Neural Network-based model of galaxy power spectrum: Fast full-shape galaxy power spectrum analysis
arxiv(2024)
摘要
We present a Neural Network based emulator for the galaxy redshift-space
power spectrum that enables several orders of magnitude acceleration in the
galaxy clustering parameter inference, while preserving 3σ accuracy
better than 0.5% up to k_max=0.25h^-1Mpc within ΛCDM
and around 0.5% w_0-w_aCDM. Our surrogate model only emulates the galaxy
bias-invariant terms of 1-loop perturbation theory predictions, these terms are
then combined analytically with galaxy bias terms, counter-terms and stochastic
terms in order to obtain the non-linear redshift space galaxy power spectrum.
This allows us to avoid any galaxy bias prescription in the training of the
emulator, which makes it more flexible. Moreover, we include the redshift z
∈ [0,1.4] in the training which further avoids the need for re-training the
emulator. We showcase the performance of the emulator in recovering the
cosmological parameters of ΛCDM by analysing the suite of 25
AbacusSummit simulations that mimic the DESI Luminous Red Galaxies at z=0.5
and z=0.8, together as the Emission Line Galaxies at z=0.8. We obtain
similar performance in all cases, demonstrating the reliability of the emulator
for any galaxy sample at any redshift in 0 < z < 1.4
更多查看译文
AI 理解论文
溯源树
样例
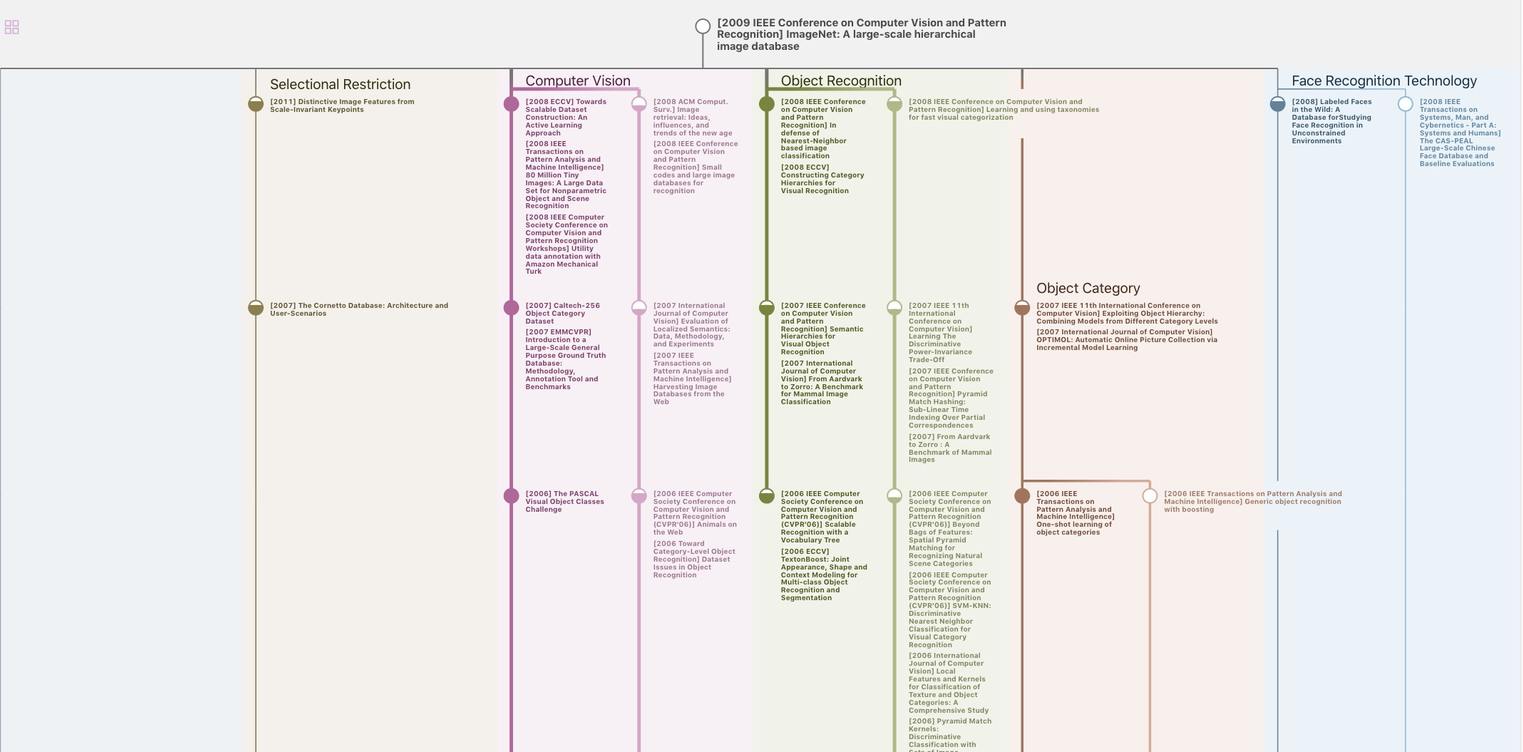
生成溯源树,研究论文发展脉络
Chat Paper
正在生成论文摘要