Online performance and proactive maintenance assessment of data driven prediction models
Journal of Intelligent Manufacturing(2024)
摘要
Many Data-driven decisions in manufacturing need accurate and reliable predictions. Due to high complexity and variability of working conditions, a prediction model may deteriorate over time after deployed. Traditional performance evaluation indexes mainly assess the prediction model from a static perspective, which is difficult to meet the actual needs of model selection and proactive maintenance, resulting in unstable online prediction performance. For regression-based prediction models, this paper designs online prediction performance evaluation indexes (OPPEI) to evaluate the prediction model in terms of its accuracy, degradation speed, and stability. For proactive maintenance, this paper proposes a model maintenance evaluation method based on Principal Component Analysis (PCA). We use PCA to transform various performance indexes and extract the first principal component as a model maintenance evaluation index, which could reduce the over-sensitive or insensitive phenomenon of single indicator. The effectiveness of online prediction performance evaluation indexes and PCA-based proactive maintenance evaluation method are verified by simulation and several real-world load forecasting experiments.
更多查看译文
关键词
Data driven model selection,Predictive model deterioration,Online predictive performance,Proactive maintenance evaluation,Machine learning
AI 理解论文
溯源树
样例
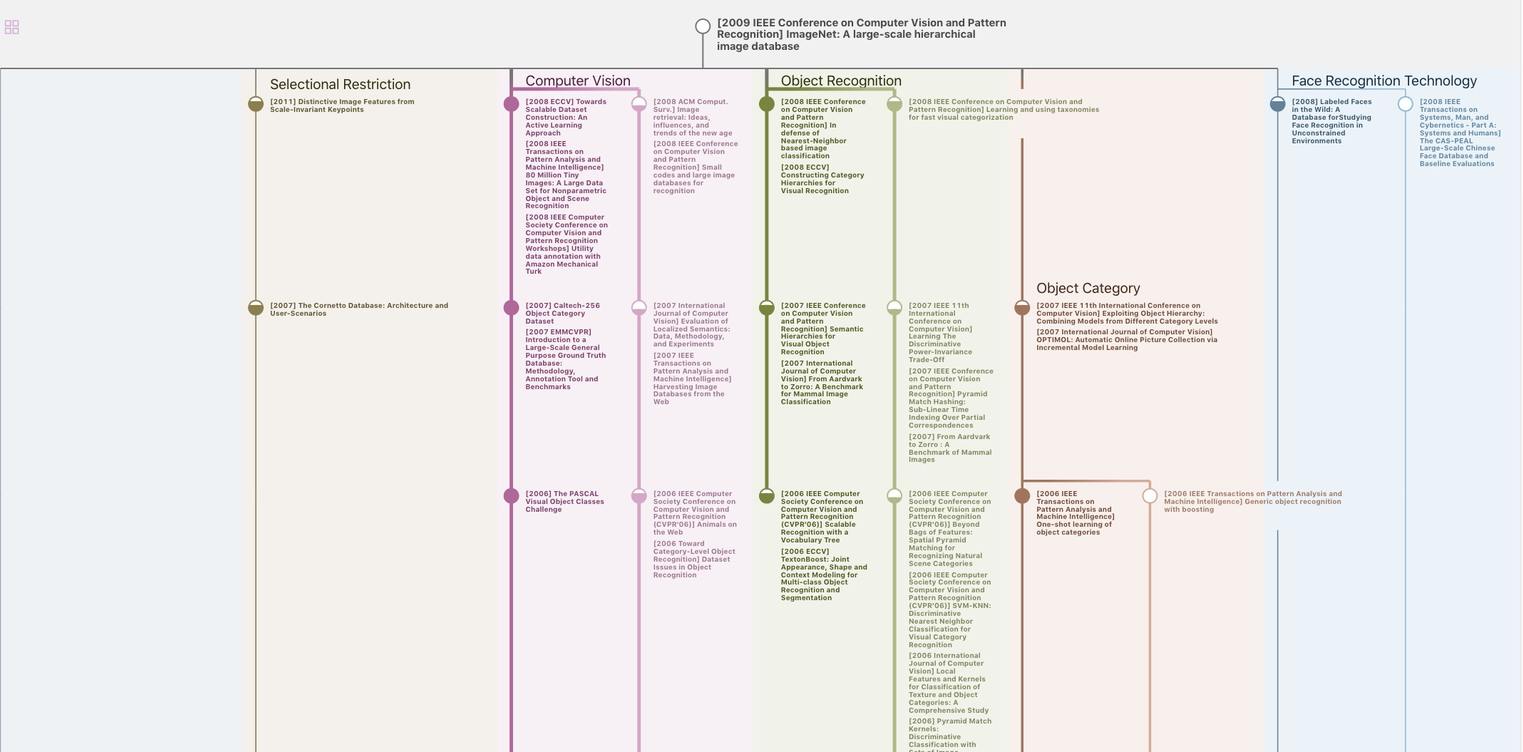
生成溯源树,研究论文发展脉络
Chat Paper
正在生成论文摘要