Msbr-Gnet:A High-Resolution Imagery Generative Optimization Model For Building Rooftop Boundary Guided By Interpretable Statistical Model In Spatial And Spectral Domain
IEEE Journal of Selected Topics in Applied Earth Observations and Remote Sensing(2024)
摘要
Automated extraction of building rooftop information is of great significance in remote sensing of land resources and other related applications. In this paper, a building roof boundary generating optimization model called multi-scale boundary regulation generative net (MSBR-GNet), guided by interpretability statistical model in the spatial and spectral domains, is proposed to solve the problem of inaccurate boundary segmentation caused by mixed pixel transition region of remote sensing images. Incorporate the boundary loss function guided by statistical models in the spatial and spectral domains into the generator loss calculation of MSBR-GNet, precisely constrain the regularized generation of building rooftop contours by interpretable mechanism. The experiments show that MSBR-GNet can extract more regular building rooftop contours, and the precision values in the INRIA, WHU and Massachusetts public datasets reached 0.9275, 0.9228 and 0.8779, respectively, which can ensure the accuracy of building extraction while achieving optimal results in the boundary morphology evaluation index. The code of this paper is open source at
https://github.com/GHLJH/MSBR-GNet
.
更多查看译文
关键词
Building Rooftops,Instance Segmentation,Edge Transition Zones,Contour Optimization,Generative Networks,Tupu Theory,Interpretability
AI 理解论文
溯源树
样例
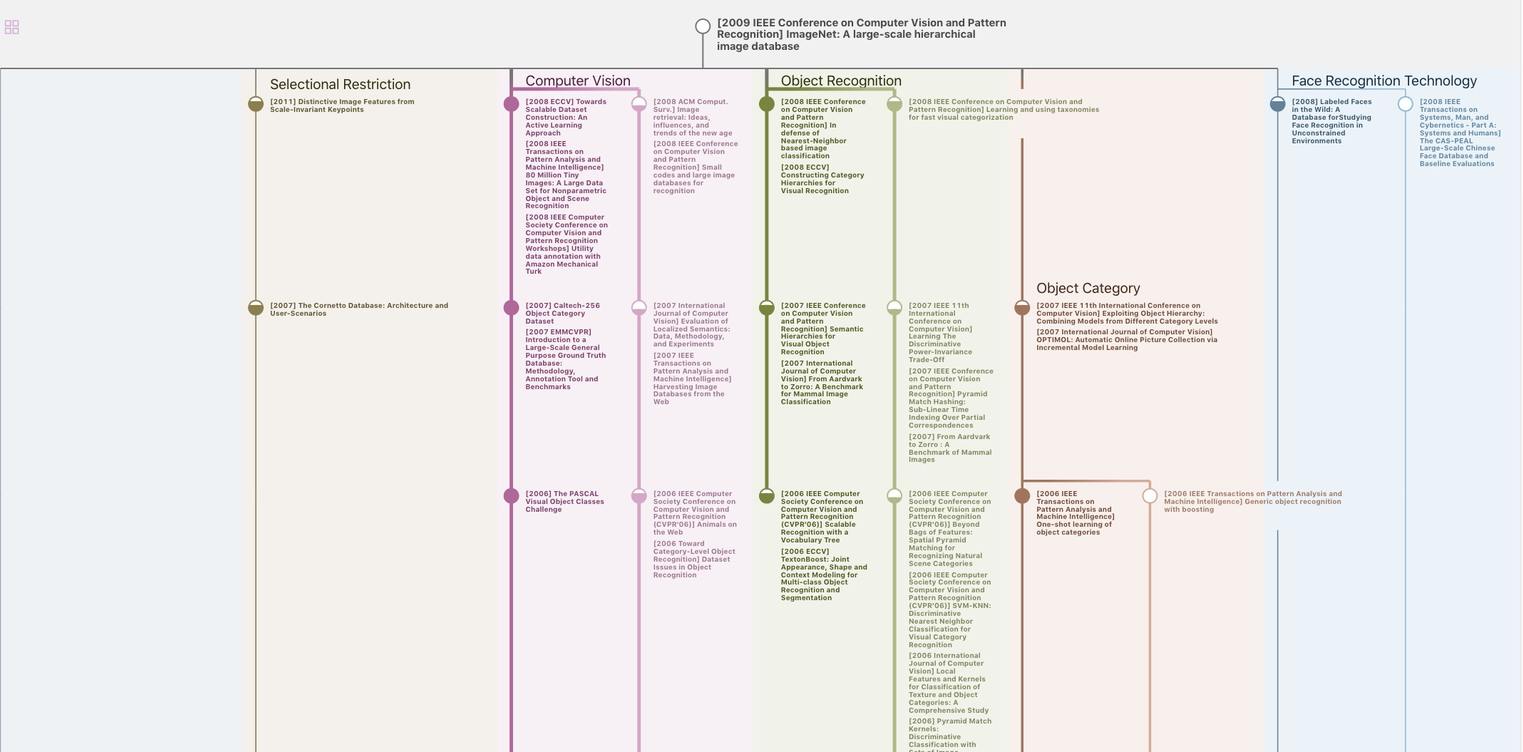
生成溯源树,研究论文发展脉络
Chat Paper
正在生成论文摘要