Elastic Modulus Prediction from Indentation Using Machine Learning: Considering Tip Geometric Imperfection
Metals and Materials International(2024)
摘要
Instrumented indentation technique provides a simple and quick means to investigate mechanical properties such as hardness and elastic modulus near the material surface. However, accurately predicting plastic pileup/sink-in during indentation remains a hurdle in calibrating real contact depth, affecting precise material property evaluation, especially in metallic materials. This study utilizes machine learning on extensive finite element analysis (FEA) data to exclusively predict elastic modulus from indentation curves. Leveraging comprehensive FEA data from sharp and spherical indentations across diverse material properties, our neural network-based models showcase impressive accuracy, achieving approximately 0.65 and 1.72
更多查看译文
关键词
Indentation,Elastic modulus,Neural network,Transfer learning
AI 理解论文
溯源树
样例
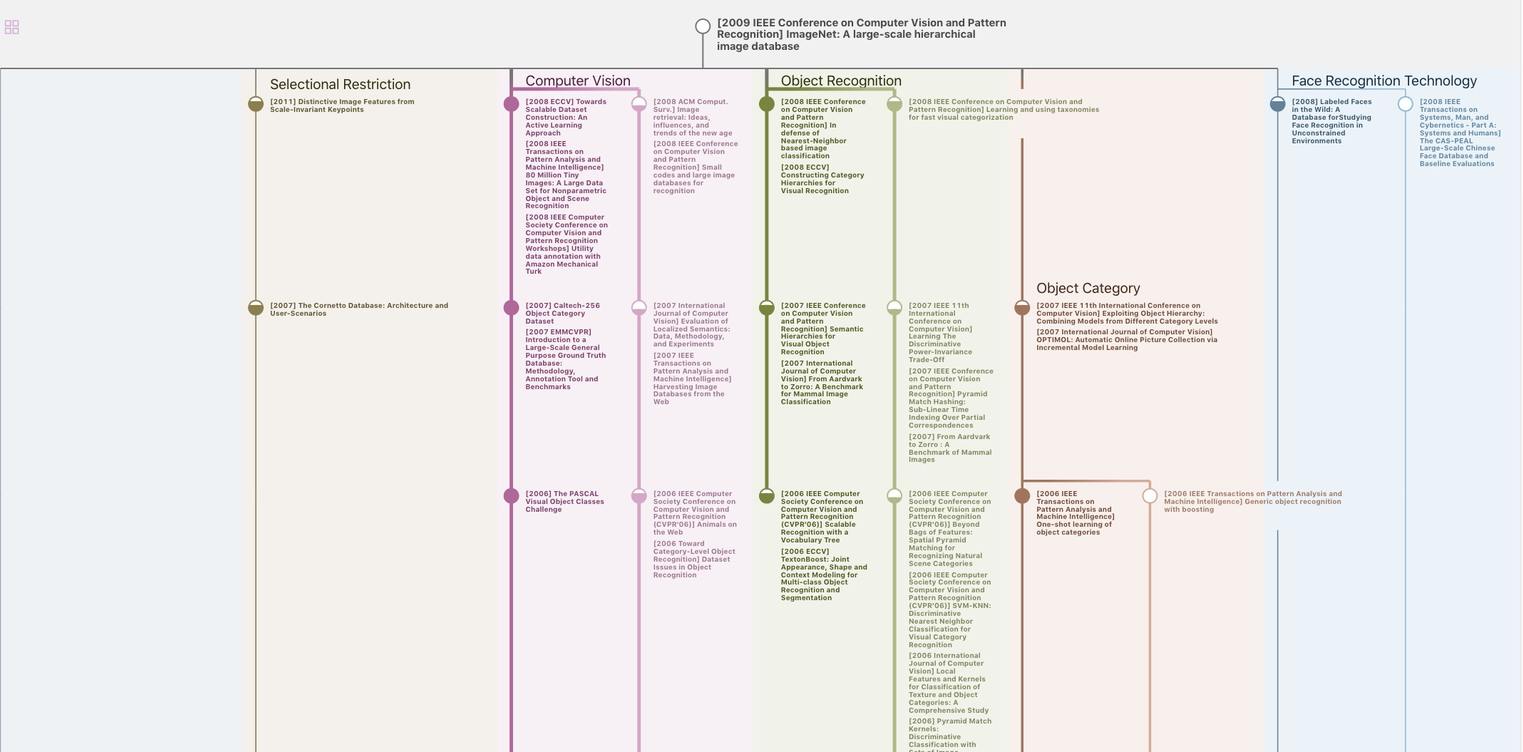
生成溯源树,研究论文发展脉络
Chat Paper
正在生成论文摘要