EDA-Driven Preprocessing for SAT Solving
arxiv(2024)
摘要
Effective formulation of problems into Conjunctive Normal Form (CNF) is
critical in modern Boolean Satisfiability (SAT) solving for optimizing solver
performance. Addressing the limitations of existing methods, our Electronic
Design Automation (EDA)-driven preprocessing framework introduces a novel
methodology for preparing SAT instances, leveraging both circuit and CNF
formats for enhanced flexibility and efficiency. Central to our approach is the
integration of a new logic synthesis technique, guided by a reinforcement
learning agent, and a novel cost-customized LUT mapping strategy, enabling
efficient handling of diverse SAT challenges. By transforming the SAT
competition benchmarks into circuit instances, our framework demonstrates
substantial performance improvements, as evidenced by a 52.42
average compared to solving directly. Moreover, our framework achieves a
remarkable 96.14
checking problems that exhibit inherent circuit structures. These results
highlight the effectiveness and versatility of our approach in handling both
CNF and circuit instances. The code is available at
https://github.com/cure-lab/EDA4SAT.
更多查看译文
AI 理解论文
溯源树
样例
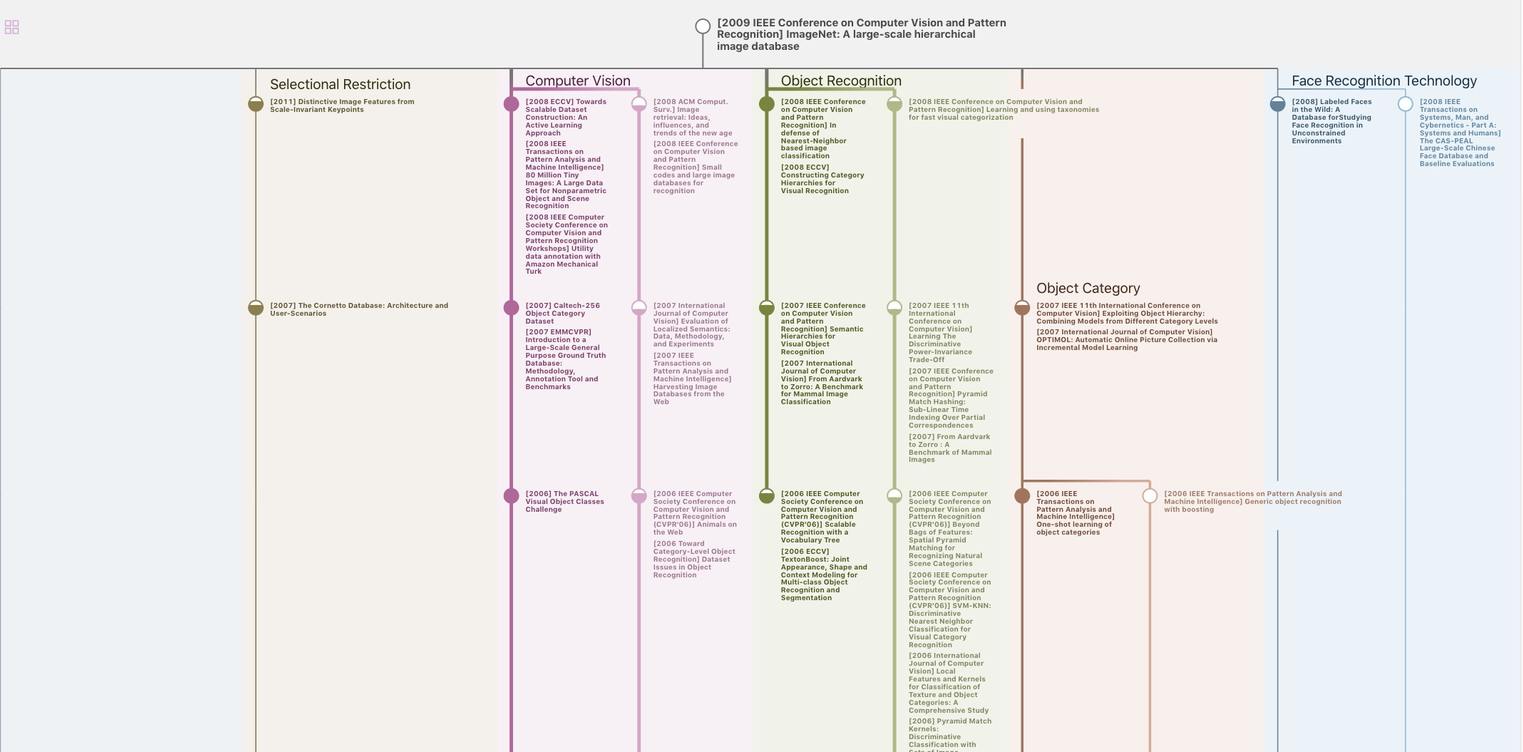
生成溯源树,研究论文发展脉络
Chat Paper
正在生成论文摘要