A Hyperspectral Deep Learning Model for Predicting Anthocyanin Content in Purple Leaf Lettuce
SPECTROSCOPY AND SPECTRAL ANALYSIS(2024)
摘要
The leaves of purple leaf lettuce are rich in anthocyanins, carotene, vitamins, minerals and other elements. Among them, anthocyanin, as the main pigment in the leaf tissue, provides a variety of repair and protection functions for the plants, and its content can reflect the physiological state of purple leaf lettuce, so that the high-accuracy prediction of anthocyanin content has practical significance. In order to efficiently and accurately estimate the anthocyanin content of purple-leaf lettuce, this paper collected hyperspectral data from purple-leaf lettuce and carried out high-precision modeling research. Five preprocessing operations, first derivative (FD), second derivative (SD), standard normal variate transformation (SNV), S-G filter and multiple scattering correction (MSC), were performed on the original average reflectance spectral data. Based on different pretreatment spectra, the partial least squares regression (PLSR) model of anthocyanin content in purple leaf lettuce was established, five preprocessing performances were compared, and MSC was illustrated as the ideal spectral pretreatment method. The competitive Adaptive Reweighted Sampling (CARS) algorithm was used to select characteristic wavebands for the original spectra and the spectra preprocessed by MSC. Based on the full band (original spectra, MSC preprocessed spectra) and characteristic wavebands based on the original spectraand the MSC preprocessed spectra separately), the PLSR model was constructed respectively, the coefficient of determination (R-2) and root mean square error (RMSE) of the best-performing MSC-CARS-PLSR on the validation set were 0.872 and 0.070 mg.L-1, respectively, and the residual prediction deviation (RPD) was 2.862. In order to improve the prediction accuracy further, this paper proposes a regression analysis framework marked as Ensemble that integrates deep convolutional features and extreme learning machines (ELM). Based on the improved Inception module, a one-dimensional convolutional neural network (1DCNN) matching the input spectral signal is designed as a feature extractor. ELM is used as an advanced regressor to replace a fully connected layer to calculate the extracted features. Through comparative analysis, the performance of Ensemble is better than that of a single 1DCNN model, ELM model and the best PLSR model based on preprocessed spectra, and its R-2 and RMSE on the validation set were 0.905 and 0.060 mg.L-1, respectively, and the RPD was 3.319, showing high prediction accuracy and excellent stability. The impact of preprocessing operations on the prediction accuracy of Ensemble is further analyzed. The experimental results show that Ensemble is much less dependent on preprocessing operations than PLSR, indicating that the model inherits the deep feature representation of 1DCNN and the high generalization of ELM at the same time, and can realize end-to-end high-precision prediction of anthocyanin content based on the original spectrum, which provides theoretical support for timely and accurate grasp of the growth situation of purple leaf lettuce.The leaves of purple leaf lettuce are rich in anthocyanins, carotene, vitamins, minerals and other elements. Among them, anthocyanin, as the main pigment in the leaf tissue, provides a variety of repair and protection functions for the plants, and its content can reflect the physiological state of purple leaf lettuce, so that the high-accuracy prediction of anthocyanin content has practical significance. In order to efficiently and accurately estimate the anthocyanin content of purple-leaf lettuce, this paper collected hyperspectral data from purple-leaf lettuce and carried out high-precision modeling research. Five preprocessing operations, first derivative (FD), second derivative (SD), standard normal variate transformation (SNV), S-G filter and multiple scattering correction (MSC), were performed on the original average reflectance spectral data. Based on different pretreatment spectra, the partial least squares regression (PLSR) model of anthocyanin content in purple leaf lettuce was established, five preprocessing performances were compared, and MSC was illustrated as the ideal spectral pretreatment method. The competitive Adaptive Reweighted Sampling (CARS) algorithm was used to select characteristic wavebands for the original spectra and the spectra preprocessed by MSC. Based on the full band (original spectra, MSC preprocessed spectra) and characteristic wavebands based on the original spectraand the MSC preprocessed spectra separately), the PLSR model was constructed respectively, the coefficient of determination (R-2) and root mean square error (RMSE) of the best-performing MSC-CARS-PLSR on the validation set were 0.872 and 0.070 mg.L-1, respectively, and the residual prediction deviation (RPD) was 2.862. In order to improve the prediction accuracy further, this paper proposes a regression analysis framework marked as Ensemble that integrates deep convolutional features and extreme learning machines (ELM). Based on the improved Inception module, a one-dimensional convolutional neural network (1DCNN) matching the input spectral signal is designed as a feature extractor. ELM is used as an advanced regressor to replace a fully connected layer to calculate the extracted features. Through comparative analysis, the performance of Ensemble is better than that of a single 1DCNN model, ELM model and the best PLSR model based on preprocessed spectra, and its R-2 and RMSE on the validation set were 0.905 and 0.060 mg.L-1, respectively, and the RPD was 3.319, showing high prediction accuracy and excellent stability. The impact of preprocessing operations on the prediction accuracy of Ensemble is further analyzed. The experimental results show that Ensemble is much less dependent on preprocessing operations than PLSR, indicating that the model inherits the deep feature representation of 1DCNN and the high generalization of ELM at the same time, and can realize end-to-end high-precision prediction of anthocyanin content based on the original spectrum, which provides theoretical support for timely and accurate grasp of the growth situation of purple leaf lettuce.
更多查看译文
关键词
Purple leaf lettuce,Anthocyanins,Hyperspectral,Partial least squares regression,Deep learning
AI 理解论文
溯源树
样例
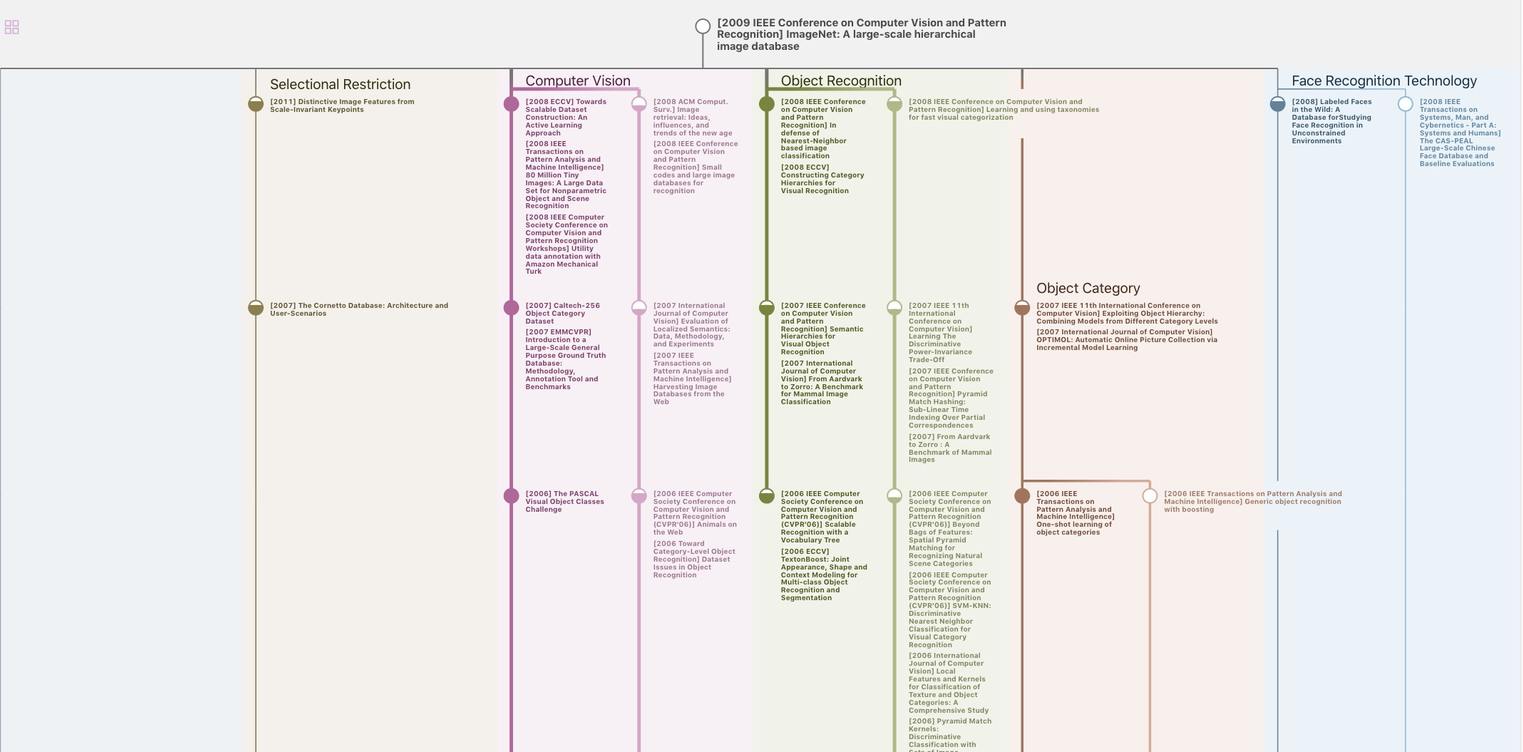
生成溯源树,研究论文发展脉络
Chat Paper
正在生成论文摘要