Accelerating discoveries in medicine using distributed vector representations of words
Expert Systems with Applications(2024)
摘要
Over the years, several neural network architectures have been proposed to process and represent texts using dense vectors (known as word embeddings): mathematical representations that encode the meaning of words or phrases. Word embeddings can be computed by many different algorithms, usually trained on large amounts of textual data aiming to capture semantic relationships between words. These embeddings revolutionized many Natural Language Processing applications, enabling more accurate and nuanced language understanding. Recently, it was demonstrated that it is possible to employ word embeddings to uncover latent knowledge, i.e., information that may be implicit in a set of texts and that would hardly be perceptible to humans. In this context, this study extends such a strategy by combining different unsupervised models to accelerate discoveries in medicine. Our word embeddings were trained on a large corpus of medical papers related to Acute Myeloid Leukemia, a highly malignant form of cancer. Our study shows that established therapies could have been developed before their first proposal due to treatment testing notifications issued by our system up to 11 years in advance. The results show the potential of uncovering latent knowledge from the biomedical field to empower faster and more efficient drug testing for medical discoveries.
更多查看译文
关键词
Distributed vector representations,Word embeddings,Knowledge discovery in databases,Natural language processing,AI in medicine
AI 理解论文
溯源树
样例
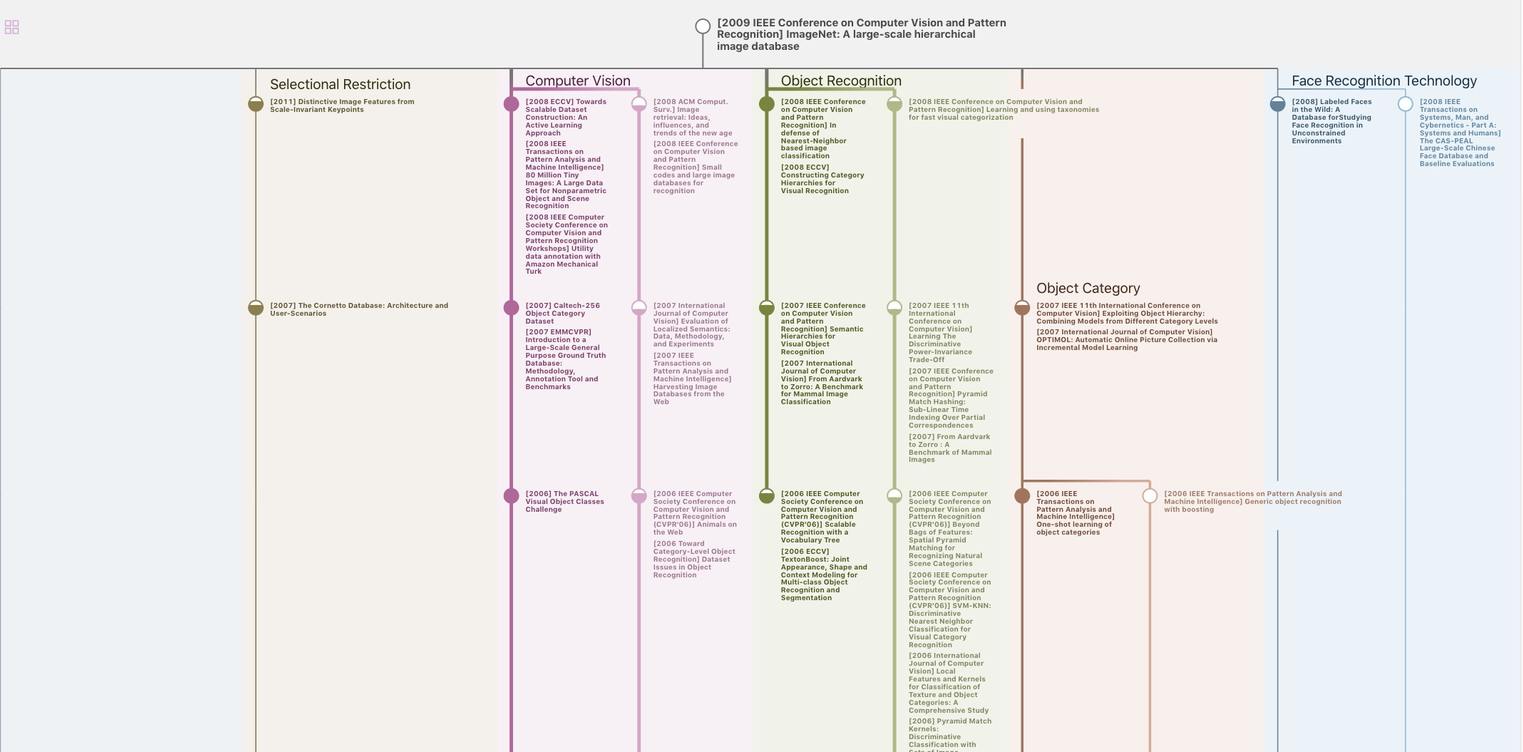
生成溯源树,研究论文发展脉络
Chat Paper
正在生成论文摘要