Physics-Driven Learned Deconvolution of Multi-Spectral Cellular MRI with Radial Sampling.
Asilomar Conference on Signals, Systems and Computers(2023)
摘要
In fluorine-19 (
19
F) cellular MRI, detection of multiple cell targets requires the ability to unmix images corresponding to different tracer molecules with different chemical shifts. The resulting chemical shift artifacts of conventional Cartesian sampling are well-defined, appearing as ‘ghost images’ along the readout direction. However, a key challenge with radial sampling is that frequency offsets lead to nonlinear smearing artifacts throughout the image. Thus, proper modeling of forward sensing operators is crucial, as the successful deconvolution of artifacts relies on the joint design of acquisition scheme and sampling pattern. In this work, we aim to address these perspectives through the lens of radial spectral deconvolution. Our goal is to develop suitable modeling of the radial chemical shift artifacts using Radon transform, which will guide our design of sensing operators that favor specific multi-spectral imaging tasks. To effectively unmix the component images under low SNR regime, we will further exploit physics-informed learning-based unrolling strategies that enable simultaneous artifact removal and weak signal detection, both of particular interest in
19
F MRI. particular interest in
19
F MRI. 1
更多查看译文
关键词
Radial Sampling,Chemical Shifts,Weak Signal,Multispectral Images,Spectral Deconvolution,Weak Detection,Radon Transform,Tracer Molecules,Ghost Imaging,National Institutes Of Health,Fourier Transform,Convolutional Neural Network,Image Object,Pulse Sequence,Noisy Data,Data-driven Methods,Bessel Function,Point Spread Function,Amount Of Shift,Total Variation Regularization,Proximal Operator,Extreme Regimes,Conventional Reconstruction
AI 理解论文
溯源树
样例
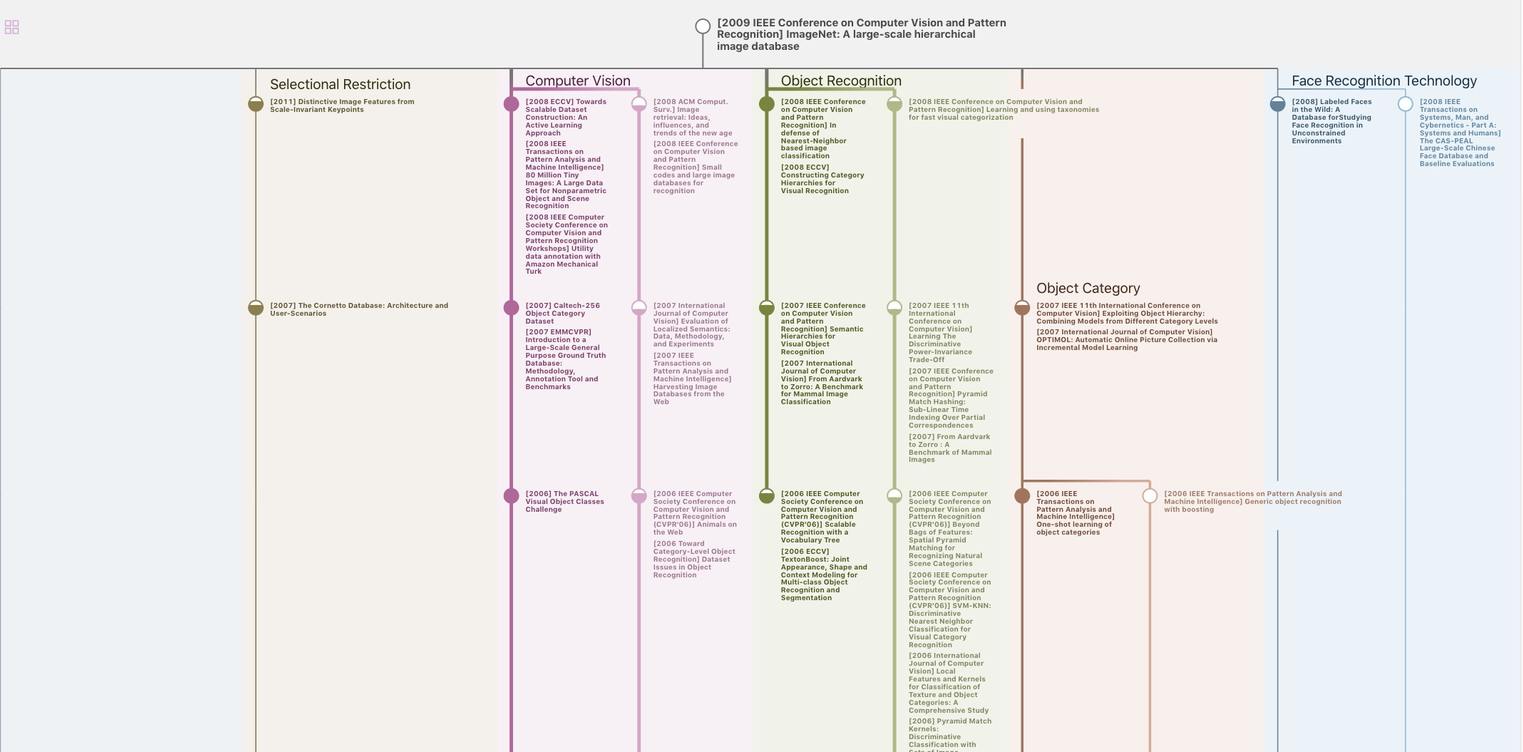
生成溯源树,研究论文发展脉络
Chat Paper
正在生成论文摘要