Triple Generative Self-Supervised Learning Method for Molecular Property Prediction
INTERNATIONAL JOURNAL OF MOLECULAR SCIENCES(2024)
摘要
Molecular property prediction is an important task in drug discovery, and with help of self-supervised learning methods, the performance of molecular property prediction could be improved by utilizing large-scale unlabeled dataset. In this paper, we propose a triple generative self-supervised learning method for molecular property prediction, called TGSS. Three encoders including a bi-directional long short-term memory recurrent neural network (BiLSTM), a Transformer, and a graph attention network (GAT) are used in pre-training the model using molecular sequence and graph structure data to extract molecular features. The variational auto encoder (VAE) is used for reconstructing features from the three models. In the downstream task, in order to balance the information between different molecular features, a feature fusion module is added to assign different weights to each feature. In addition, to improve the interpretability of the model, atomic similarity heat maps were introduced to demonstrate the effectiveness and rationality of molecular feature extraction. We demonstrate the accuracy of the proposed method on chemical and biological benchmark datasets by comparative experiments.
更多查看译文
关键词
generative supervised learning,variational auto-encoders,molecular feature extraction,molecular property prediction,artificial intelligence
AI 理解论文
溯源树
样例
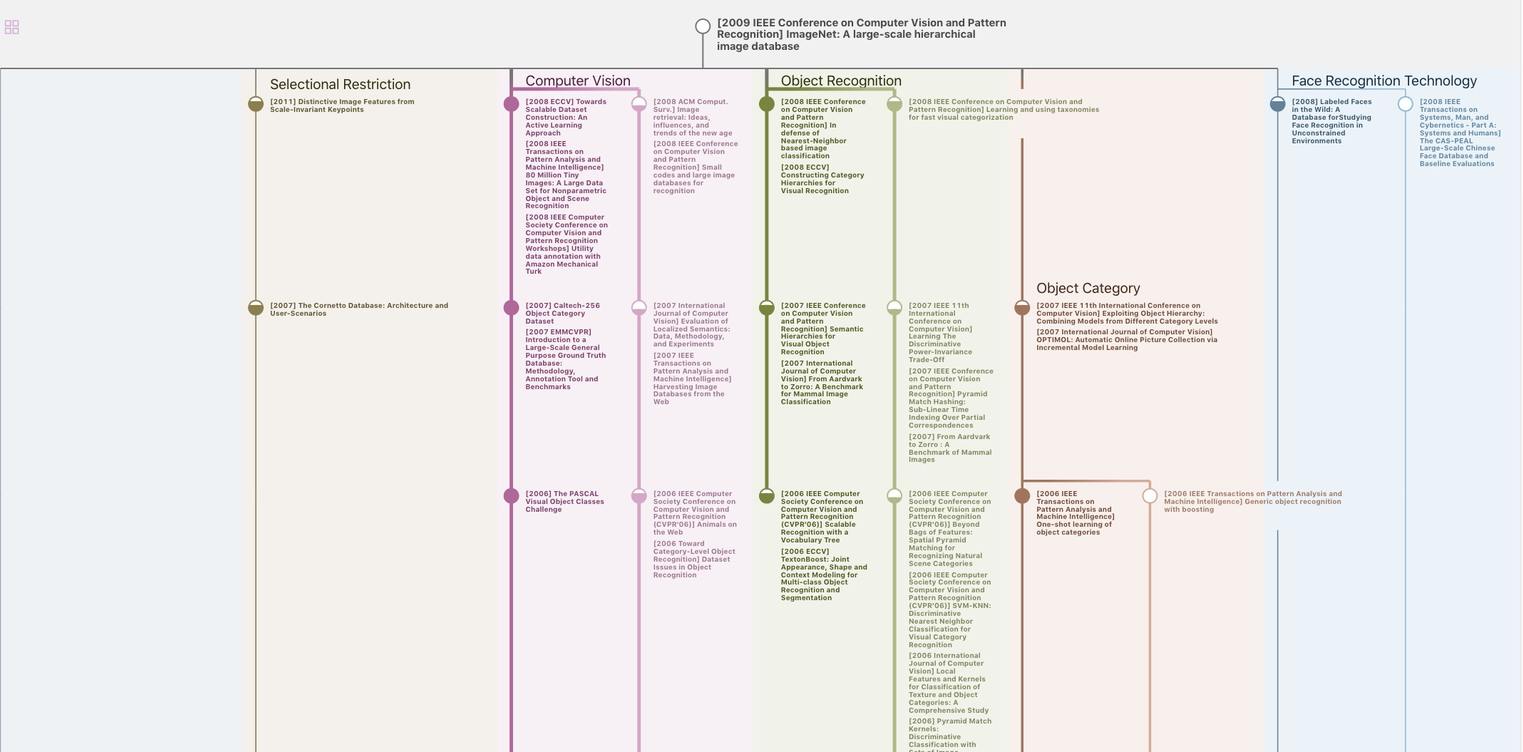
生成溯源树,研究论文发展脉络
Chat Paper
正在生成论文摘要