Enhancing High-Resolution Image Compression Through Local-Global Joint Attention Mechanism
IEEE Signal Processing Letters(2024)
摘要
Deep learning-based image compression algorithms have witnessed remarkable advancements. However, compressing high-resolution images often suffers from color bias and joint seams between reconstructed patches as it ignores the global features of whole images. To address this issue, we propose a novel image compression autoencoder based on the local-global joint attention mechanism (CAE-LG). Specifically, CAE-LG extracts local and global features, and then refines and fuses these two features to obtain the reconstructed image. We introduce a feature attention block that leverages dynamic convolution and attention layers to enhance the encoding and decoding of feature maps. We validated our method on datasets comprising both natural and medical images. Experimental results demonstrate that our proposed approach outperforms the existing image compression methods. In addition, performance improvements on different datasets show the generality of our model. Ablation studies confirm the effectiveness of both the joint learning of local and global features and the feature attention block.
更多查看译文
关键词
Image compression,dynamic convolution,joint learning,deep learning
AI 理解论文
溯源树
样例
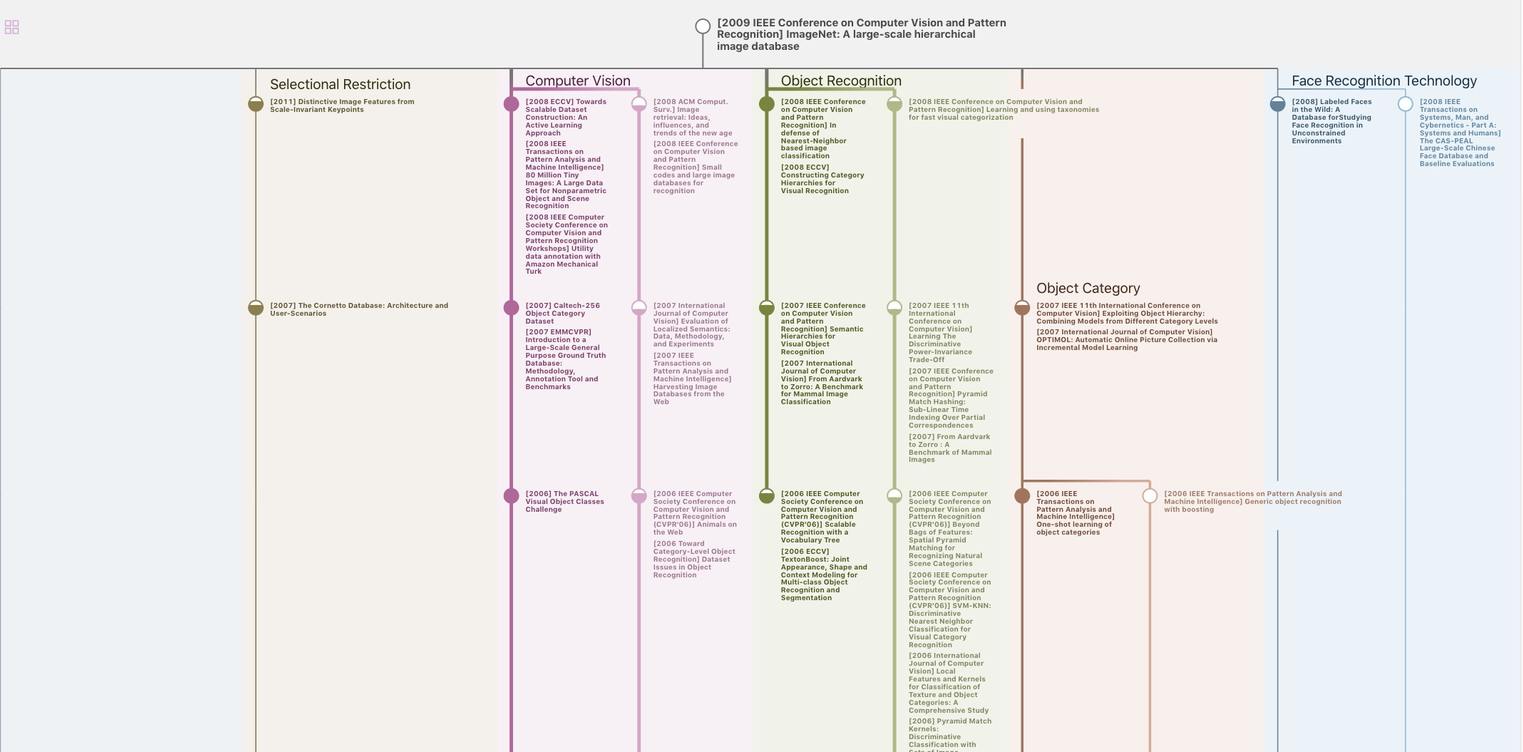
生成溯源树,研究论文发展脉络
Chat Paper
正在生成论文摘要