On Feature Selection Techniques for Detecting DoS Attacks with a Multi-class Classifier
crossref(2024)
摘要
In a hyperconnected society, computer networks play a pivotal role in all activities that permeate our daily lives. In the context of smart cities, theses networks play a pivotal role in enhancing urban efficiency and sustainability. The continuous flow of packets in these networks poses security challenges, from personal data leakage to service unavailability. Anomaly-based methods are commonly used to detect network attacks. In this context, Software-Defined Networking (SDN) emerges as a solution for anomaly detection in network traffic, facilitating comprehensive, real-time analyses and dynamic adaptation to changes. This study aims to present a systematic review of feature selection techniques and evaluate the effectiveness of attribute selection with a multi-class classifier in network anomaly detection. The objective of this paper is to inspire future research and identify trade-offs among these techniques for detecting Denial of Service (DoS) attacks. Based on the findings, the analysis of the entire dataset yields superior results in terms of precision (99%), but using the output of OneR, although resulting in a slight loss of precision compared to the complete dataset, presents the highest precision among other studied techniques, indicating a trade-off between precision and processing time efficiency.
更多查看译文
AI 理解论文
溯源树
样例
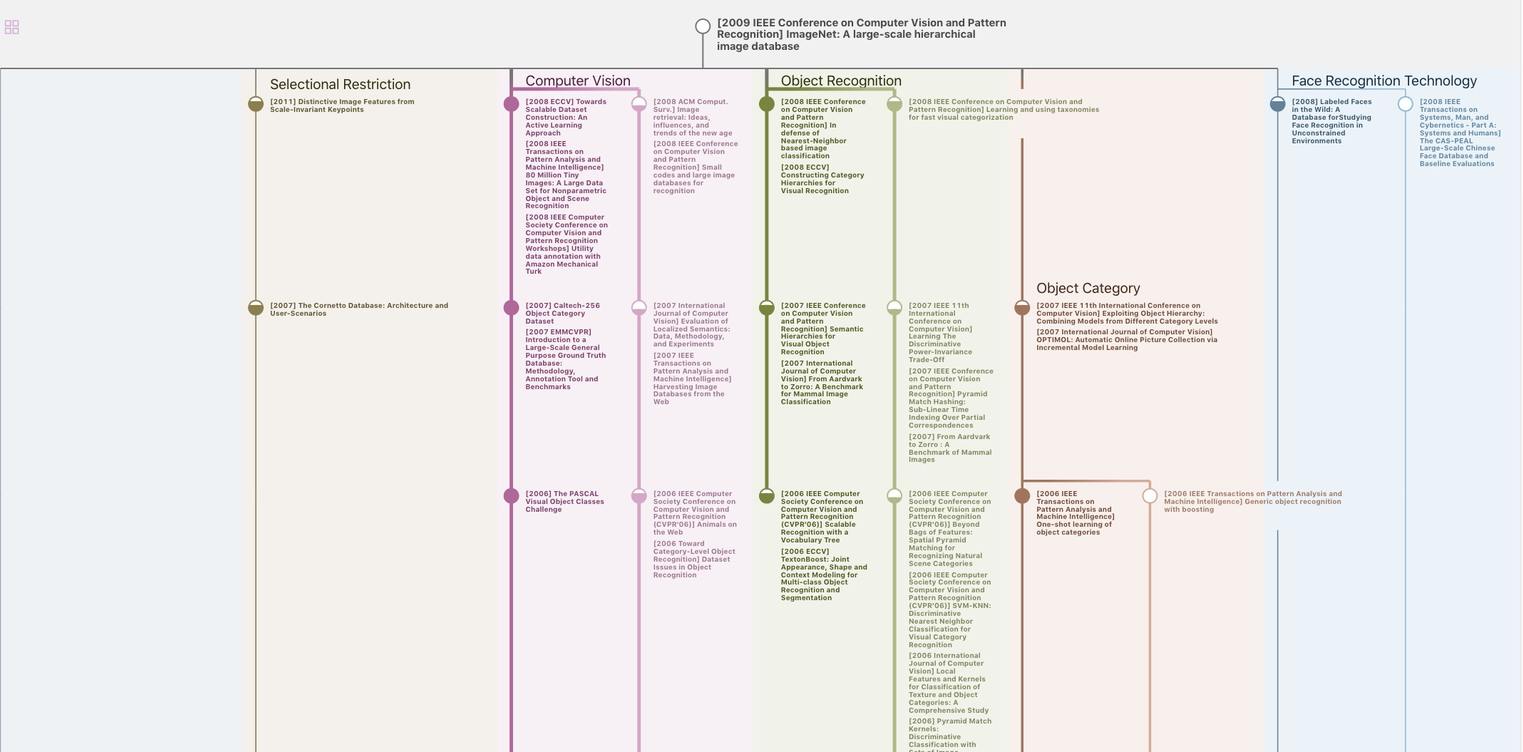
生成溯源树,研究论文发展脉络
Chat Paper
正在生成论文摘要