A novel approach for demand estimation under a flexible mixed logit model
Knowledge-Based Systems(2024)
摘要
This study explores customer heterogeneity and proposes a flexible mixed logit (FML) model without prior knowledge. We investigate a demand estimation problem under the FML model to estimate the choice probabilities of customers and identify the best-fitting mixing distribution for these probabilities. To address this problem, we introduce the loss-likelihood estimation (LLE) method to convert the estimation problem into a constrained convex problem and then design the Frank-Wolfe based (FWB) algorithm to solve the constrained convex problem and prevent model misspecification. This two-stage approach is referred to as the LLE-FWB approach. To validate this approach, we conduct a convergence analysis to calculate its convergence rate and perform numerical experiments to examine its effectiveness and robustness. The theoretical results show that the convergence rate of the LLE-FWB approach is O(1/k), and the simulation results show that this approach performs better, particularly for large data volumes and assortment sizes. Besides, we apply this approach to real-world data to support the implementation of personalized marketing strategies. The results show that the average expected revenue obtained in real-world instances is 17.42% higher than the actual revenue.
更多查看译文
关键词
Demand estimation,Customer heterogeneity,Flexible mixed logit model,Frank-Wolfe algorithm
AI 理解论文
溯源树
样例
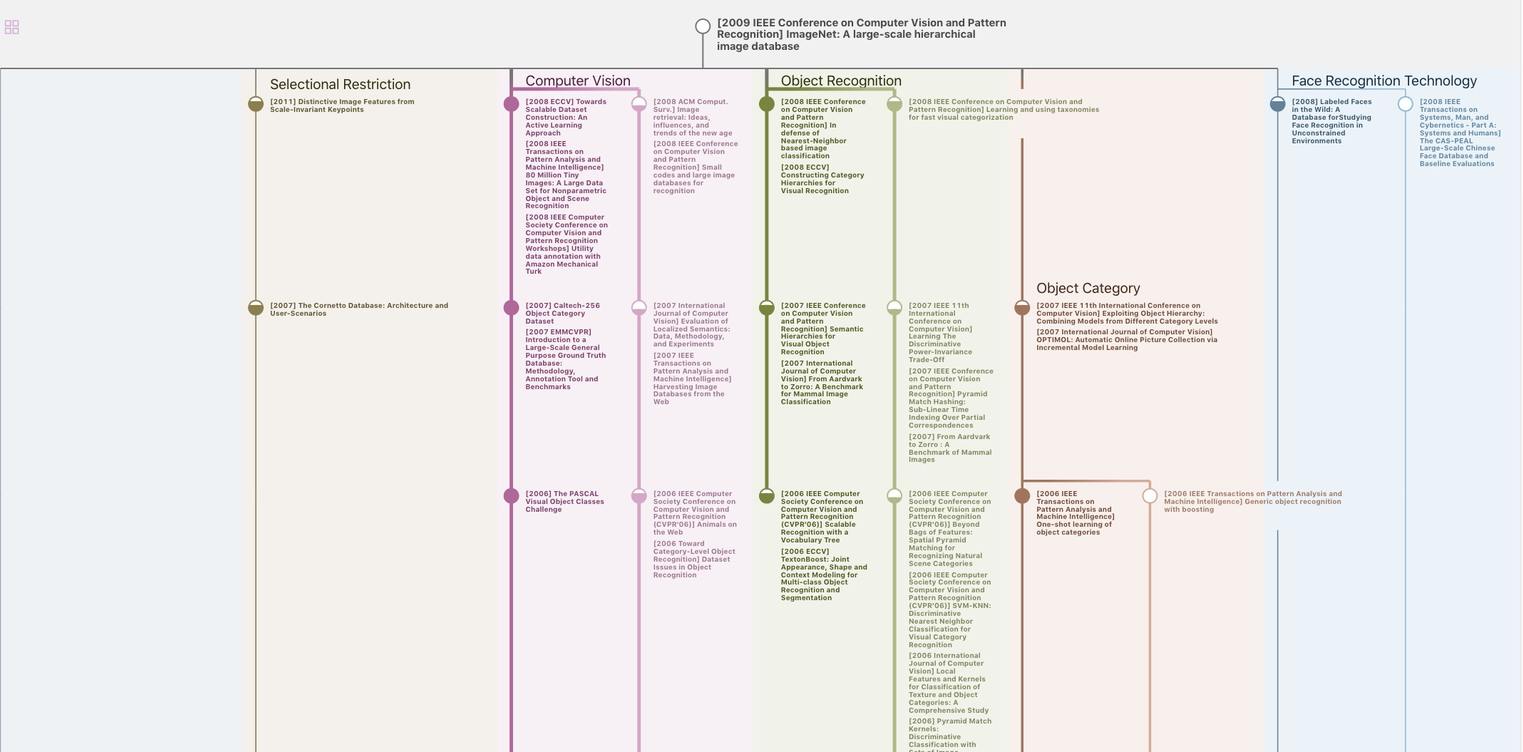
生成溯源树,研究论文发展脉络
Chat Paper
正在生成论文摘要