A recommender network perspective on the informational value of critics and crowds
arxiv(2024)
摘要
How do the ratings of critics and amateurs compare and how should they be
combined? Previous research has produced mixed results about the first
question, while the second remains unanswered. We have created a new, unique
dataset, with wine ratings from critics and amateurs, and simulated a
recommender system using the k-nearest-neighbor algorithm. We then formalized
the advice seeking network spanned by that algorithm and studied people's
relative influence. We find that critics are more consistent than amateurs, and
thus their advice is more predictive than advice from amateurs. Getting advice
from both groups can further boost performance. Our network theoretic approach
allows us to identify influential critics, talented amateurs, and the
information flow between groups. Our results provide evidence about the
informational function of critics, while our framework is broadly applicable
and can be leveraged to devise good decision strategies and more transparent
recommender systems.
更多查看译文
AI 理解论文
溯源树
样例
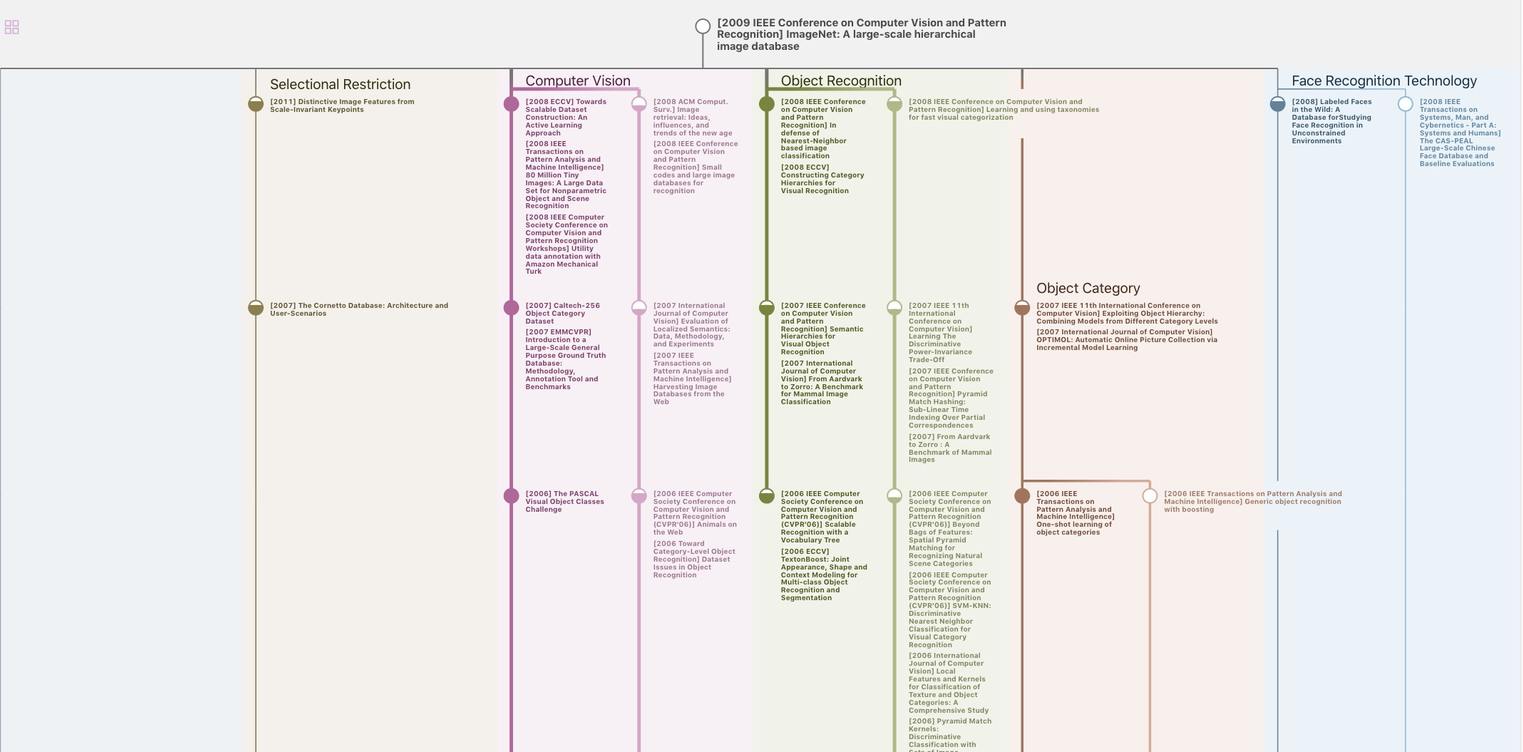
生成溯源树,研究论文发展脉络
Chat Paper
正在生成论文摘要