Baselining the Buzz Trastuzumab-HER2 Affinity, and Beyond
crossref(2024)
摘要
There is currently considerable interest in the field of de novo antibody design, and deep learning techniques are now regularly applied to optimise antibody properties such as binding affinity. However, robust baselines within this field have not kept up with recent developments.
In this study, we generate a dataset of over 524,000 Trastuzumab variants and use this to show that standard computational methods such as BLOSUM, AbLang, ESM, and Protein-MPNN can be used to design diverse antibody libraries from just a single starting sequence. These novel libraries are predicted to be enriched in binding variants and experimental validation of 700 of these designs is ongoing. We also demonstrate that, even with only a very small number of experimental data points, simple machine learning classifiers can be trained in seconds to accurately pre-screen future designs. This pre-screening maintains library diversity and saves experimental time and money.
### Competing Interest Statement
V.G. declares advisory board positions in aiNET GmbH, Enpicom B.V, Absci, Omniscope, and Diagonal Therapeutics. V.G. is a consultant for Adaptive Biosystems, Specifica Inc, Roche/Genentech, immunai, LabGenius, and FairJourney Biologics. J.R.J. is employed by GlaxoSmithKline plc. The remaining authors declare no competing interests.
更多查看译文
AI 理解论文
溯源树
样例
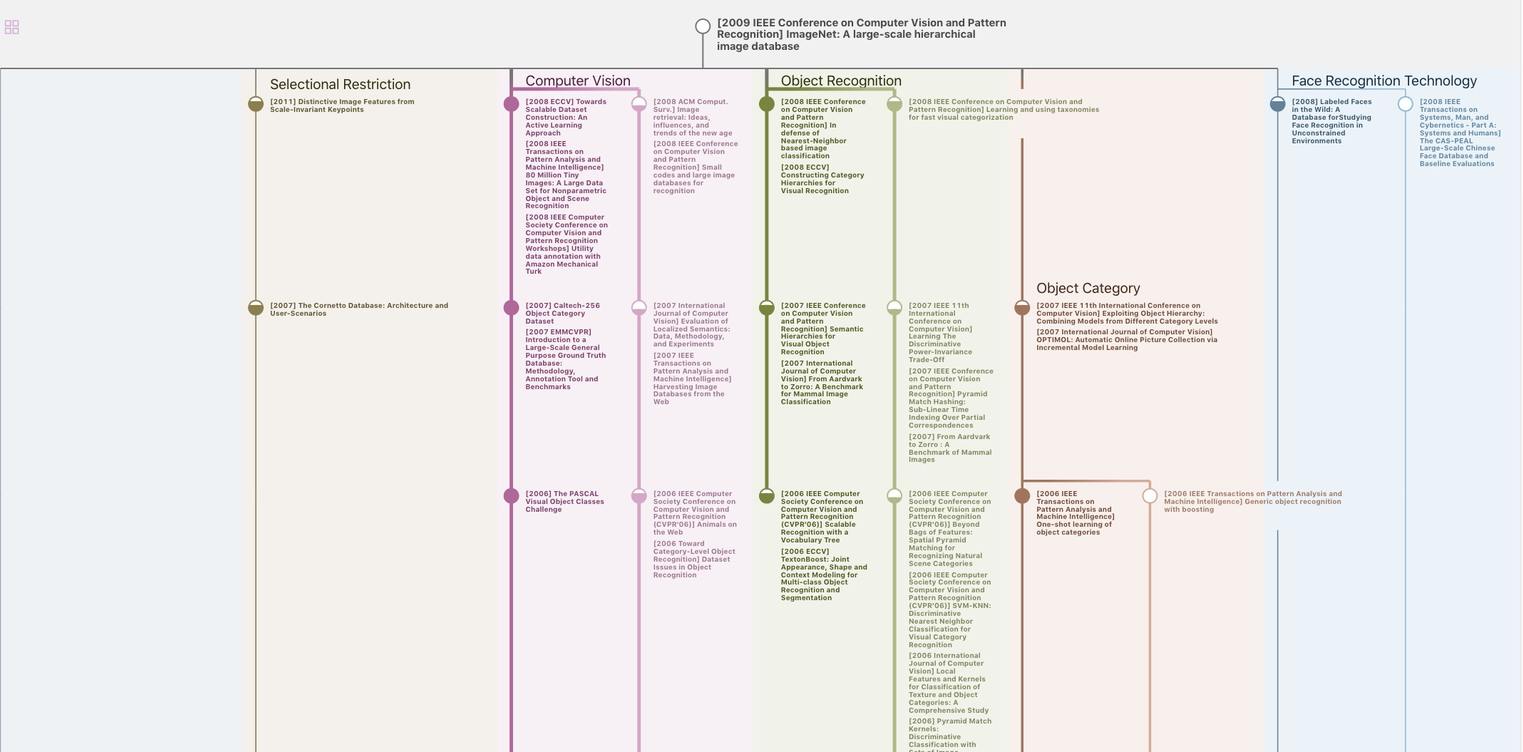
生成溯源树,研究论文发展脉络
Chat Paper
正在生成论文摘要