Comparative analysis of the performance of supervised learning algorithms for photovoltaic system fault diagnosis
SCIENCE AND TECHNOLOGY FOR ENERGY TRANSITION(2024)
摘要
New trends were introduced in using PhotoVoltaic (PV) energy which are mostly attributable to new laws internationally having a goal to decrease the usage of fossil fuels. The PV systems efficiency is impacted significantly by environmental factors and different faults occurrence. These faults if they were not rapidly identified and fixed may cause dangerous consequences. A lot of methods have been introduced in the literature to detect faults that may occur in a PV system such as using Current-Voltage (I-V) curve measurements, atmospheric models and statistical methods. In this paper, various machine learning techniques in particular supervised learning techniques are used for PV array failure diagnosis. The main target is the identification and categorization of several faults that may occur such as shadowing, degradation, open circuit and short circuit faults that have a great impact on PV systems performance. The results showed the technique's high ability of fault diagnosis capability. The K-Nearest Neighbor (KNN) technique showed the best fault prediction performance. It achieves prediction accuracy of 99.2% and 99.7% Area Under Curve-Receiver Operating Curve (AUC-ROC) score. This shows its superiority in fault prediction in PV systems over other used methods Decision Tree, Na & iuml;ve Bayes, and Logistic Regression.
更多查看译文
关键词
PV,Machine learning,Logistic regression,Decision tree
AI 理解论文
溯源树
样例
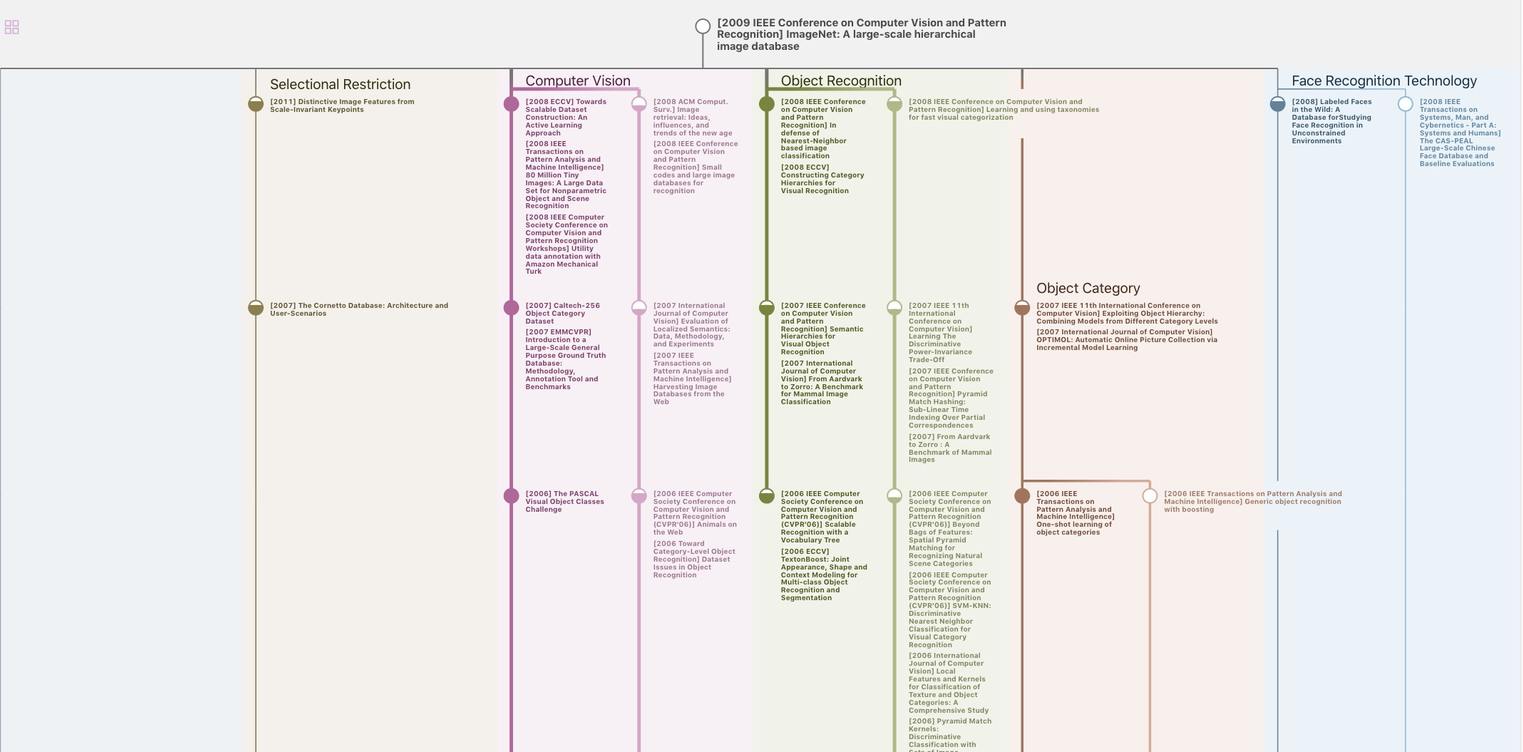
生成溯源树,研究论文发展脉络
Chat Paper
正在生成论文摘要