H-Consistency Guarantees for Regression
arxiv(2024)
摘要
We present a detailed study of H-consistency bounds for regression. We
first present new theorems that generalize the tools previously given to
establish H-consistency bounds. This generalization proves essential for
analyzing H-consistency bounds specific to regression. Next, we prove a
series of novel H-consistency bounds for surrogate loss functions of the
squared loss, under the assumption of a symmetric distribution and a bounded
hypothesis set. This includes positive results for the Huber loss, all ℓ_p
losses, p ≥ 1, the squared ϵ-insensitive loss, as well as a
negative result for the ϵ-insensitive loss used in squared Support
Vector Regression (SVR). We further leverage our analysis of H-consistency
for regression and derive principled surrogate losses for adversarial
regression (Section 5). This readily establishes novel algorithms for
adversarial regression, for which we report favorable experimental results in
Section 6.
更多查看译文
AI 理解论文
溯源树
样例
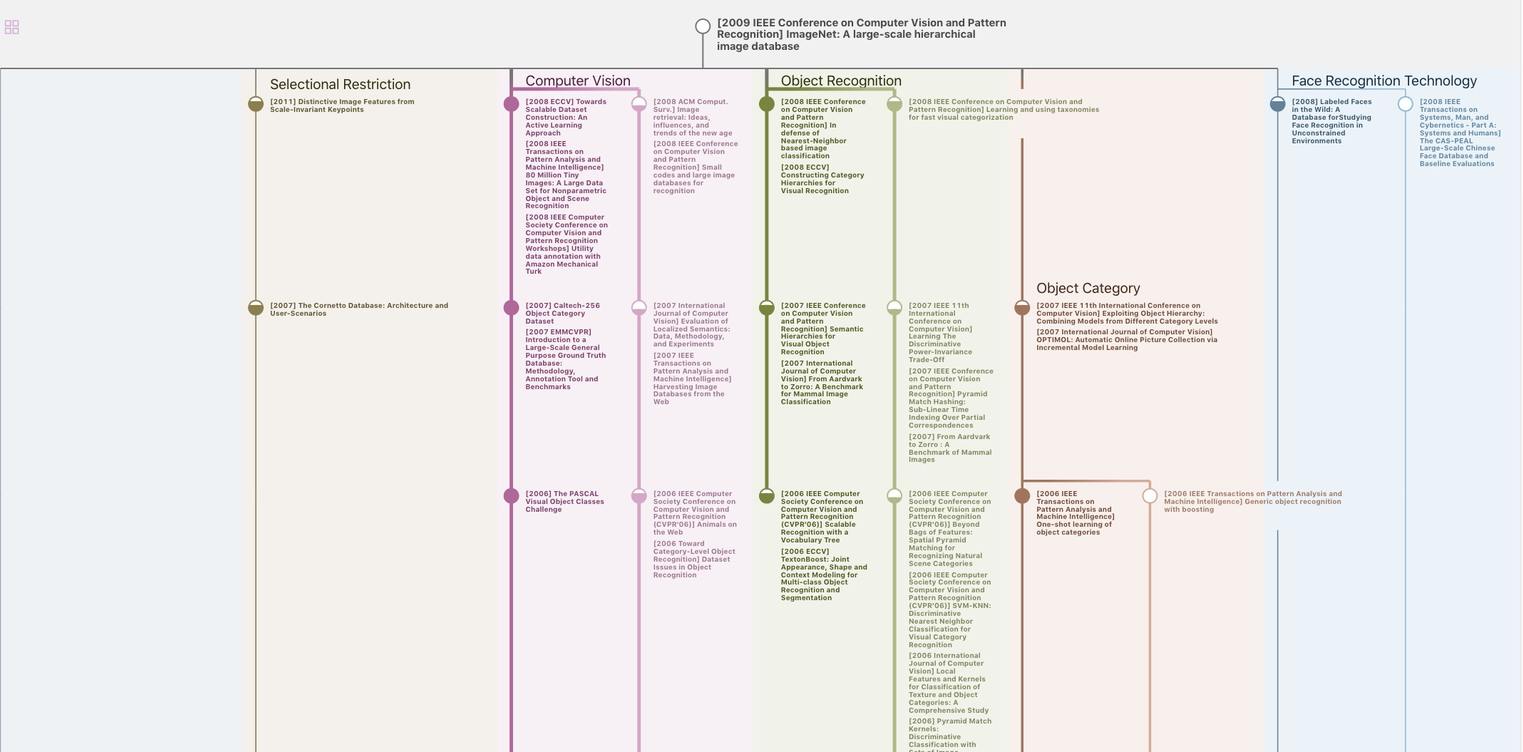
生成溯源树,研究论文发展脉络
Chat Paper
正在生成论文摘要