Adaptive Bitrate Algorithms via Deep Reinforcement Learning With Digital Twins Assisted Trajectory
IEEE Transactions on Network Science and Engineering(2024)
摘要
Adaptive bitrate (ABR) algorithms based on deep reinforcement learning (RL) can continuously improve their adaptability to network conditions. Existing methods usually adopt a reward function to train the ABR policies. However, in some network conditions, the quality of experience (QoE) performances obtained from the deterministic reward functions are not consistent with the users' perception. In this paper, we propose a novel learning-based deep reward model from video trajectory preference data to obtain a dynamic reward function that better reflects users' preference of the video stream. To augment the training data with more precise aggregation in a quick way, we leverage the digital twin (DT) technology to map the users' trajectory into virtual space. The digital twin can mimic users' video trajectory preferences which is used to train a reward model that can precisely predict QoE. Integrated with this reward model, the RL algorithm can generate an ABR policy that optimizes the user-perceived QoE. Experiment results show that the accuracy of the proposed reward model outperforms state-of-the-art reward functions by 13.6% in preference prediction. Compared with other RL-based ABR policies, our RL-based trajectory-preferred ABR algorithm can increase the average user QoE by 16.4%.
更多查看译文
关键词
Adaptive bitrate,reinforcement learning,digital twin,quality of experience,preference,video trajectory
AI 理解论文
溯源树
样例
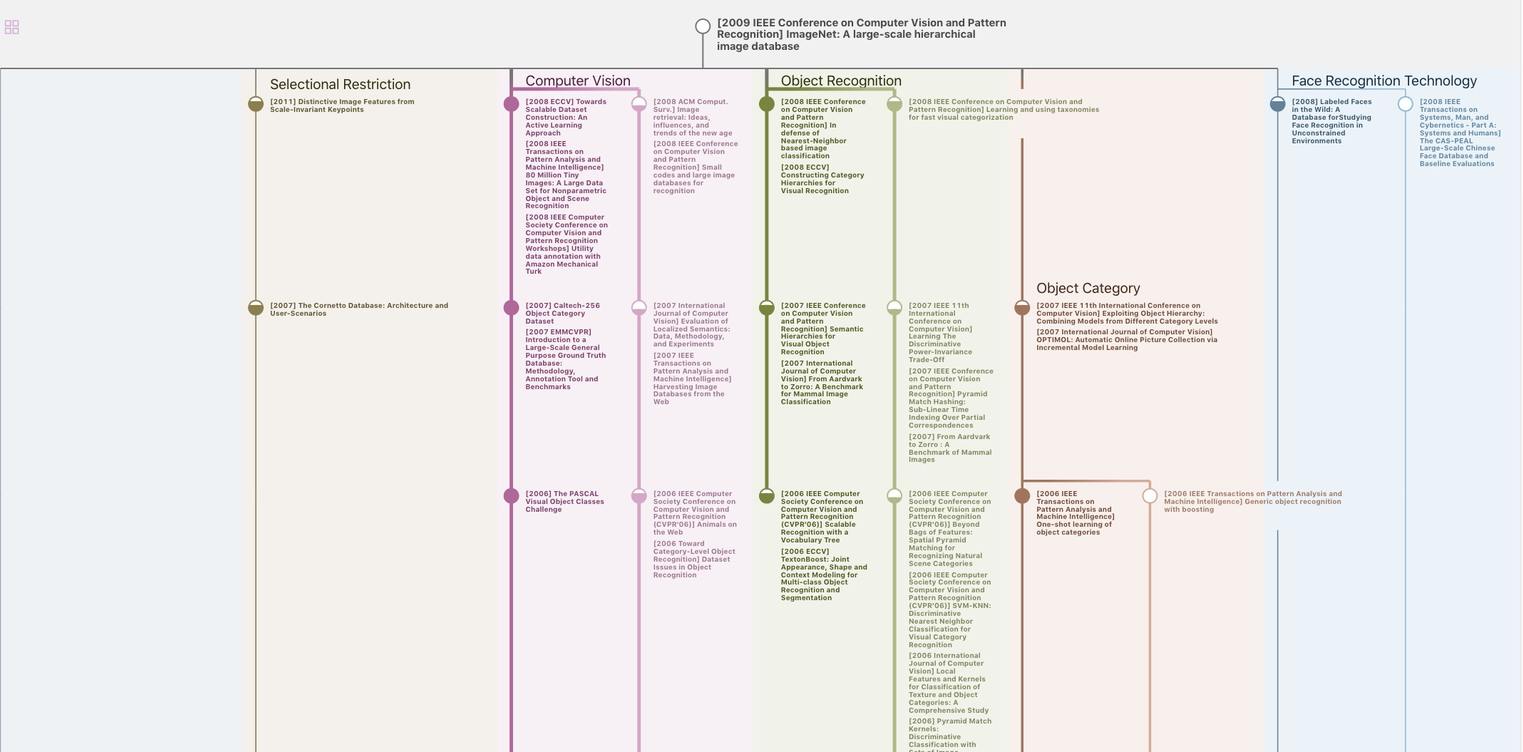
生成溯源树,研究论文发展脉络
Chat Paper
正在生成论文摘要