Context-Awareness Network with Multi-Level Feature Fusion for Building Change Detection
The 6th International Conference on Numerical Modelling in Engineering Advances in Science and Technology(2024)
摘要
Building change detection is critical for urban management. Deep learning methods are more discriminatory and learnable than traditional change detection methods. But in complicated backdrop environments, it is still difficult to precisely pinpoint change zones of interest. Most change detection networks suffer from inaccurate feature characterization during feature extraction and fusion. As a solution to these problems, we propose the use of multilevel feature fusion in conjunction with aware networks to detect building changes. To obtain multi-scale change characteristics, our Context-awareness network employs multi-scale patch embedding. Followed by multi-path Transformers to enhance learning and extract more suitable features. The multi-scale fusion module can ensure semantic consistency of change features, making detected change regions more accurate. Visual comparisons and quantitative evaluations of our method showed that it outperformed seven popular change detection methods on the LEVIR-CD dataset.
更多查看译文
AI 理解论文
溯源树
样例
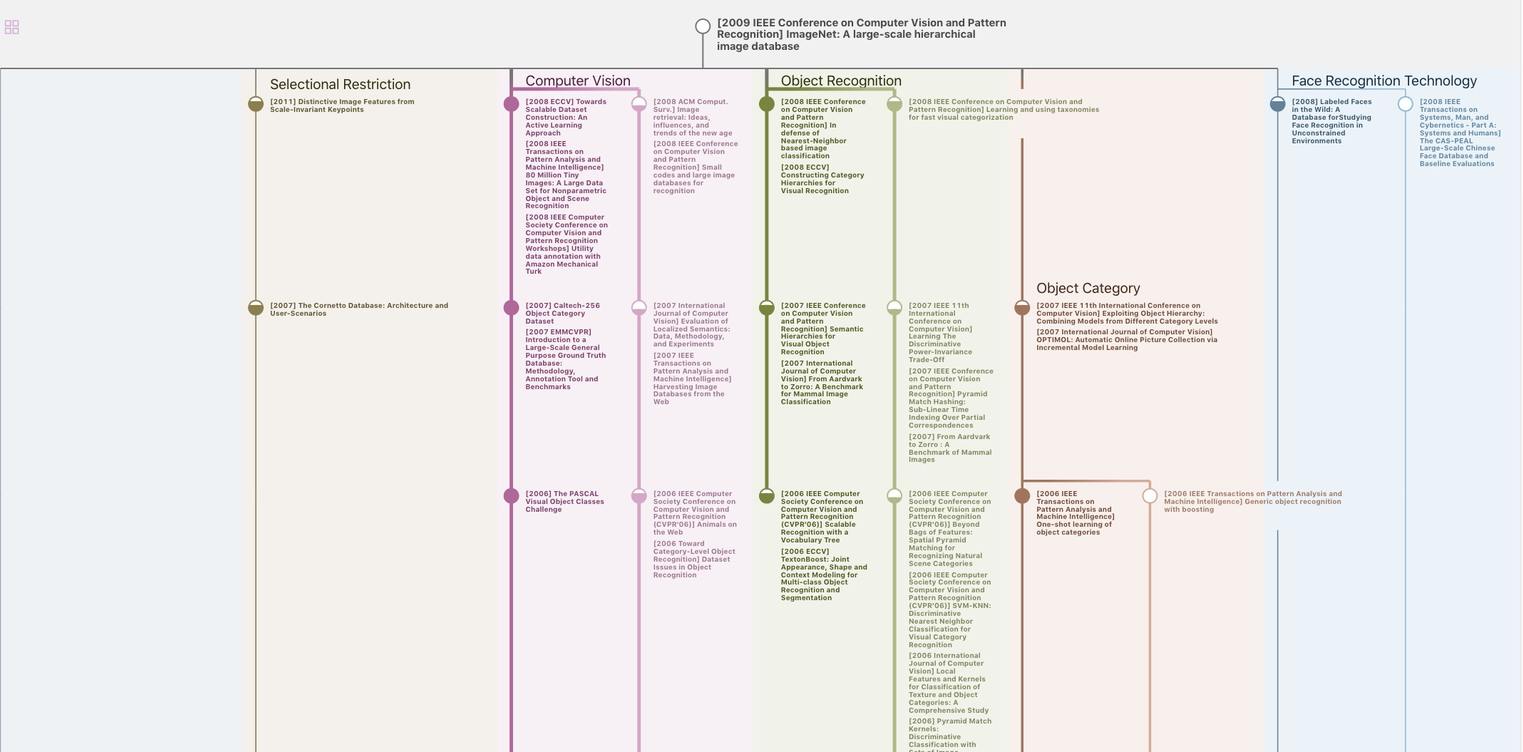
生成溯源树,研究论文发展脉络
Chat Paper
正在生成论文摘要