Safety-Critical Planning and Control for Dynamic Obstacle Avoidance Using Control Barrier Functions
arxiv(2024)
摘要
Dynamic obstacle avoidance is a challenging topic for optimal control and
optimization-based trajectory planning problems, especially when in a tight
environment. Many existing works use control barrier functions (CBFs) to
enforce safety constraints within control systems. Inside these works, CBFs are
usually formulated under model predictive control (MPC) framework to anticipate
future states and make informed decisions, or integrated with path planning
algorithms as a safety enhancement tool. However, these approaches usually
require knowledge of the obstacle boundary equations or have very slow
computational efficiency. In this paper, we propose a novel framework to the
iterative MPC with discrete-time CBFs (DCBFs) to generate a collision-free
trajectory. The DCBFs are obtained from convex polyhedra generated in
sequential grid maps, without the need to know the boundary equations of
obstacles. Additionally, a path planning algorithm is incorporated into this
framework to ensure the global optimality of the generated trajectory. We
demonstrate through numerical examples that our framework enables a unicycle
robot to safely and efficiently navigate through tight and dynamically changing
environments, tackling both convex and nonconvex obstacles with remarkable
computing efficiency and reliability in control and trajectory generation.
更多查看译文
AI 理解论文
溯源树
样例
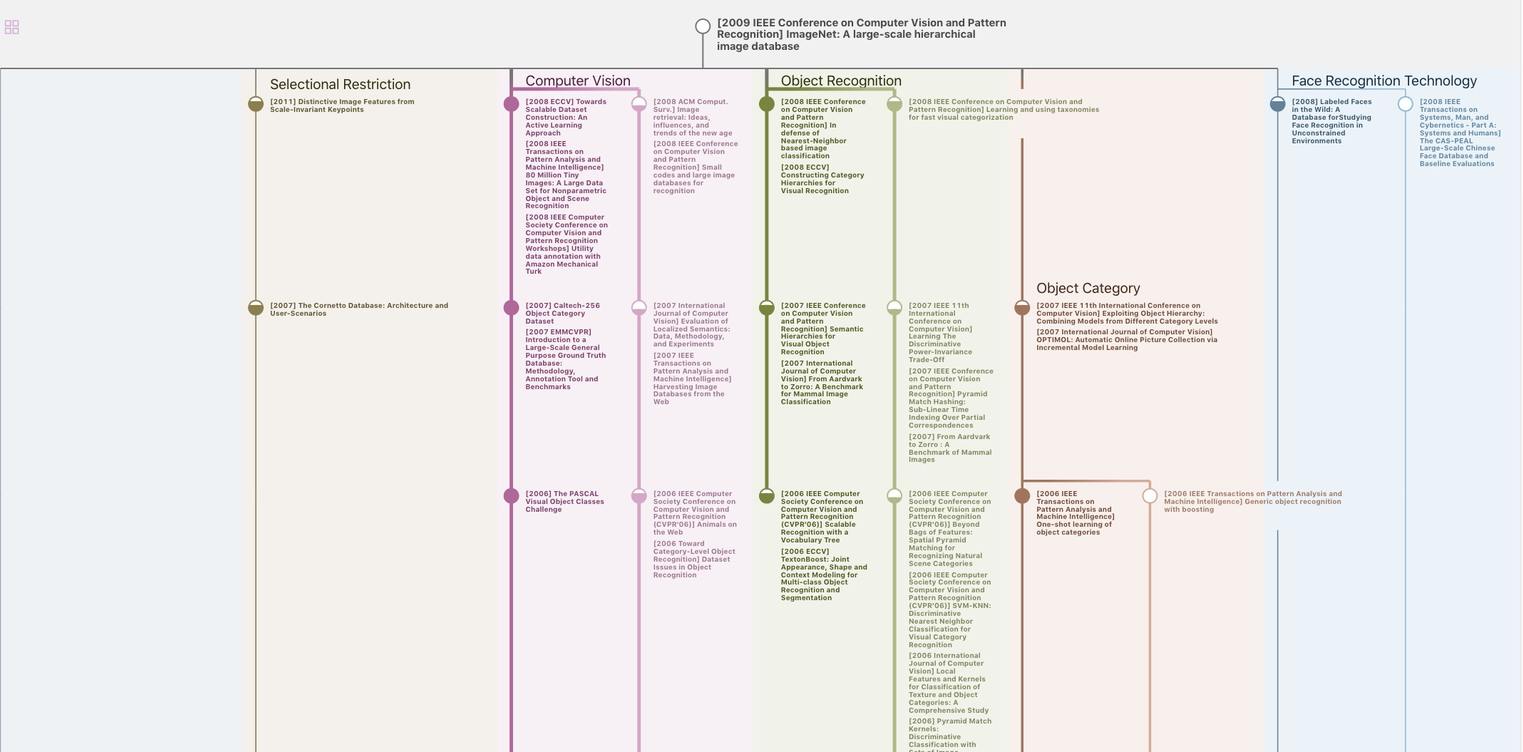
生成溯源树,研究论文发展脉络
Chat Paper
正在生成论文摘要