Highly Accurate Profiling of Exosome Phenotypes Using Super-resolution Tricolor Fluorescence Co-localization
ACS NANO(2024)
摘要
Exosomes contain a wealth of proteomic information, presenting promising biomarkers for the noninvasive early diagnosis of diseases, especially cancer. However, it remains a great challenge to accurately and reliably distinguish exosomes secreted from different types of cell lines. Fluorescence immunoassay is frequently used for exosome detection. Nonspecific adsorption in immunoassays is unavoidable and affects the reliability of assay results. Despite the fact that various methods have been proposed to reduce nonspecific adsorption, a more effective method that can eliminate the influence of nonspecific adsorption is still lacking. Here, we report a more convenient way (named SR-TFC) to remove the artifacts caused by nonspecific adsorption, which combines tricolor fluorescence labeling of target exosomes, tricolor super-resolution imaging, and pixel counting. The pixel counting method (named CFPP) is realized by MATLAB and can eliminate nonspecific binding sites at the single-pixel level, which has never been achieved before and could improve the reliability of detection to the maximum extent. Furthermore, as a proof-of-concept, profiling of exosomal membrane proteins and identification of breast cancer subpopulations are demonstrated. To enable multiplex breast cancer phenotypic analysis, three kinds of specific proteins are labeled to obtain the 3D phenotypic information on various exosomes. Breast cancer subtypes can be accurately identified according to the super-resolution images of some clinically relevant exosomal proteins. Worth mentioning is that, by selecting other biomarkers, classification of other cancers could also be realized using SR-TFC. Hence, the present work holds great potential in clinical cancer diagnosis and precision medicine.
更多查看译文
关键词
exosomes,phenotypes,multicolor fluorescenceco-localization,pixel counting,super-resolutionfluorescence imaging
AI 理解论文
溯源树
样例
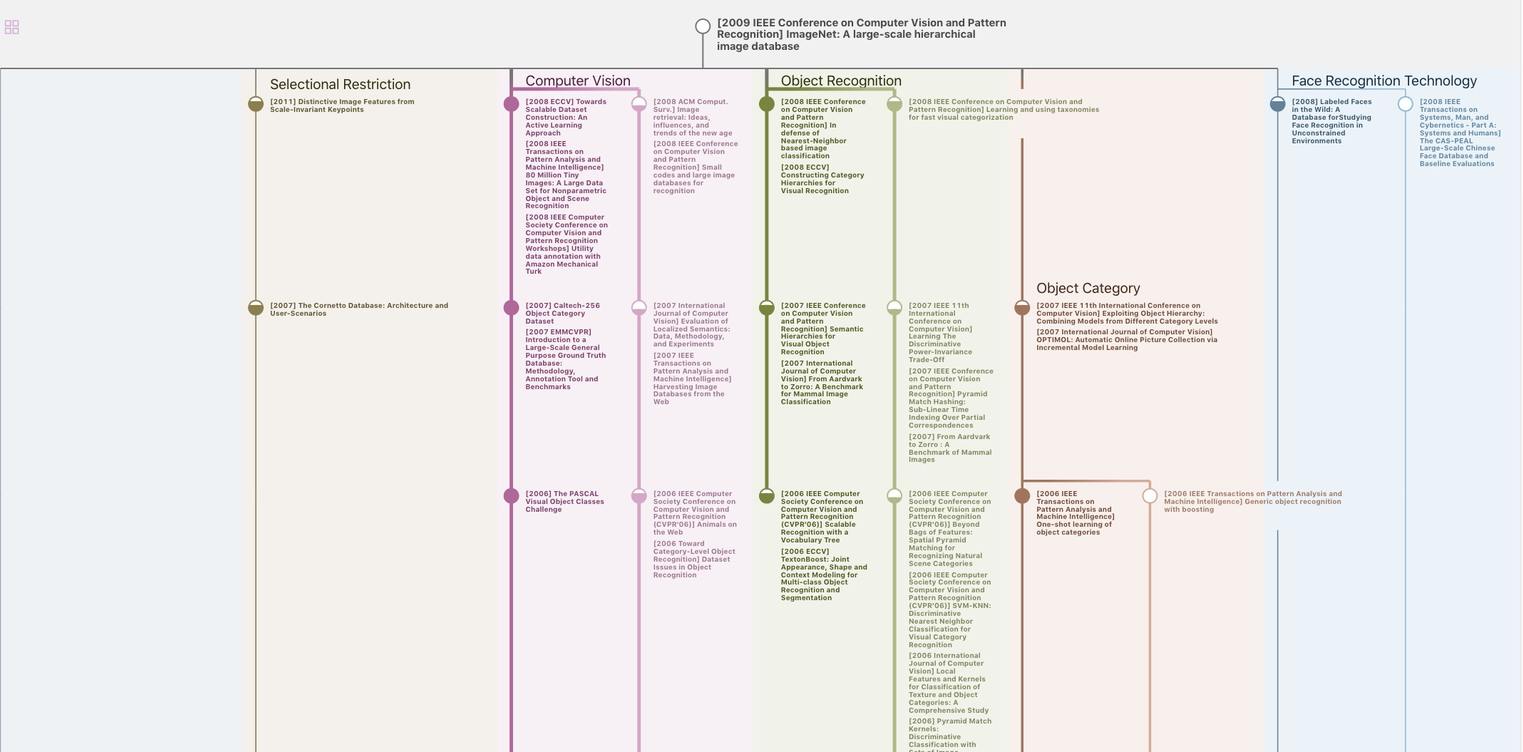
生成溯源树,研究论文发展脉络
Chat Paper
正在生成论文摘要