Hierarchical spatial–temporal autocorrelation graph neural network for online wind turbine pitch system fault detection
Neurocomputing(2024)
摘要
The advancement of low-cost, non-manually labeled big data technologies for fault detection in wind turbines is crucial to guarantee their safe and efficient operation. In this context, Normal Behavior Modelling (NBM) is a prevalent approach. However, existing NBM methods for wind turbine fault detection inadequately utilize complex spatial–temporal correlations within the Supervisory Control and Data Acquisition (SCADA) during the normal behavior prediction stage.To cope with this limitation, this paper propose a hierarchical spatial–temporal autocorrelation graph neural network for online wind turbine pitch system fault detection. This approach integrates a dynamic graph network with an adaptive structure for spatial correlation learning and a transformer model with autocorrelation attention to identify periodic time-distance correlations. Furthermore, a hierarchical neural network, specifically designed for wind turbine physical structure, enhances local information extraction through seven latent graph neural networks and a probsparse attention mechanism. The proposed method demonstrates significant effectiveness in real-world applications, as evidenced by data from a Shanghai wind farm.
更多查看译文
关键词
Wind turbine,Pitch system,Online fault detection,Hierarchical,Spatial–temporal,Autocorrelation,Graph neural network
AI 理解论文
溯源树
样例
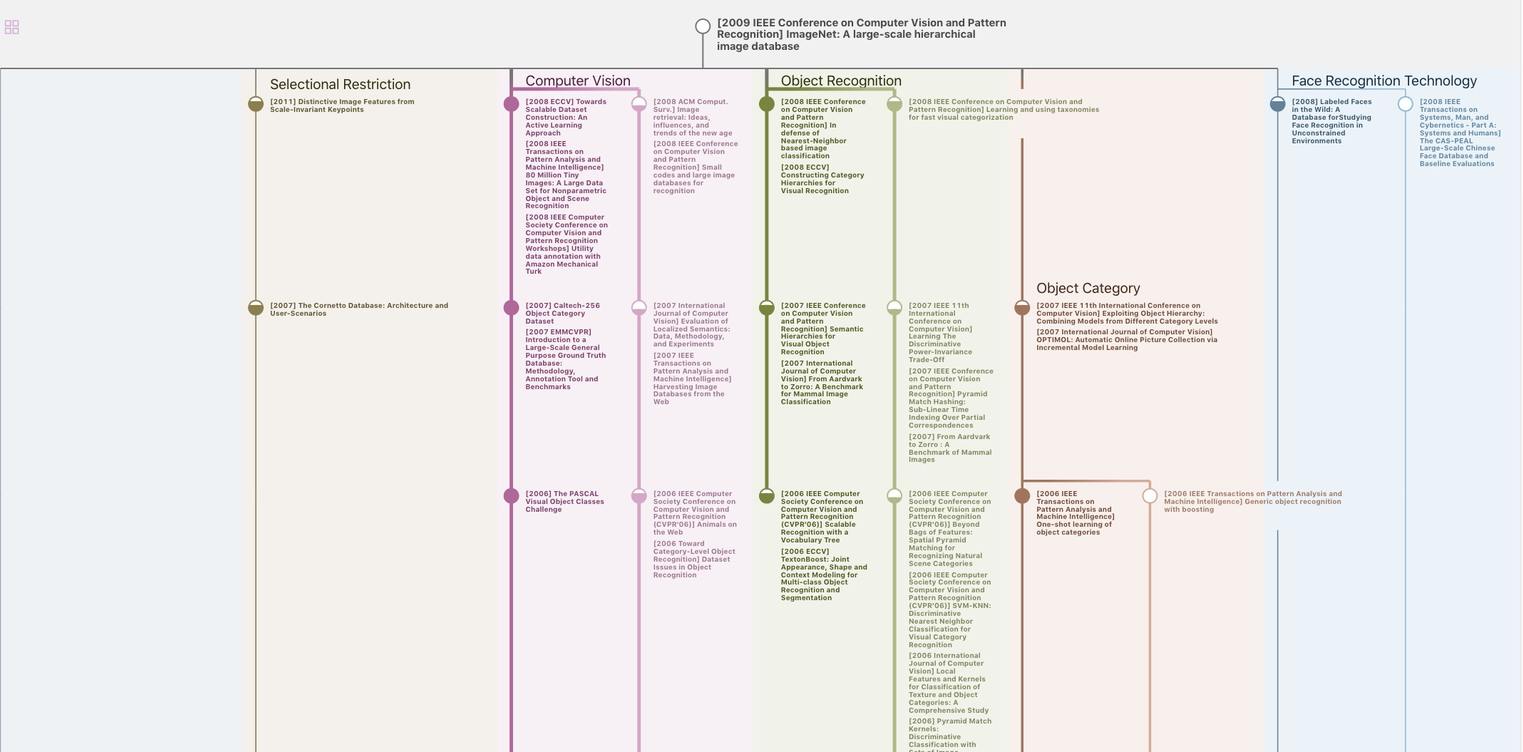
生成溯源树,研究论文发展脉络
Chat Paper
正在生成论文摘要