Feature selection based on dynamic crow search algorithm for high-dimensional data classification
Expert Systems with Applications(2024)
摘要
High-dimensional biomedical data plays an important role in disease diagnosis and classification. To analyze the high-dimensional biomedical data, machine learning algorithms are widely used. However, a great amount of redundant, irrelevant or weak correlation features are prevalent in these datasets, which significantly deteriorate the capability of machine learning techniques. It is necessary to select the most informative features and discard the useless ones for enhancing the classification accuracy and reducing the dimensionality. To address this issue, an improved Crow Search Algorithm (CSA) which is known as the Dynamic CSA (DCSA) is proposed to find the optimal feature subset in high-dimensional classification tasks. In DCSA, three modifications are investigated. Firstly, we propose a dynamic bi-level awareness probability to guide the transition between global search and local search. Consequently, the balance between exploration and exploitation is improved. Secondly, levy flight with a unfixed step length control parameter is employed as the global search and the random search mechanism of the original CSA is abandoned. Thirdly, a dynamic flight length strategy is adopted to enhance the local search and speed up convergence. All in all, DCSA is developed as a wrapper feature selection model, where KNN classifier is applied as the feature subset evaluator. The performance of DCSA is measured on seven high-dimensional biomedical datasets. Experimental results show that DCSA outperforms other state-of-the-art methods. Specifically, DCSA achieved the highest average accuracy in six data. In terms of numbers of selected features, DCSA gained the smallest subset size in a seven data. And for SRBCT data, the prediction accuracy of DCSA is at 100%.
更多查看译文
关键词
Feature selection,High-dimensional data classification,Crow search algorithm
AI 理解论文
溯源树
样例
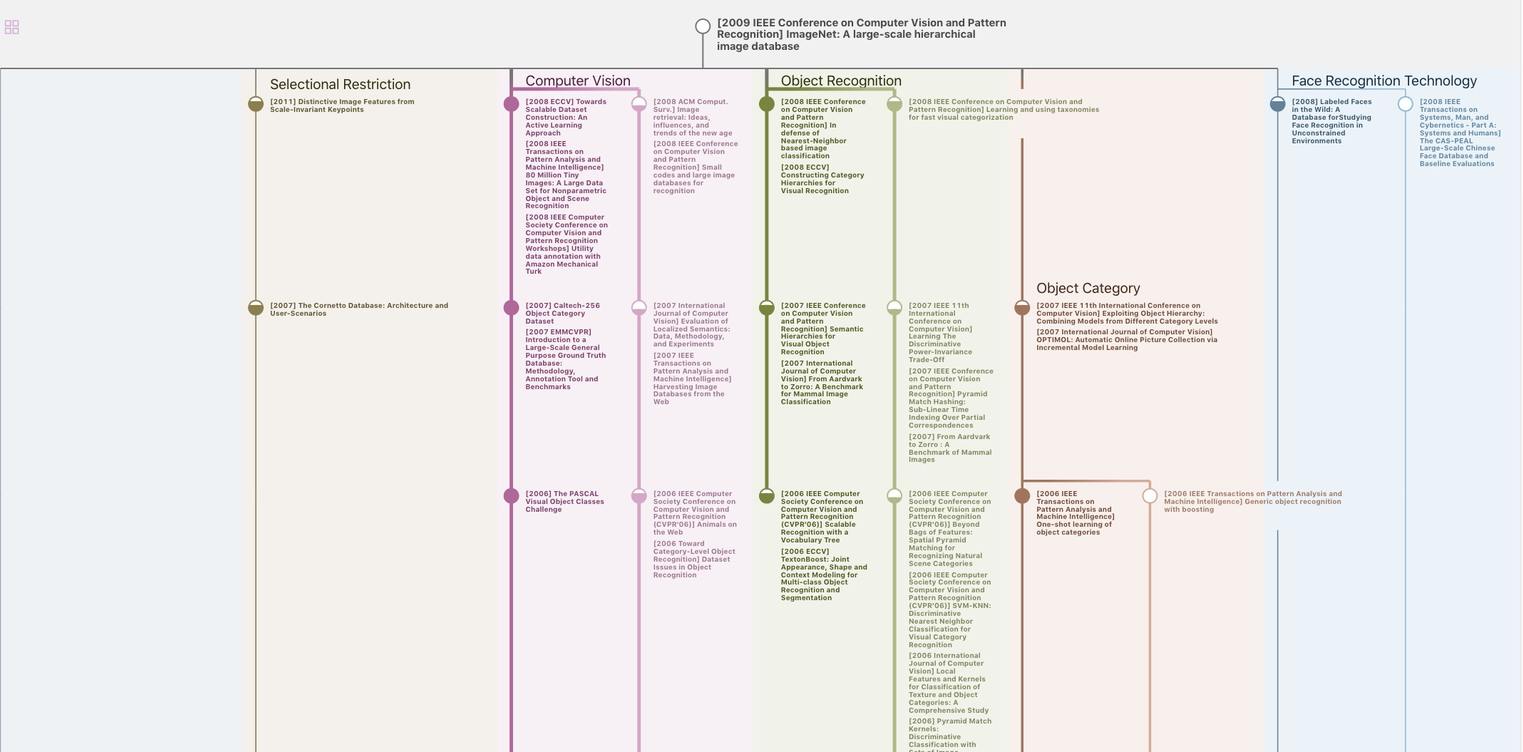
生成溯源树,研究论文发展脉络
Chat Paper
正在生成论文摘要