Deep Embedding Clustering Based on Residual Autoencoder
Neural Processing Letters(2024)
摘要
Clustering algorithm is one of the most widely used and influential analysis techniques. With the advent of deep learning, deep embedding clustering algorithms have rapidly evolved and yield promising results. Much of the success of these algorithms depends on the potential expression captured by the autoencoder network. Therefore, the quality of the potential expression directly determines the algorithm’s performance. In view of this, researchers have proposed many improvements. Although the performance has been slightly improved, they all have one shortcoming, that is, too much emphasis is placed on the original data reconstruction ability during the process of feature expression, which greatly limits the further expression of potential features according to specific clustering tasks. Moreover, there is a large amount of noise in the original data, so blindly emphasizing reconstruction will only backfire. Hence, we innovatively propose a deep embedding clustering algorithm based on residual autoencoder (DECRA) after in-depth research. Specifically, a novel autoencoder network with residual structure is proposed and introduced into deep embedded clustering tasks. The network introduces an adaptive weight layer in feature representation z, which can make it have good robustness, generalization for specific tasks, and adaptive learning of better feature embeddings according to category classification. In this paper, the reasons for the validity of this structure are explained theoretically, and comprehensive experiments on six benchmark datasets including various types show that the clustering performance of the DECRA is very competitive and significantly superior to the most advanced methods.
更多查看译文
关键词
Deep embedded clustering,Residual structure,Autoencoder network,Representation learning
AI 理解论文
溯源树
样例
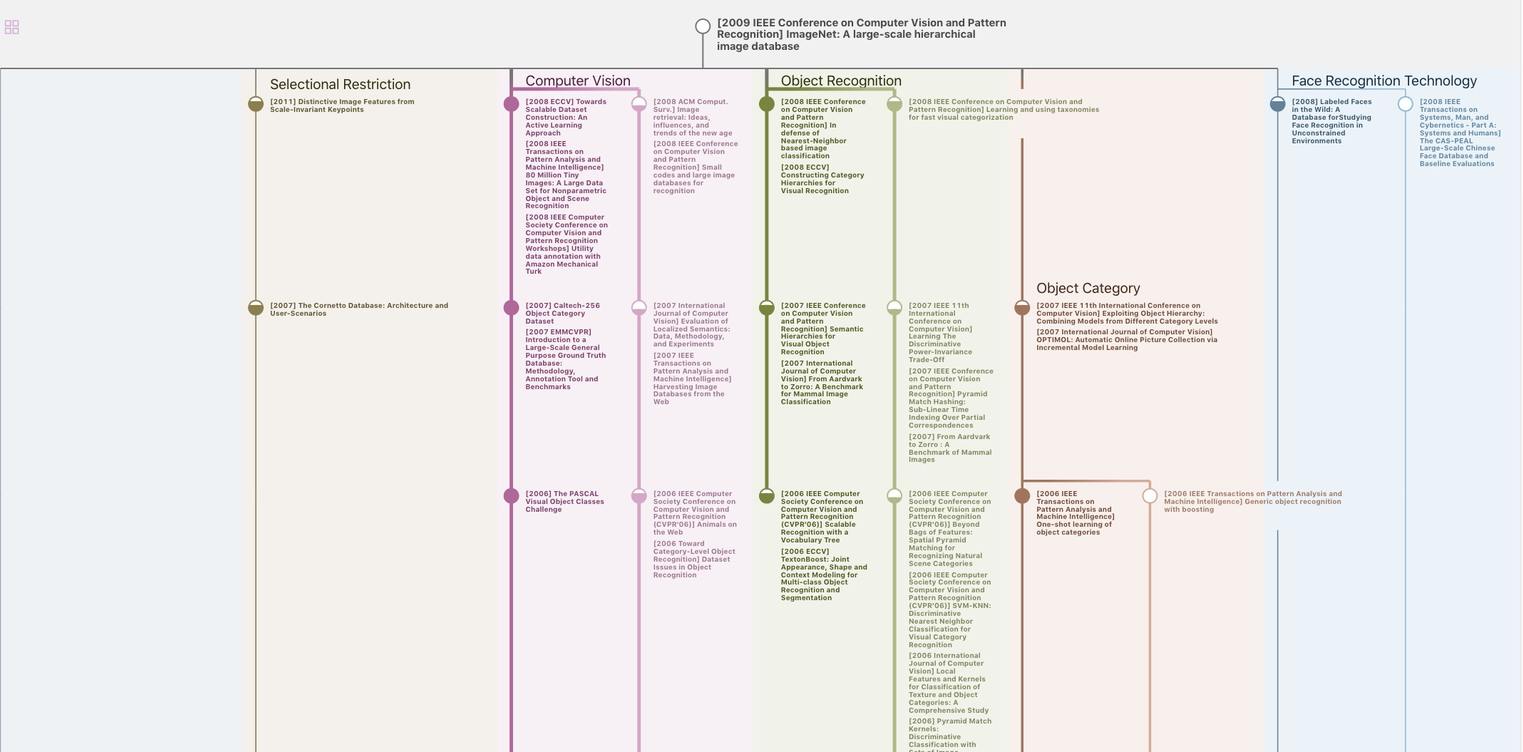
生成溯源树,研究论文发展脉络
Chat Paper
正在生成论文摘要