Comparative study of physics-based model and machine learning model for epidemic forecasting and countermeasure
Computational and Applied Mathematics(2024)
摘要
Forecasting the transmission patterns of infectious diseases is of paramount importance in gaining valuable insights into outbreak growth and optimizing the allocation of medical resources. In this paper, we conduct a comparative study between a physics-based model and a machine learning (ML) model for epidemic forecasting and countermeasures, considering criteria such as accuracy and practicality. We develop four ML models: back-propagation (BP), long short-term memory, support vector machine, and extreme learning machine. In addition, we propose a reaction–diffusion (R–D) model that incorporates factors such as susceptibility heterogeneity, lockdown measures, population movement, and dynamically dependent rates. The experimental results highlight the superior accuracy of the BP model for forecasting, while the R–D model provides comprehensive insights into disease dynamics, including stability and potential control strategies.
更多查看译文
关键词
Physics-based model,Machine learning model,Disease dynamics,Forecasting,Countermeasure,35B05,92D30
AI 理解论文
溯源树
样例
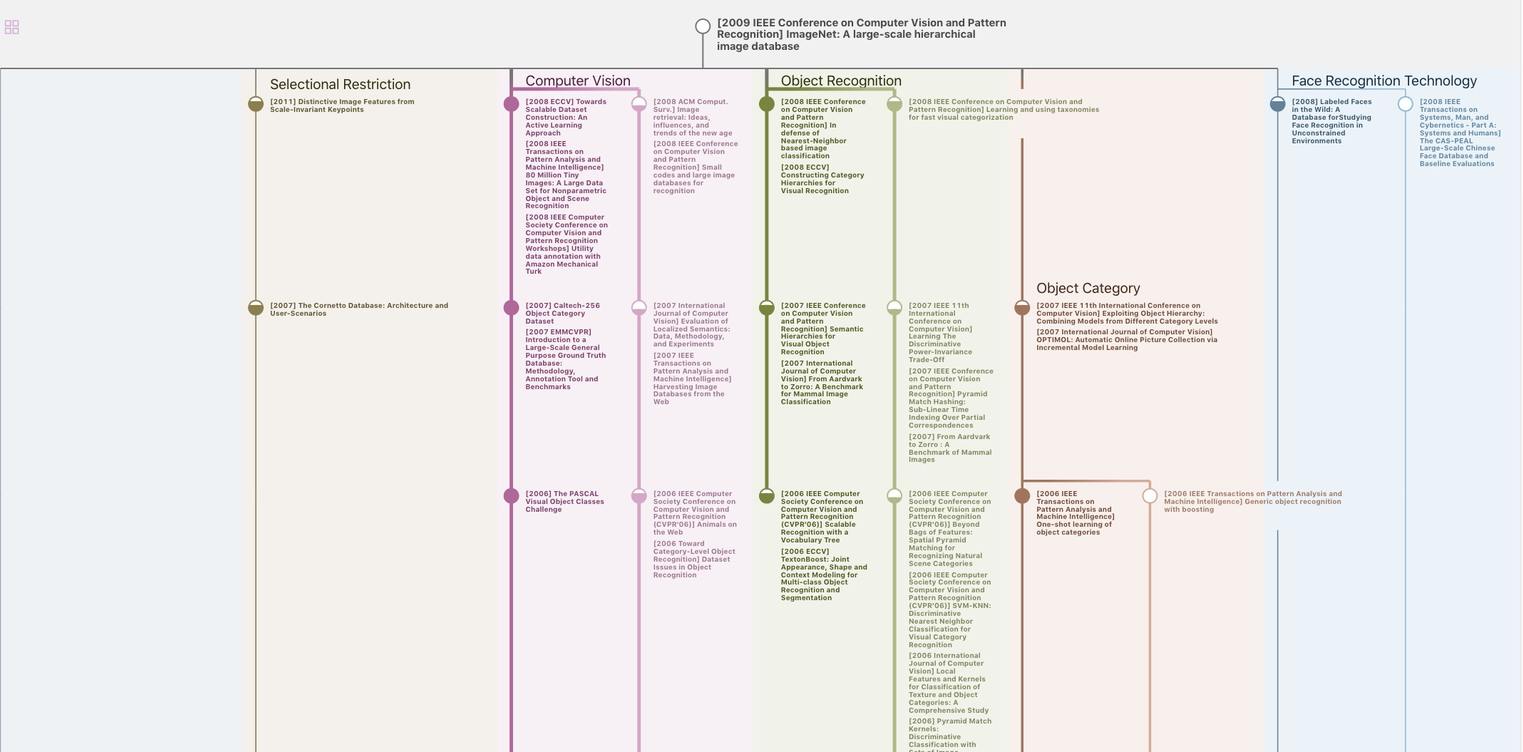
生成溯源树,研究论文发展脉络
Chat Paper
正在生成论文摘要