Efficient Feature Selection Techniques for Accurate Cancer Analysis Using Krill Heard Optimization
2024 International Conference on Emerging Systems and Intelligent Computing (ESIC)(2024)
摘要
In this research, we introduce a novel biologically-inspired optimization algorithm known as Krill Herd (KH) to address feature selection challenges in cancer analysis. Inspired by the collective behaviour of krill individuals, the KH algorithm optimizes feature subsets by minimizing individual krill distances from food sources and herd density. We model the dynamic positions of krill individuals considering factors such as interactions with peers, foraging behaviour, and random diffusion. To enhance the algorithm’s performance, we incorporate adaptive genetic operators. Our method’s efficiency is evaluated across various benchmark optimization problems, demonstrating its capability to outperform eight established algorithms. In parallel, we delve into the critical domain of Cancer Feature Selection and classification in computational molecular biology. Identifying cancer-causing genes is pivotal for precise treatments and drug discovery. To address these challenges, we propose an enhanced Krill Herd Optimization (KHO) technique for gene selection, combined with Random Forest (RF) for classification. RF is chosen for its high classification accuracy. This research advances the field of cancer analysis by offering efficient and accurate feature selection techniques, providing insights into cancer-related genes, and facilitating more precise treatments and drug discoveries.
更多查看译文
关键词
Krill Herd Optimization (KHO),Feature Selection,Cancer Classification,Gene Selection,Random Forest (RF)
AI 理解论文
溯源树
样例
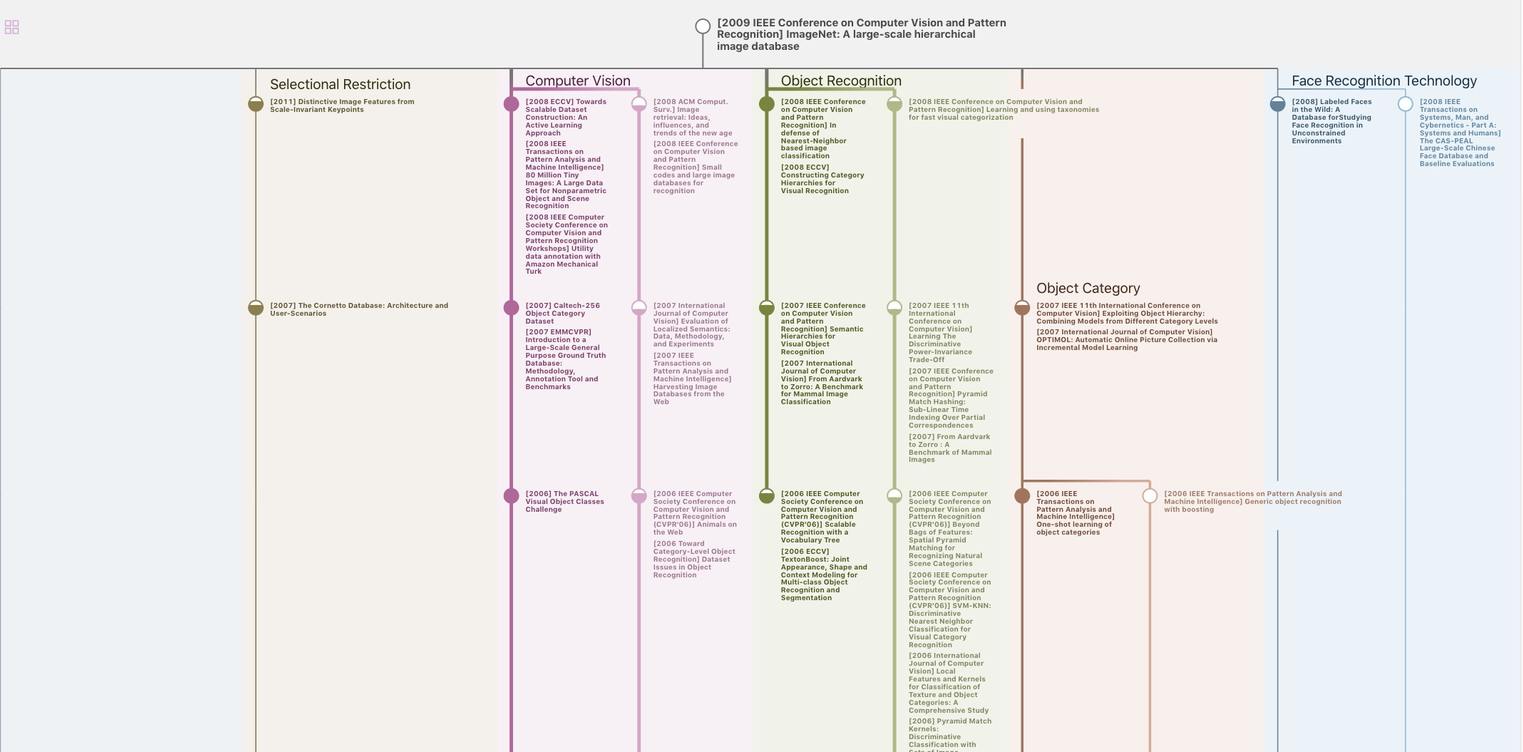
生成溯源树,研究论文发展脉络
Chat Paper
正在生成论文摘要