Deep Convolutional Neural Network for Dedicated Regions-of-Interest Based Multi-Parameter Quantitative Ultrashort Echo Time (UTE) Magnetic Resonance Imaging of the Knee Joint
Journal of Imaging Informatics in Medicine(2024)
摘要
We proposed an end-to-end deep learning convolutional neural network (DCNN) for region-of-interest based multi-parameter quantification (RMQ-Net) to accelerate quantitative ultrashort echo time (UTE) MRI of the knee joint with automatic multi-tissue segmentation and relaxometry mapping. The study involved UTE-based T1 (UTE-T1) and Adiabatic T1ρ (UTE-AdiabT1ρ) mapping of the knee joint of 65 human subjects, including 20 normal controls, 29 with doubtful-minimal osteoarthritis (OA), and 16 with moderate-severe OA. Comparison studies were performed on UTE-T1 and UTE-AdiabT1ρ measurements using 100
更多查看译文
关键词
Quantitative MRI,Automated segmentation,DCNN,RMQ-Net,UTE,Knee joint,OA
AI 理解论文
溯源树
样例
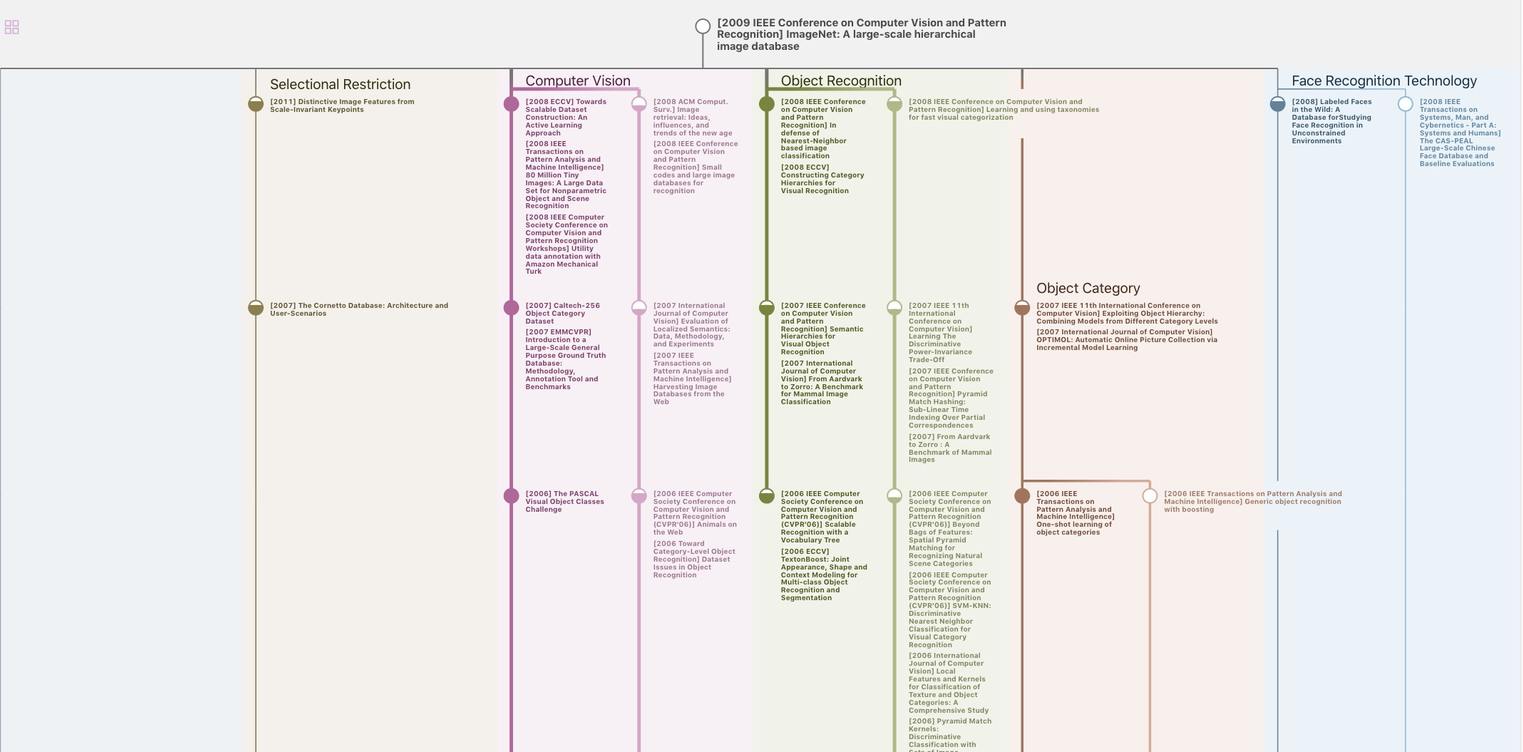
生成溯源树,研究论文发展脉络
Chat Paper
正在生成论文摘要