Pruning‐guided feature distillation for an efficient transformer‐based pose estimation model
IET Computer Vision(2024)
摘要
AbstractThe authors propose a compression strategy for a 3D human pose estimation model based on a transformer which yields high accuracy but increases the model size. This approach involves a pruning‐guided determination of the search range to achieve lightweight pose estimation under limited training time and to identify the optimal model size. In addition, the authors propose a transformer‐based feature distillation (TFD) method, which efficiently exploits the pose estimation model in terms of both model size and accuracy by leveraging transformer architecture characteristics. Pruning‐guided TFD is the first approach for 3D human pose estimation that employs transformer architecture. The proposed approach was tested on various extensive data sets, and the results show that it can reduce the model size by 30% compared to the state‐of‐the‐art while ensuring high accuracy.
更多查看译文
AI 理解论文
溯源树
样例
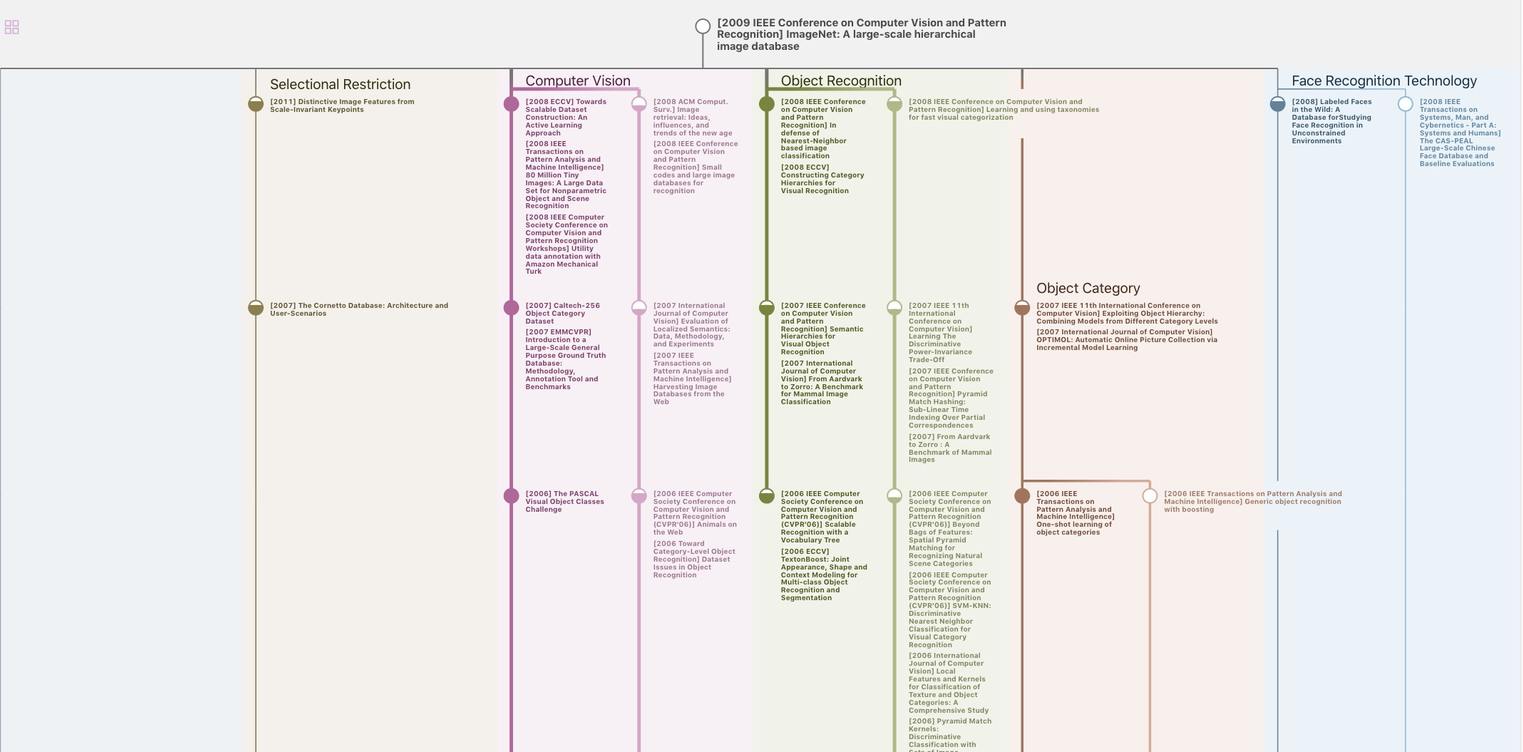
生成溯源树,研究论文发展脉络
Chat Paper
正在生成论文摘要