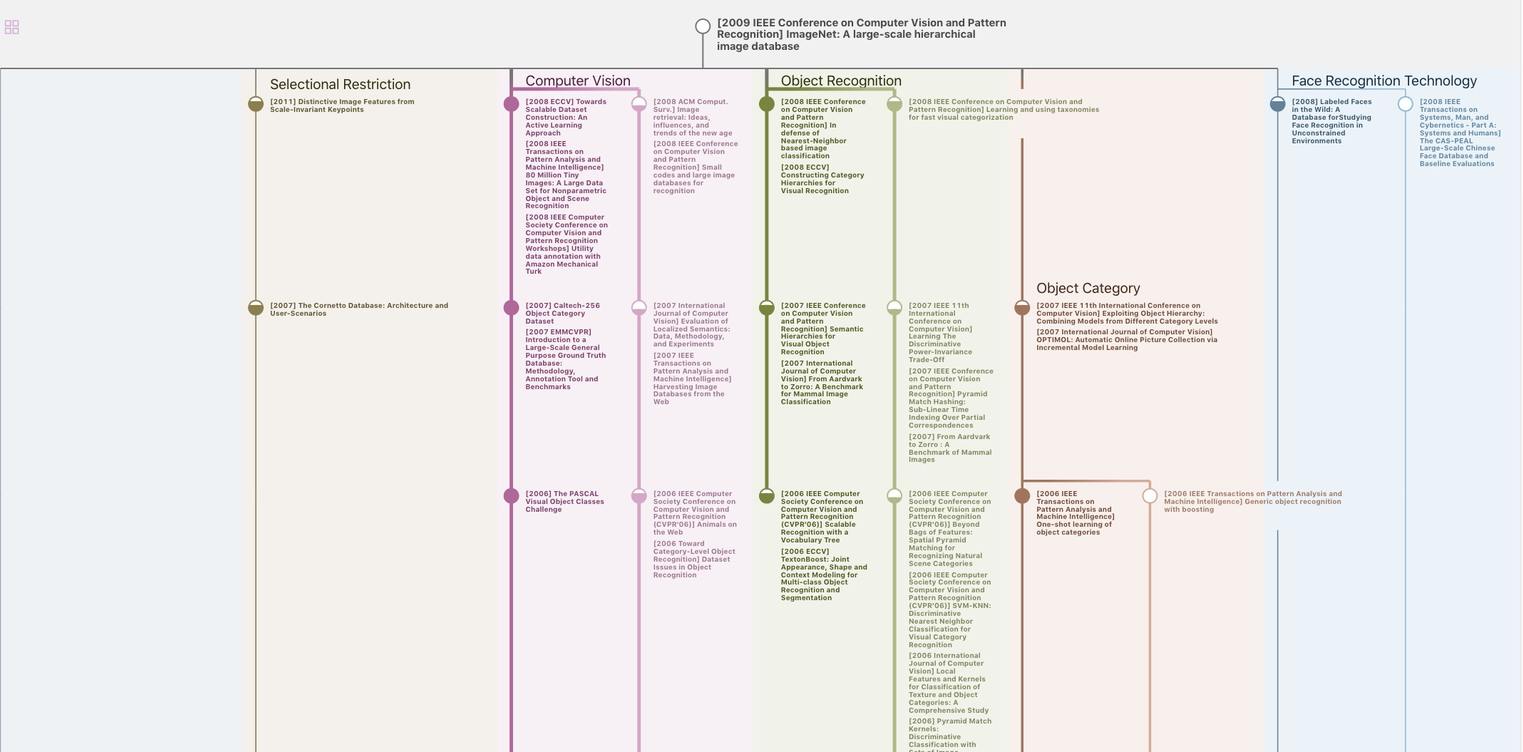
生成溯源树,研究论文发展脉络
Chat Paper
正在生成论文摘要