Condensing Molecular Docking CNNs via Knowledge Distillation
crossref(2024)
摘要
Knowledge of the bound protein-ligand structure is critical to many drug discovery tasks. One tool for in silico bound structure elucidation is molecular docking, which samples and scores ligand binding conformations. Recent work has demonstrated that convolutional neural networks (CNNs) for protein-ligand pose scoring outperform conventional scoring functions. Scoring performance can be further increased by taking the average of multiple CNN models, termed ensembles. However, ensembles of large parameter models require significant computational resources and therefore are difficult to apply to high-throughput molecular docking for virtual screening. We investigate knowledge distillation as a framework to condense the knowledge of large, powerful CNN model ensembles into a single reduced CNN model for a significant reduction in computational cost. Ensemble KD produces single models that outperform non-KD trained single models.
更多查看译文
AI 理解论文
溯源树
样例
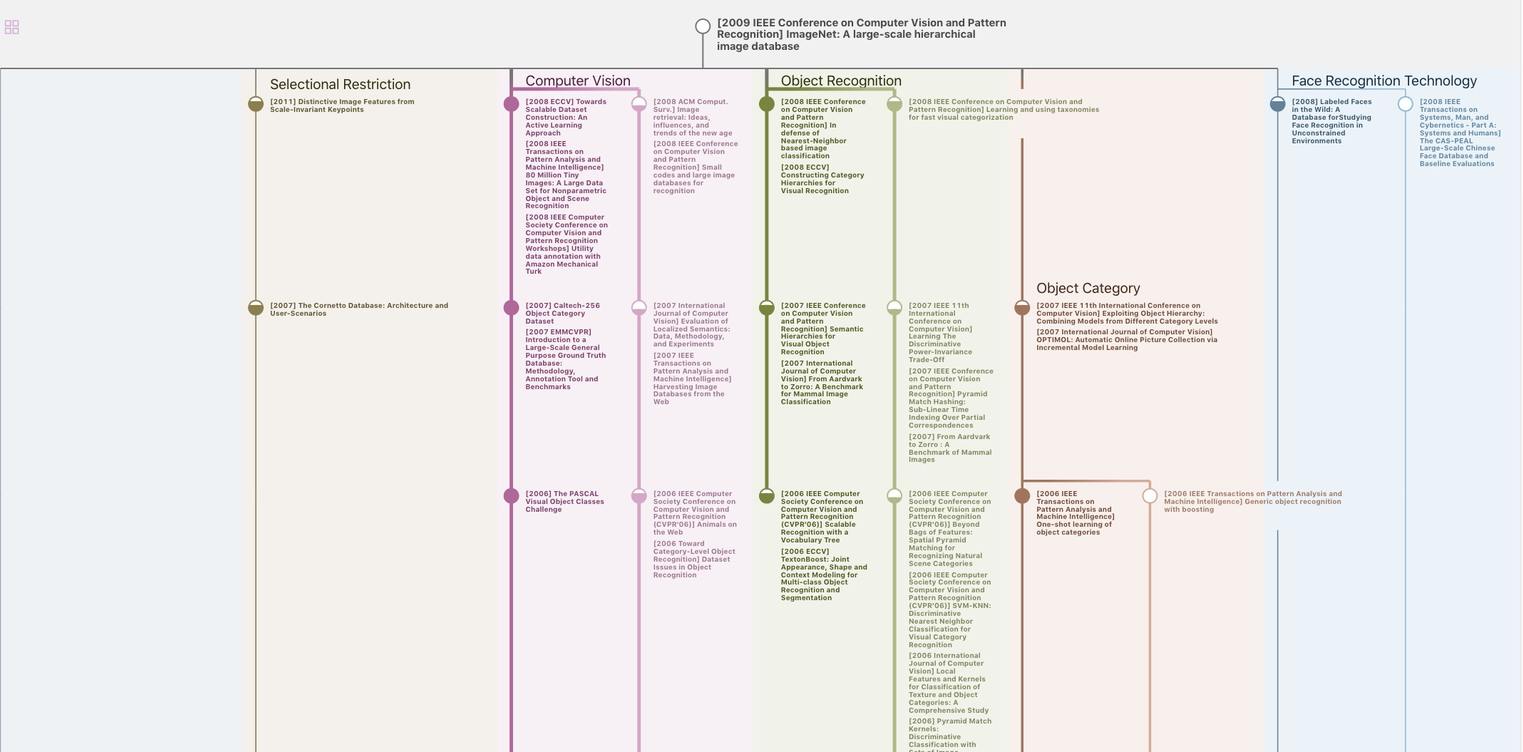
生成溯源树,研究论文发展脉络
Chat Paper
正在生成论文摘要